Mathematical Models of Human Learning
Cambridge University Press eBooks(2023)
摘要
Although learning was a key focus during the early years of mathematical psychology, the cognitive revolution of the 1960s caused the field to languish for several decades. Two breakthroughs in neuroscience resurrected the field. The first was the discovery of long-term potentiation and long-term depression, which served as promising models of learning at the cellular level. The second was the discovery that humans have multiple learning and memory systems that each require a qualitatively different kind of model. Currently, the field is well represented at all of Marr’s three levels of analysis. Descriptive and process models of human learning are dominated by two different, but converging, approaches – one rooted in Bayesian statistics and one based on popular machine-learning algorithms. Implementational models are in the form of neural networks that mimic known neuroanatomy and account for learning via biologically plausible models of synaptic plasticity. Models of all these types are reviewed, and advantages and disadvantages of the different approaches are considered.
更多查看译文
关键词
learning,models,human
AI 理解论文
溯源树
样例
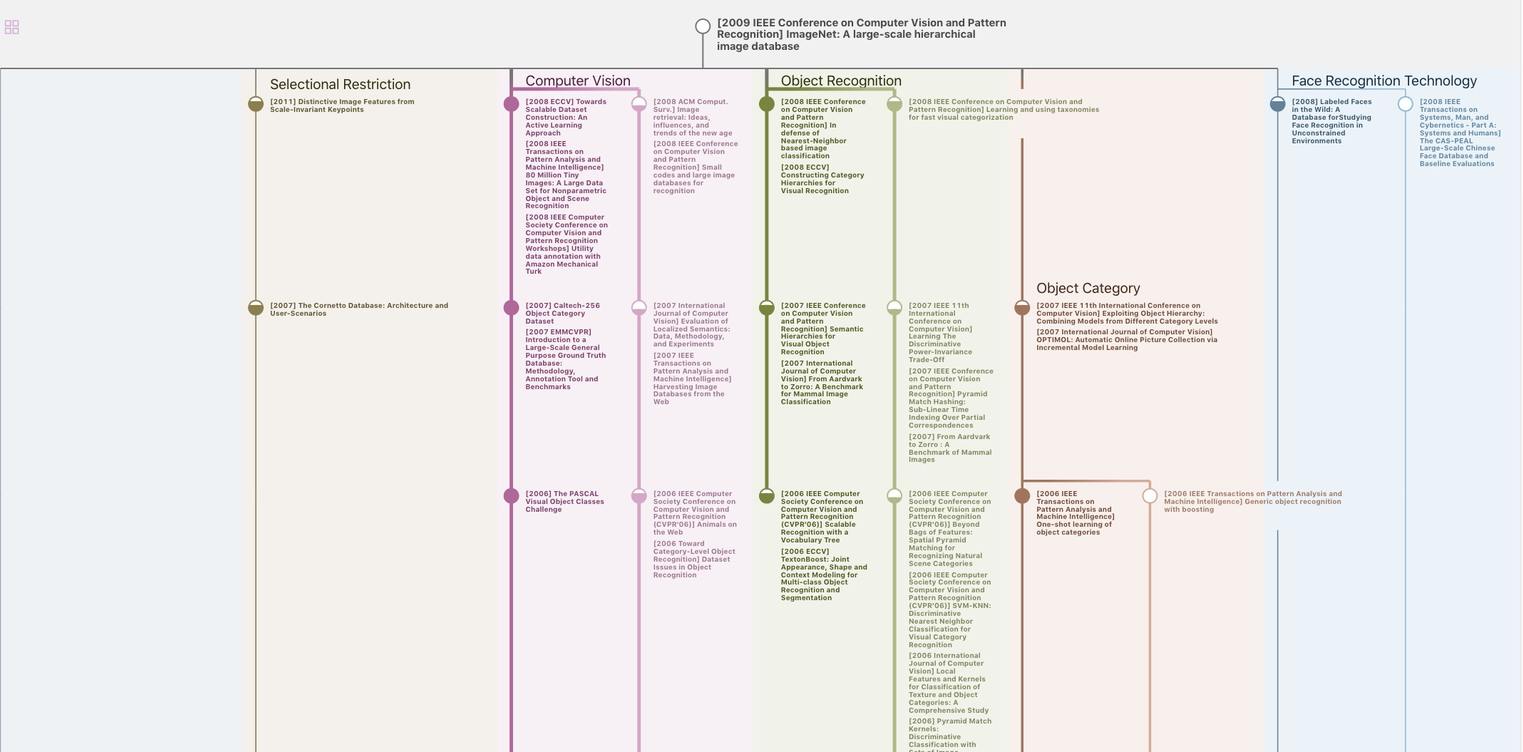
生成溯源树,研究论文发展脉络
Chat Paper
正在生成论文摘要