Abstract P2-11-08: Multimodal Prediction of Breast Cancer Recurrence Assays and Risk of Recurrence
Cancer Research(2023)
摘要
Background: Hormone receptor positive breast cancer constitutes about 70% of newly diagnosed early-stage disease in the United States, and gene-expression based recurrence assays such as Oncotype DX (ODX) are strongly recommended by guidelines to aid in treatment decisions. However, recurrence assays are costly, time-consuming, underutilized in low resource settings, and unavailable in developing countries. Deep Learning (DL) using hematoxylin and eosin (H&E) stained digital pathology has been shown to approximate gene expression patterns for multiple cancer types, and may provide a cost-effective, fast, and scalable method to predict risk of recurrence in community settings. Methods: We first developed a model for ODX using only DL on pathology, comprised of two Xception-based modules, trained on 1,039 slides from The Cancer Genome Atlas (TCGA) tessellated into 10x magnification image tiles. The first module predicts tumor likelihood, and was trained using pathologist annotations for tumor regions versus normal stroma. The second module was trained to predict ODX score, estimated from gene expression data within TCGA. Patient-level predictions were calculated by weighting the predicted recurrence score by tumor likelihood for all tiles within a slide. Separately, ODX score was predicted from clinical variables using the University of Tennessee Nomogram, which incorporates grade, progesterone receptor, size, age, and histologic subtype. Finally, we developed a combined model by fitting a logistic regression to the DL pathologic model and the clinical nomogram predictions. Performance of the clinical nomogram, pathologic, and combined models were then compared in a single-institution external validation cohort of patients diagnosed with breast cancer between 2006 and 2020, all of whom had the commercial ODX assay run. Results: We identified 428 cases for our diverse validation cohort (69% White, 24% Black, 6% Asian, and 3% Hispanic) with mean ODX score of 18. Chemotherapy was administered for 104 (24.3%) of patients, the remaining 323 (75.4%) received endocrine therapy alone. Area under the receiver operating characteristic curve (AUROC) for prediction of high ODX score (≥ 26) of the combined model was 0.83 (95% confidence interval [CI] 0.78 – 0.89) in the validation cohort, which was higher than either the DL pathology model (AUROC 0.80, 95% CI 0.75 – 0.85, p = 0.026) or the Tennessee nomogram (AUROC 0.77, 85% CI 0.70 – 0.83, p = 0.003). Performance was similar in Black (AUROC 0.86, 95% CI 0.78 – 0.94) and White (AUROC of 0.81, 95% CI 0.74 – 0.88) subgroups. The combined model was more accurate in prediction of recurrence-free interval in patients receiving endocrine therapy (hazard ratio [HR] 2.02 per standard deviation [SD], 95% CI 1.16 – 3.52, p = 0.013, Concordance [C]-index 0.75) than the clinical nomogram (HR 1.75 per SD, 95% CI 1.09 – 2.81, p = 0.021, C-index 0.68). No model was prognostic in patients receiving chemotherapy. Pathologist review of heatmaps of DL model predictions identified lymphovascular invasion, necrosis, high grade, and infiltrative borders as features contributing to model prediction of high risk. Conclusions: DL can improve on existing clinical prediction of breast cancer with low recurrence risk. This approach could improve the speed at which treatment decisions are made due to the time-consuming nature of genomic testing and simultaneously reduce the cost of care. Given the equal performance in racial subgroups, this approach has promise for application in global health settings where genomic assays are not widely available or are prohibitively expensive. Citation Format: Frederick M. Howard, James M. Dolezal, Sara Kochanny, Galina Khramtsova, Jasmine Vickery, Andrew Srisuwananukorn, Anna Woodard, Nan Chen, Rita Nanda, Charles Perou, Olufunmilayo I. Olopade, Dezheng Huo, Alexander Pearson. Multimodal Prediction of Breast Cancer Recurrence Assays and Risk of Recurrence [abstract]. In: Proceedings of the 2022 San Antonio Breast Cancer Symposium; 2022 Dec 6-10; San Antonio, TX. Philadelphia (PA): AACR; Cancer Res 2023;83(5 Suppl):Abstract nr P2-11-08.
更多查看译文
关键词
breast cancer recurrence assays,breast cancer,multimodal prediction
AI 理解论文
溯源树
样例
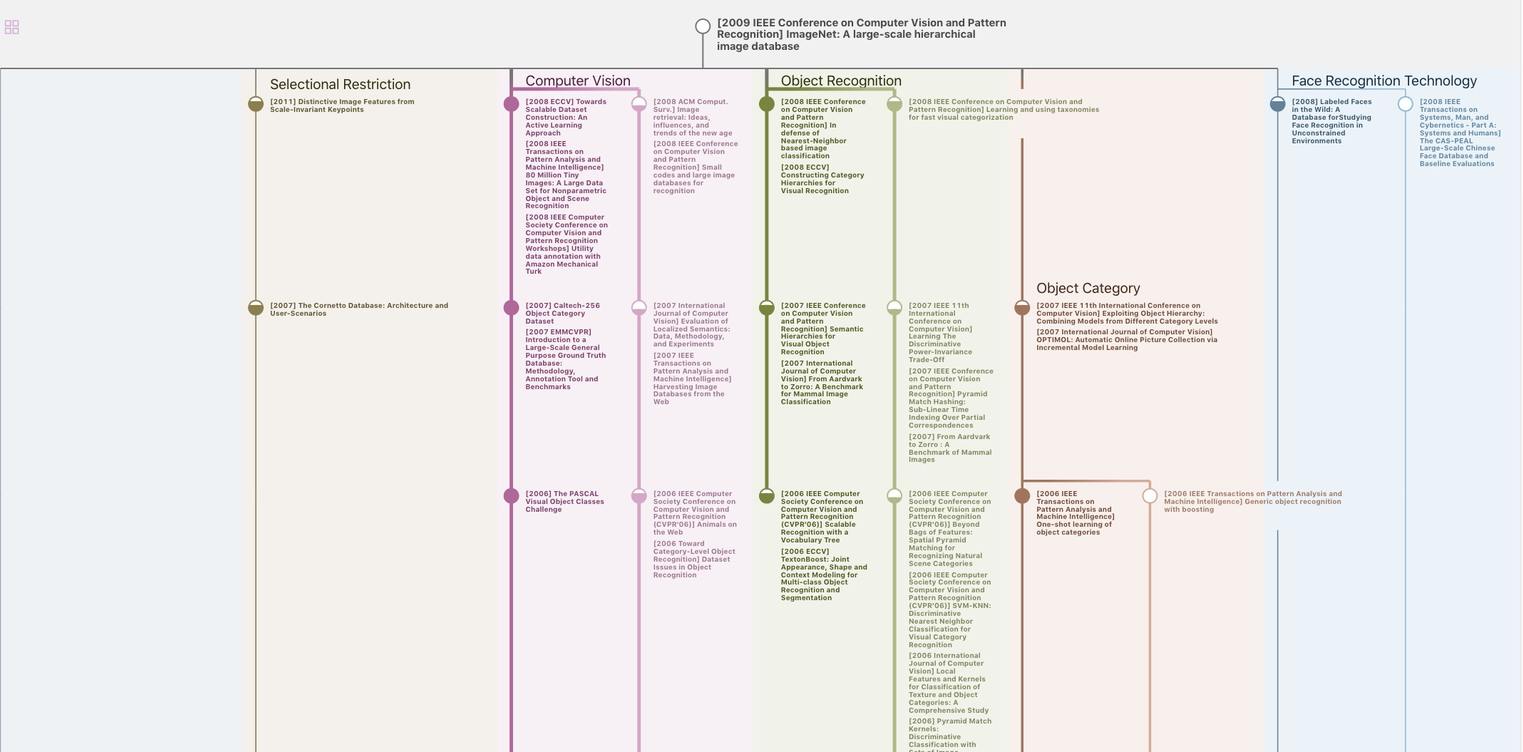
生成溯源树,研究论文发展脉络
Chat Paper
正在生成论文摘要