Regulatory Arbitrage or Random Errors? Implications of Race Prediction Algorithms in Fair Lending Analysis
Social Science Research Network(2023)
摘要
When race is not directly observed, regulators and analysts commonly predict it using algorithms based on last name and address. In small business lending—where regulators assess fair lending law compliance using the Bayesian Improved Surname Geocoding (BISG) algorithm—we document large prediction errors among Black Americans. The errors bias measured racial disparities in loan approval rates downward by 43%, with greater bias for traditional vs. fintech lenders. Regulation using self-identified race would increase lending to Black borrowers, but also shift lending toward affluent areas because errors correlate with socioeconomics. Overall, using race proxies in policymaking and research presents challenges.
更多查看译文
关键词
Racial disparities,Disparate impact analysis,Race-conscious policies,Race prediction,Small business lending,BISG
AI 理解论文
溯源树
样例
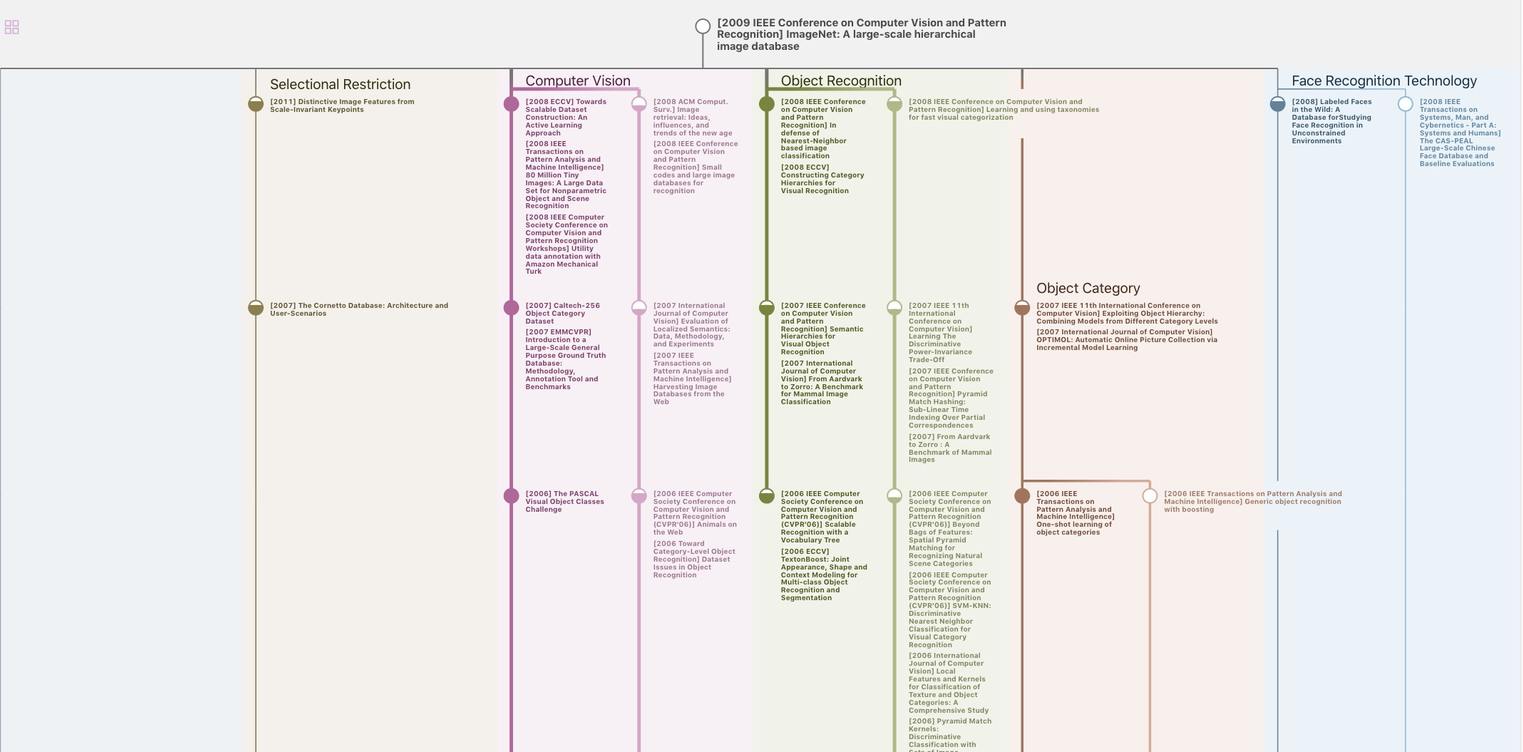
生成溯源树,研究论文发展脉络
Chat Paper
正在生成论文摘要