DCE-Net: A Dynamic Context Encoder Network for Liver Tumor Segmentation
Research Square (Research Square)(2023)
摘要
Abstract Segmentation of a tumor region from medical images is critical for clinical diagnosis and the planning of surgical treatments. Recent advancements in machine learning have shown that convolutional neural networks are powerful in such image processing while largely reducing human labor. However, the variant shapes of liver tumors with blurred boundaries in medical images cause a great challenge for accurate segmentation. The feature extraction capability of a neural network can be improved by expanding its architecture, but it inevitably demands more computing resources in training and hyperparameter tuning. This paper presents a Dynamic Context Encoder Network (DCE-Net), which incorporated multiple new modules, such as the Involution Layer, Dynamic Residual Module, Context Extraction Module, and Channel Attention Gates, for feature extraction and enhancement. In the experiment, we adopted a liver tumor CT dataset of LiTS2017 to train and test the DCE-Net for liver tumor segmentation. Experimental results showed that the precision, recall, Dice, and AUC were 0.8961, 0.9711, 0.9270, and 0.9875, respectively, outperforming other state-of-the-art networks. Furthermore, our ablation study shows that the accuracy and training efficiency are markedly superior to those networks without involution or dynamic residual modules. Therefore, the proposed DCE-Net has potential for the automatic segmentation of liver tumors in clinical settings.
更多查看译文
关键词
liver tumor segmentation,dynamic context encoder network,dce-net
AI 理解论文
溯源树
样例
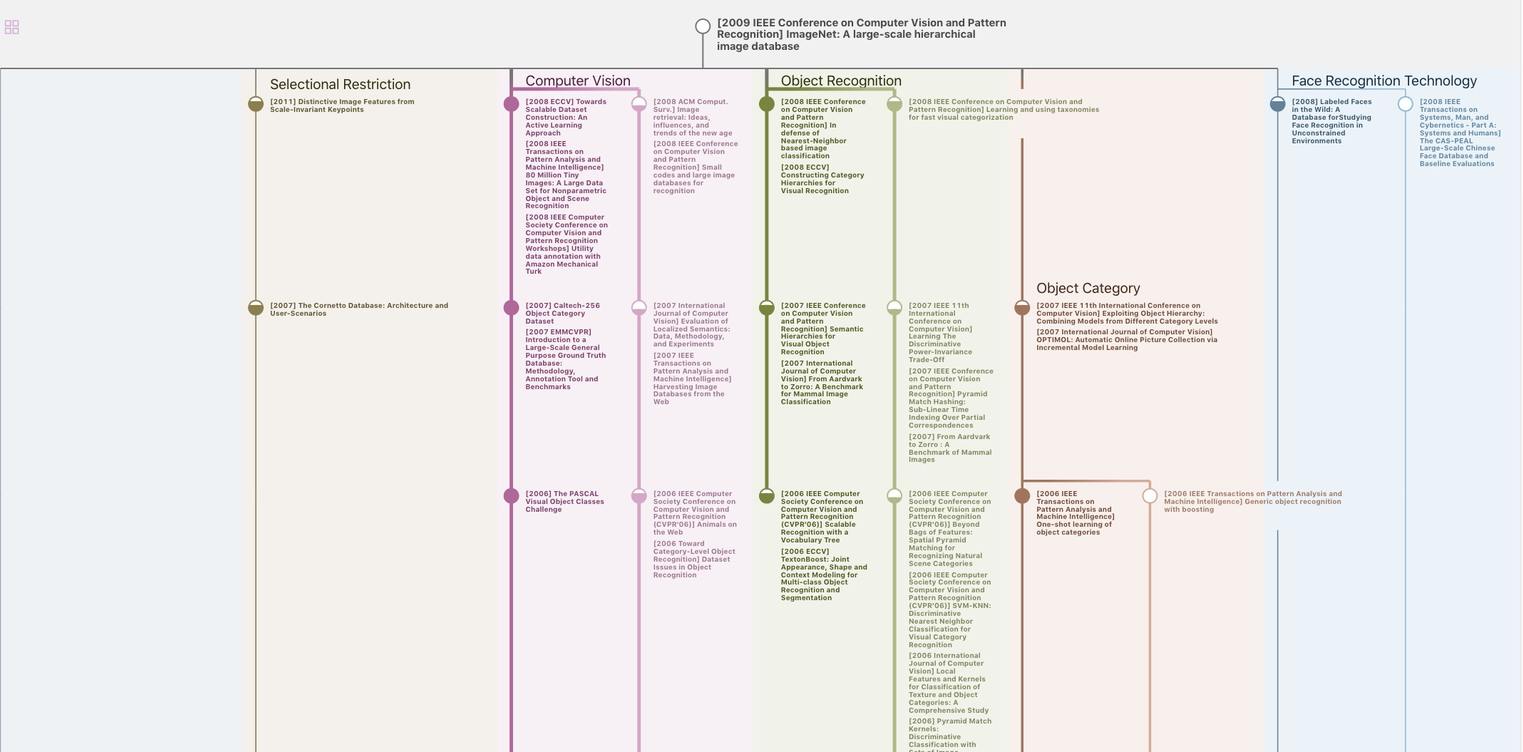
生成溯源树,研究论文发展脉络
Chat Paper
正在生成论文摘要