EGCN: A Node Classification Model Based on Transformer and Spatial Feature Attention GCN for Dynamic Graph
ARTIFICIAL NEURAL NETWORKS AND MACHINE LEARNING, ICANN 2023, PT VI(2023)
摘要
Node classification is an important area in graph data-related research and has attracted the attention of many researchers. Graph structure data can be divided into static graphs and dynamic graphs. Due to the temporal characteristics of dynamic graphs, dynamic graphs have higher information density and richer data features than static graph data. In order to better complete the extraction of the features for dynamic graphs, we design a comprehensive feature extraction module and then create the dynamic graph node classification model EGCN. The introduced time dimension in the dynamic graph not only brings the temporal feature but also brings the implicit spatial feature, which is mostly ignored in related research. So we propose a method for modeling implicit spatial features and combine it with GCN to introduce SAGCN for implicit spatial feature attention. We also introduce a temporal feature extraction module called TEncoder based on the transformer and combine them to design the dynamic graph node classification model EGCN. EGCN has two variants, EGCN-S and EGCN-T, which differ in their temporal and spatial characteristics of attention. Experiments show that EGCN achieves state-of-the-art performance. EGCN-S and EGCNT achieved 1% and 5% improvement over the main baseline DS-TAGCN on dataset DBLP.
更多查看译文
关键词
dynamic graph,node classification,graph convolutional network
AI 理解论文
溯源树
样例
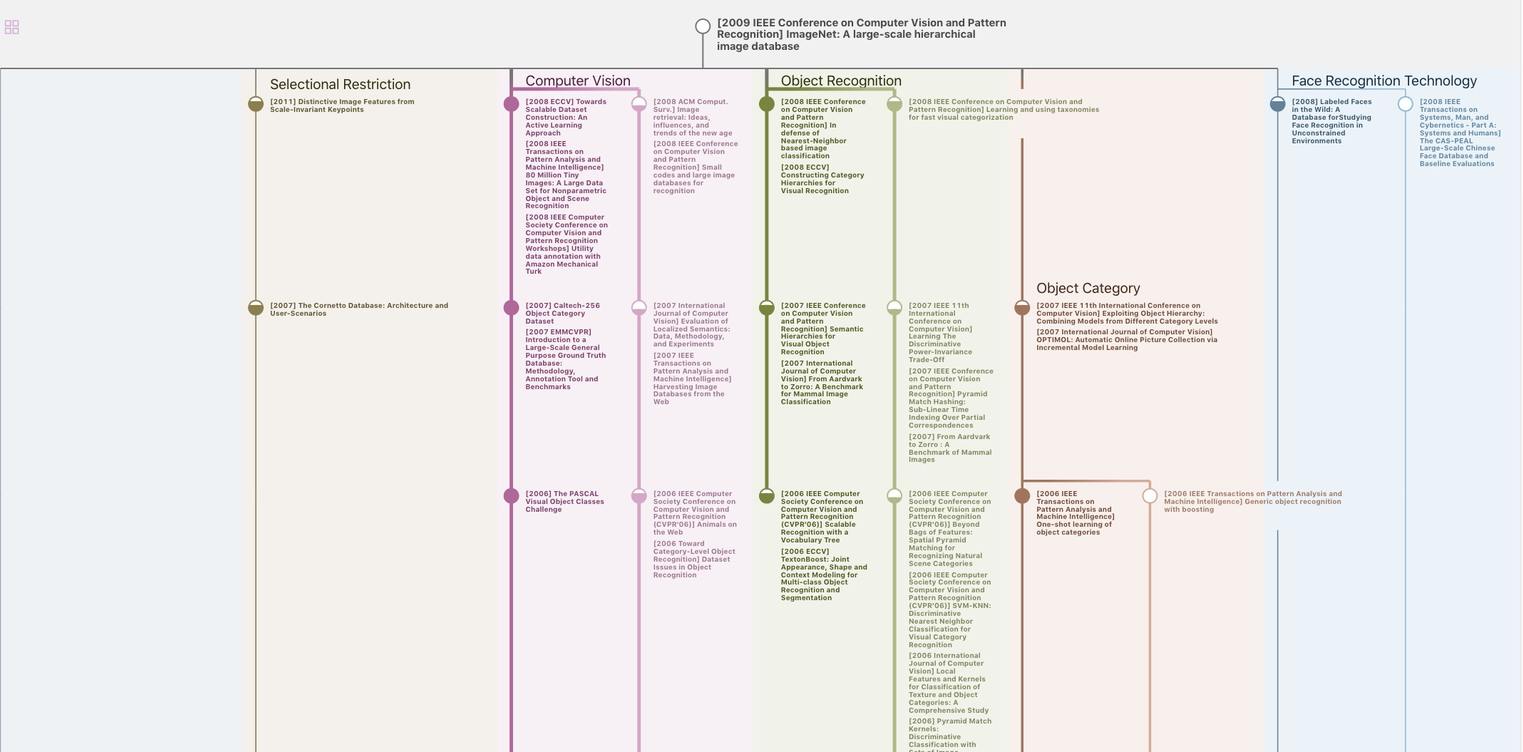
生成溯源树,研究论文发展脉络
Chat Paper
正在生成论文摘要