Data-Driven Fast Charging Optimization for Lithium-Ion Battery Using Bayesian Optimization with Fast Convergence
IEEE Transactions on Transportation Electrification(2023)
摘要
Search for the minimum charging time without damaging the batteries is one of the most crucial challenges in electric vehicles. Optimization using electrochemical models achieves this goal by solving a large-scale constrained optimal control problem. However, the high dimensionality of possible charging protocols, high computational complexity, and uncertainty in model identification have become the major obstacles limiting model-based methods. As an alternative, data-driven solutions using battery test data can be employed, but they are expensive in terms of test time, equipment, and cost of cells. With the aim of reducing the test time and cost of cells, this paper proposes a data-driven fast-charging optimization scheme using Bayesian optimization with fast convergence. In particular, this paper focuses on a hybrid Bayesian optimization algorithm by combining mesh grid adaptive search. The proposed algorithm can explicitly deal with battery safety constraints and achieve competitive performance with an affordable computational and test overhead. The proposed algorithm is model-free by regarding battery fast-charging control as a black-box optimization problem. Different search strategies and acquisition functions are evaluated on multiple constant charge protocols. Results verify the effectiveness of the proposed algorithm in terms of a minimum charging time and fast convergence speed.
更多查看译文
关键词
Fast charging,Battery management system,Bayesian Optimization,Data-driven
AI 理解论文
溯源树
样例
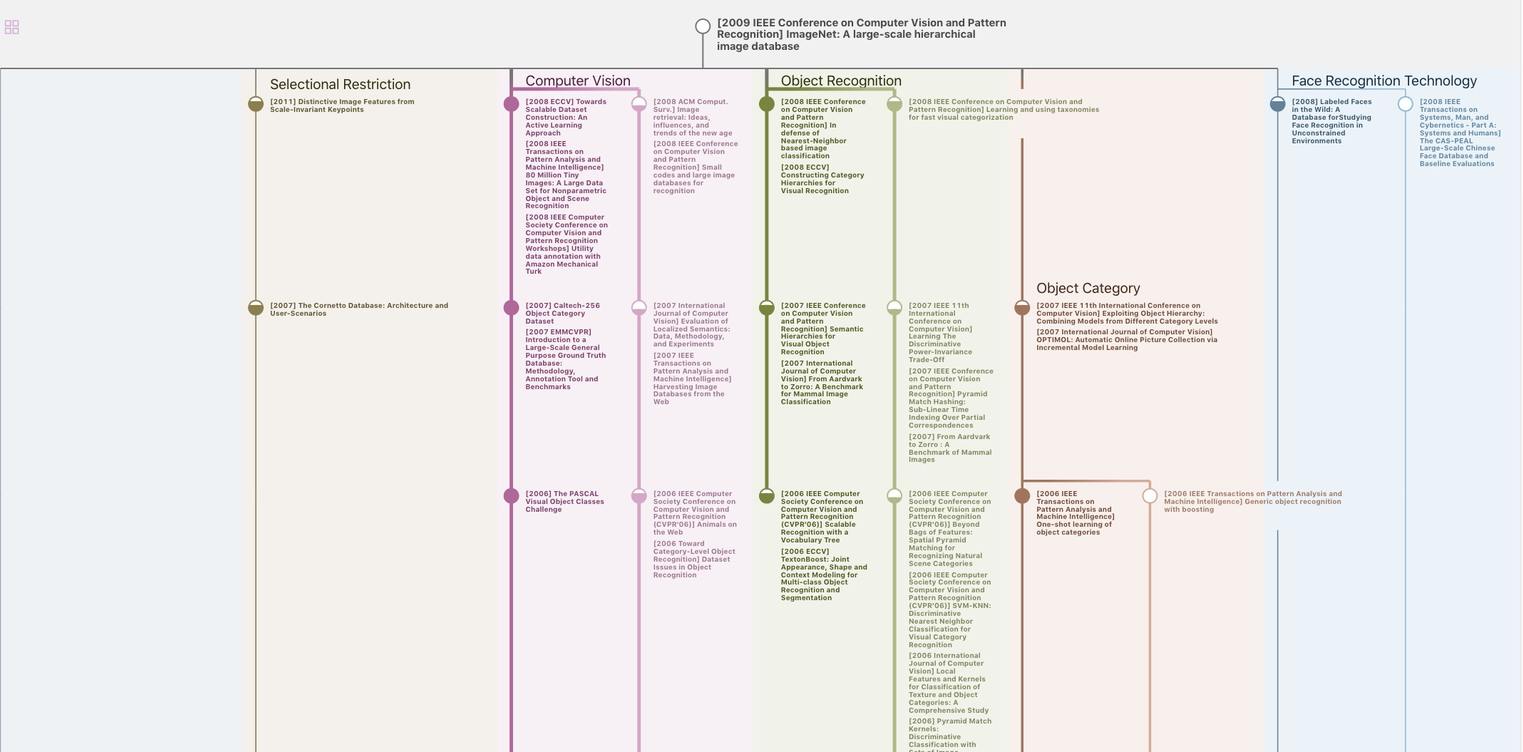
生成溯源树,研究论文发展脉络
Chat Paper
正在生成论文摘要