Gradient-free adversarial attack algorithm based on differential evolution
INTERNATIONAL JOURNAL OF BIO-INSPIRED COMPUTATION(2023)
摘要
Deep learning models are susceptible to adversarial examples even in the black-box setting. This means there are security risks in intelligent systems based on deep learning. Research on adversarial attacks is crucial to improving the robustness of deep learning models. Most of the existing algorithms are query-intensive and require models to provide more detailed results. We focus on a restrictive threat model and propose a gradient-free adversarial attack algorithm based on differential evolution. In particular, we design two fitness functions to achieve targeted attacks and non-targeted attacks. And we introduce an elimination mechanism in the selection phase to speed up the convergence of the algorithm. Experiments on MNIST, CIFAR-10, and ImageNet show the effectiveness of the proposed method. The comparison with C&W, ZOO and GenAttack shows our method has better advantages in the attack success rate, the number of queries required for a successful attack, and the information obtained in a single query.
更多查看译文
关键词
black-box adversarial attack,partial information setting,differential evolution,gradient-free
AI 理解论文
溯源树
样例
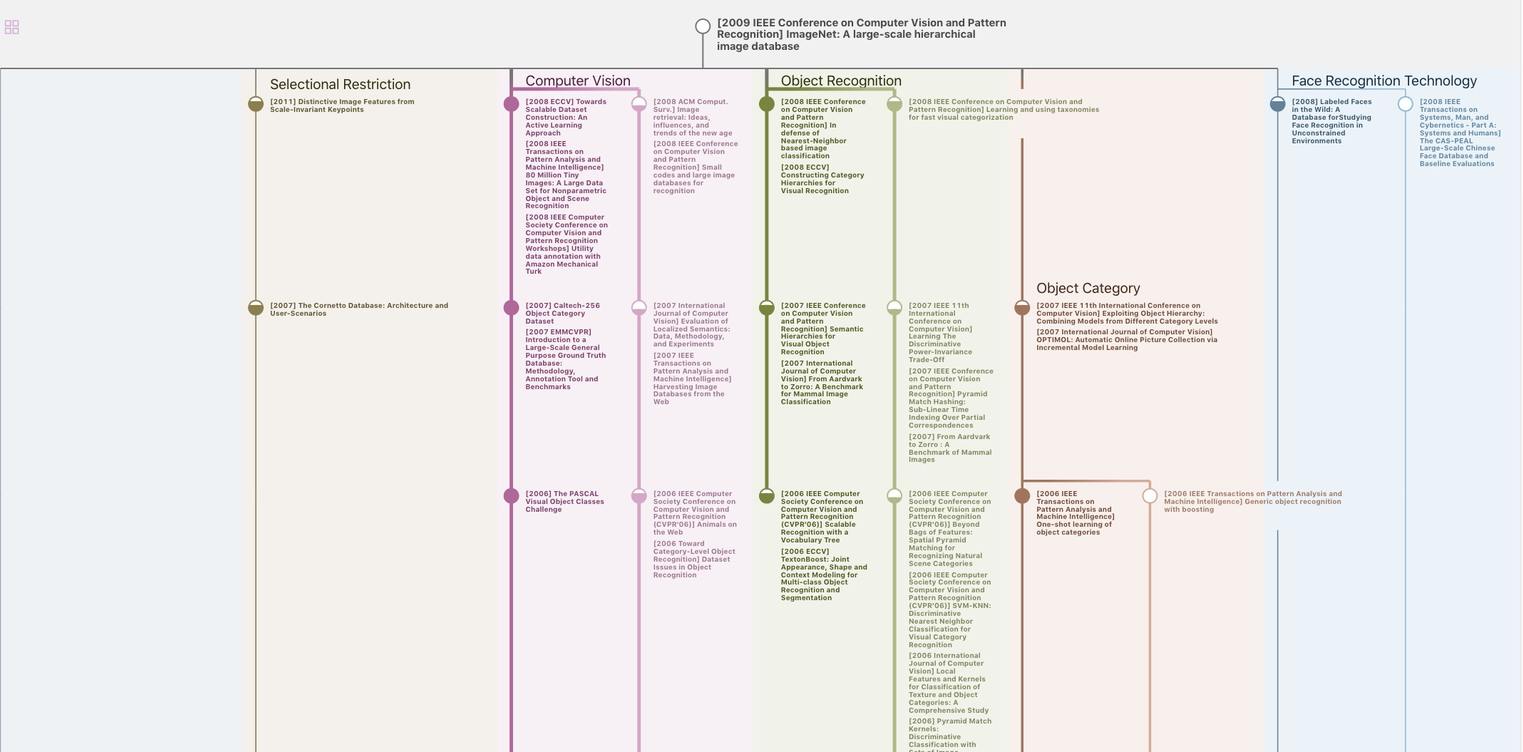
生成溯源树,研究论文发展脉络
Chat Paper
正在生成论文摘要