Photodynamics With Neural Networks and Kernel Ridge Regression
Elsevier eBooks(2024)
摘要
Photodynamics simulations can unravel the secrets and intricacies of photochemistry in an intuitive way. These simulations can be carried out efficiently, if machine learning is used to obtain the excited-state properties that steer the dynamics, like energies, forces, nonadiabatic couplings, spin-orbit couplings or dipole moments. In the present chapter, an introduction to such machine-learning based photodynamics simulations is given. The basics of the most commonly used machine learning techniques, neural networks and kernel ridge regression, are provided. The tricks and pitfalls when predicting excited-state properties with these machine learning techniques are discussed.
更多查看译文
关键词
kernel ridge regression,neural networks
AI 理解论文
溯源树
样例
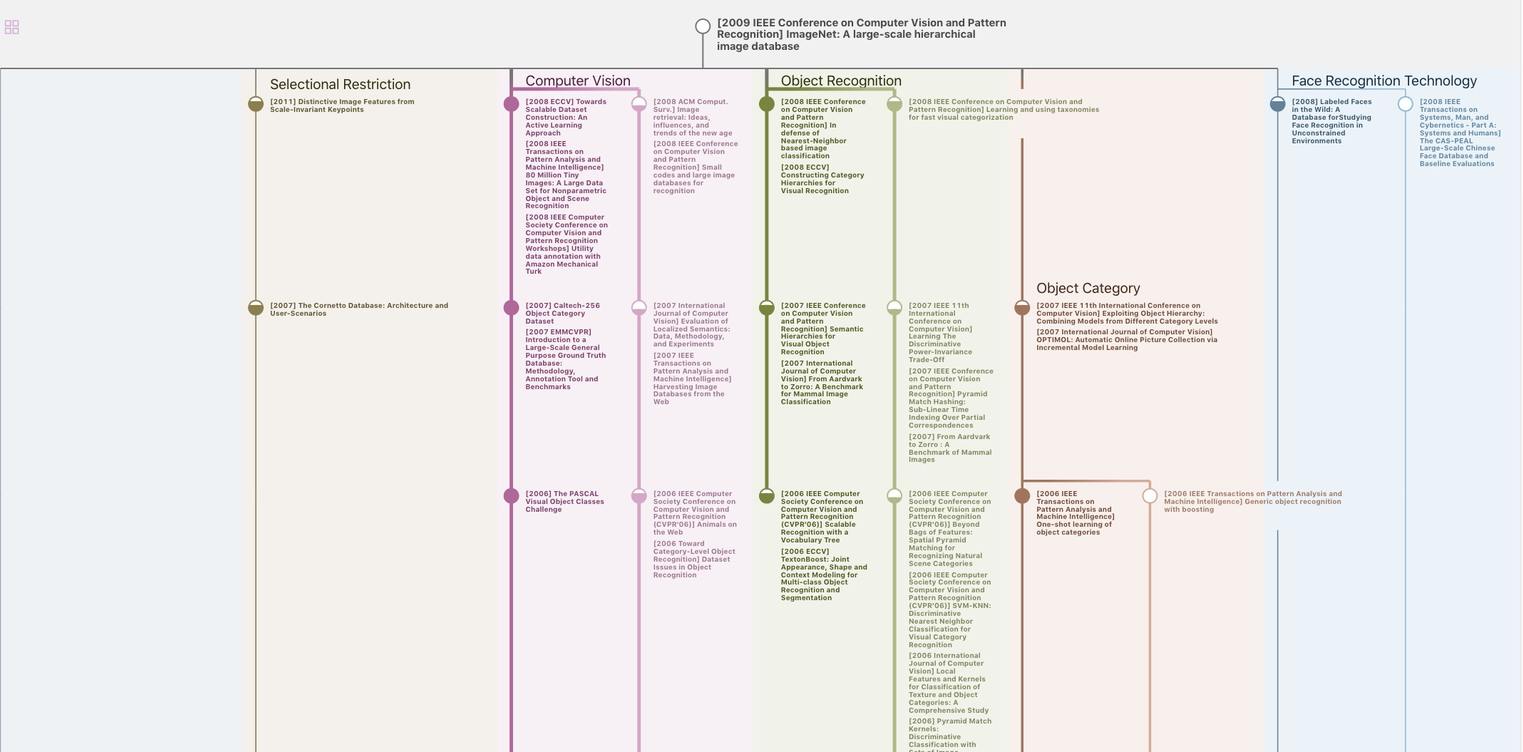
生成溯源树,研究论文发展脉络
Chat Paper
正在生成论文摘要