Lasso and Friends
Statistics and computing(2023)
摘要
Regularized linear models are generalized linear regression models with a penalty for large coefficients to regulate the bias-variance tradeoff. For Gaussian linear regression, choosing an L2 penalty leads to ridge regression and choosing an L1 penalty leads to the Lasso. The same penalties can be applied to logistic regression. Both penalties tend to reduce the magnitude of coefficients. Because the L1 penalty can reduce coefficients to zero, the L1 penalty can be used to for variable selection. The elastic net is a mixture of a model with an L1 penalty and an L2 penalty. The case study estimates birth weight as a function of the smoking status of the mother and socio-demographic variables of both parents.
更多查看译文
AI 理解论文
溯源树
样例
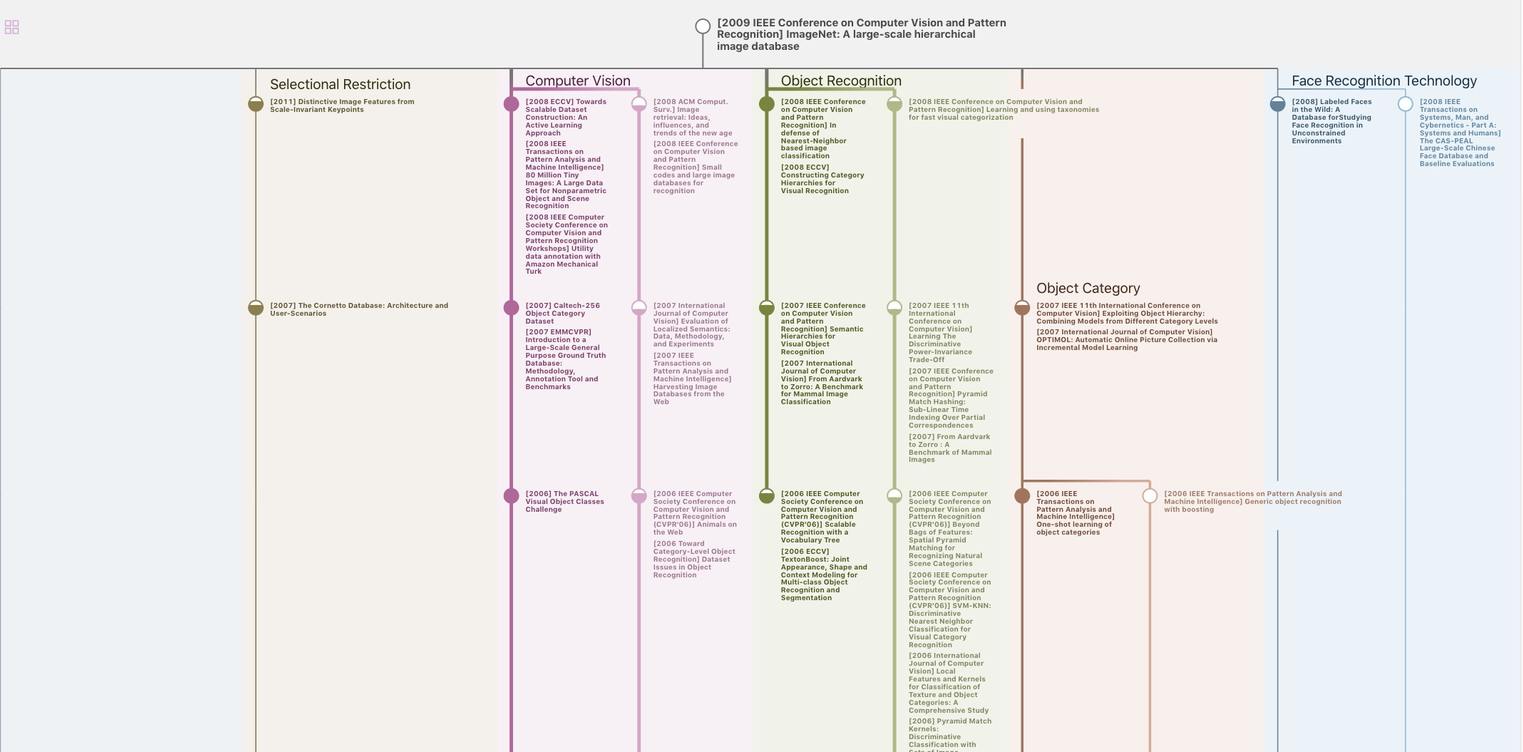
生成溯源树,研究论文发展脉络
Chat Paper
正在生成论文摘要