Adversarial Dynamic Load-Altering Cyberattacks Against Peak Shaving Using Residential Electric Water Heaters
IEEE TRANSACTIONS ON SMART GRID(2024)
摘要
Innovative smart grid technologies such as demand-side management (DSM) and smart home systems promise more optimal energy consumption; however, they expose the grid to load-altering cyberattacks wherein adversaries take control of customers' connected high-wattage appliances and consequently induce harmful load fluctuations. In this article, we present a novel adversarial machine learning algorithm powered by black-box optimization to synthesize stealthy dynamic load-altering attacks aiming at evading existing intrusion detection measures while maximizing the damage inflicted on the grid. The proposed algorithm is applied on a case study involving peak shaving using direct load control of residential electric water heaters. It is capable of designing stealthy attacks that can increase the detectors' misclassification probability by up to 44%. It can also be adapted to other load-altering attacks involving different DSM programs or other classes of loads. The algorithm can be used by electric power utilities to evaluate and harden the robustness of their cybersecurity solutions.
更多查看译文
关键词
Smart grid,cybersecurity,peak shaving,demand-side management,intrusion detection,adversarial machine learning,adversarial training,time-delay neural networks,black-box optimization
AI 理解论文
溯源树
样例
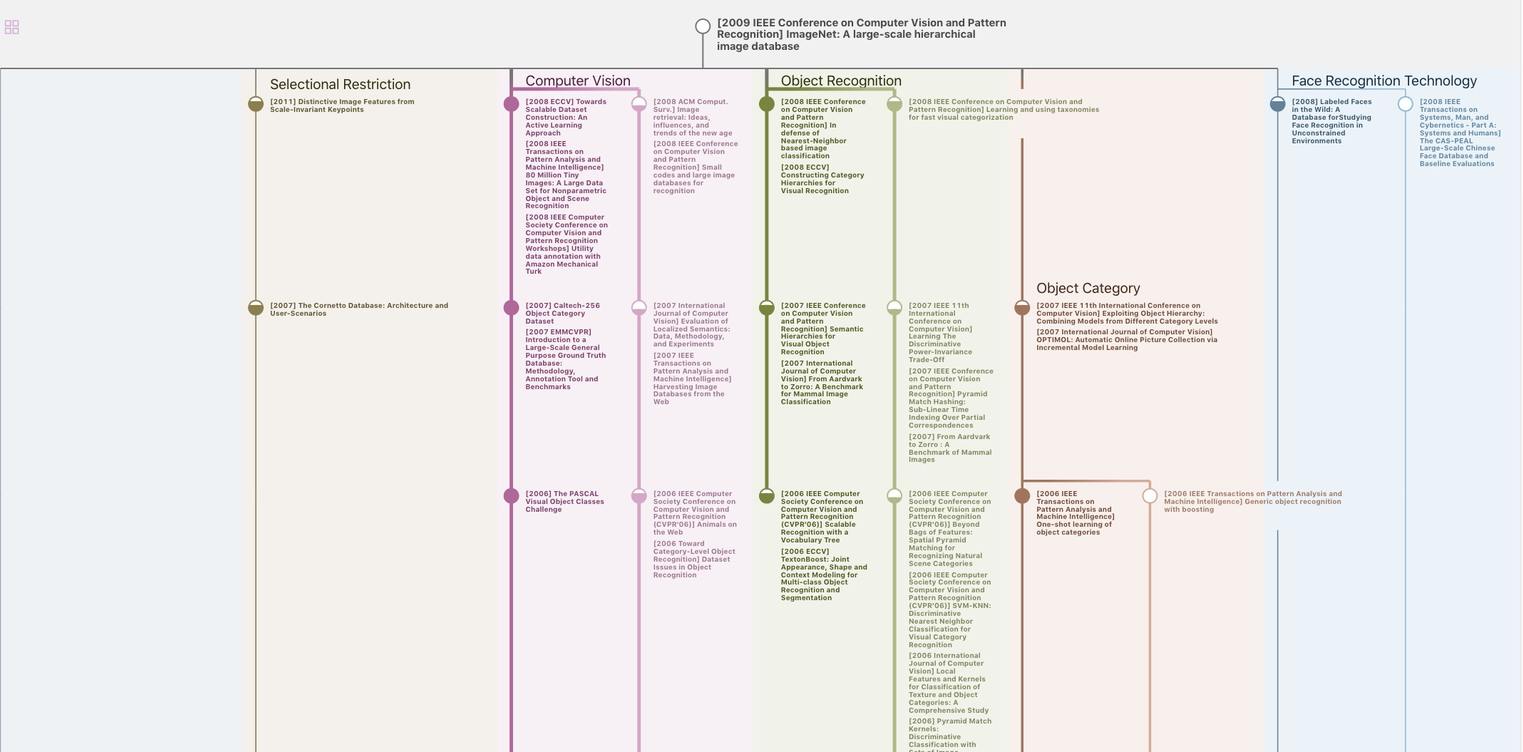
生成溯源树,研究论文发展脉络
Chat Paper
正在生成论文摘要