Learning-based model predictive control for safe trajectory planning and control
Authorea (Authorea)(2023)
摘要
The control performance of model predictive control (MPC) strongly depends on the accuracy of the model description. To better capture and predict the dynamic behaviors of the controlled plant, a non-parametric model which is regressed by the Gaussian Process (GP) is proposed in this paper to evaluate the unknown deviation between the nominal model and the physical system. Firstly, an efficient MPC formulation that integrates a nominal model with GP model which evaluates the unmodeled dynamics is designed for safe and robust maneuver planning. Secondly, the geometric hard constraints for collision avoidance between ego cars and obstacles are softened by using a relaxed barrier function for optimization efficiency. A configuration space convexification algorithm is designed for convexifying the corridor constraints in path pre-selection and re-planned for obstacle avoidance. The control performance of the learning-based MPC is demonstrated and compared with the standard MPC strategy under two typical scenarios. Numerical simulation as well as experiment results indicate that the proposed method could keep the safety, stability, and maneuverability of the ego vehicle during obstacle avoidance.
更多查看译文
关键词
safe trajectory planning,predictive control,learning-based
AI 理解论文
溯源树
样例
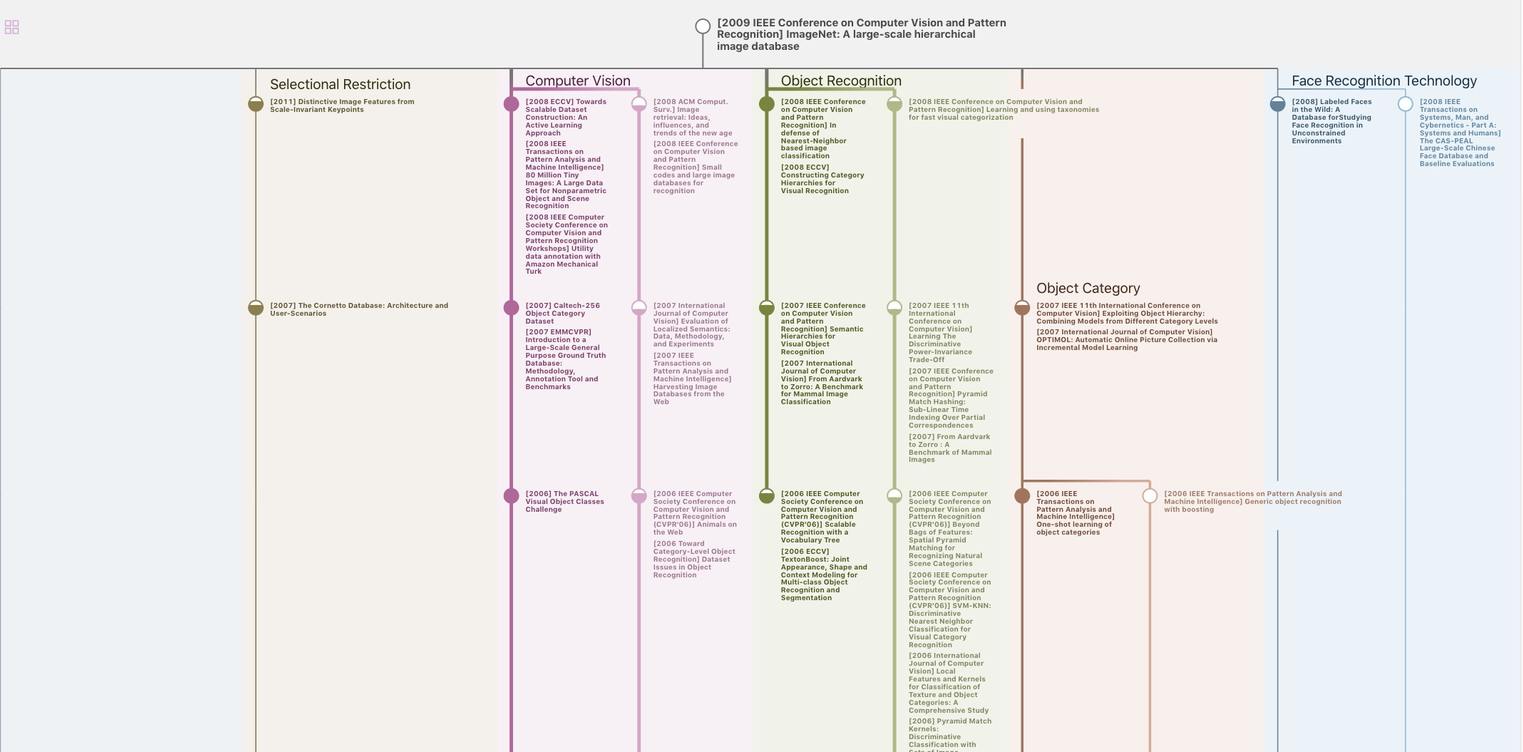
生成溯源树,研究论文发展脉络
Chat Paper
正在生成论文摘要