Application of dimension truncation error analysis to high-dimensional function approximation in uncertainty quantification
arXiv (Cornell University)(2023)
Abstract
Parametric mathematical models such as parameterizations of partial differential equations with random coefficients have received a lot of attention within the field of uncertainty quantification. The model uncertainties are often represented via a series expansion in terms of the parametric variables. In practice, this series expansion needs to be truncated to a finite number of terms, introducing a dimension truncation error to the numerical simulation of a parametric mathematical model. There have been several studies of the dimension truncation error corresponding to different models of the input random field in recent years, but many of these analyses have been carried out within the context of numerical integration. In this paper, we study the $L^2$ dimension truncation error of the parametric model problem. Estimates of this kind arise in the assessment of the dimension truncation error for function approximation in high dimensions. In addition, we show that the dimension truncation error rate is invariant with respect to certain transformations of the parametric variables. Numerical results are presented which showcase the sharpness of the theoretical results.
MoreTranslated text
Key words
dimension truncation error analysis,uncertainty,function approximation,quantification,high-dimensional
AI Read Science
Must-Reading Tree
Example
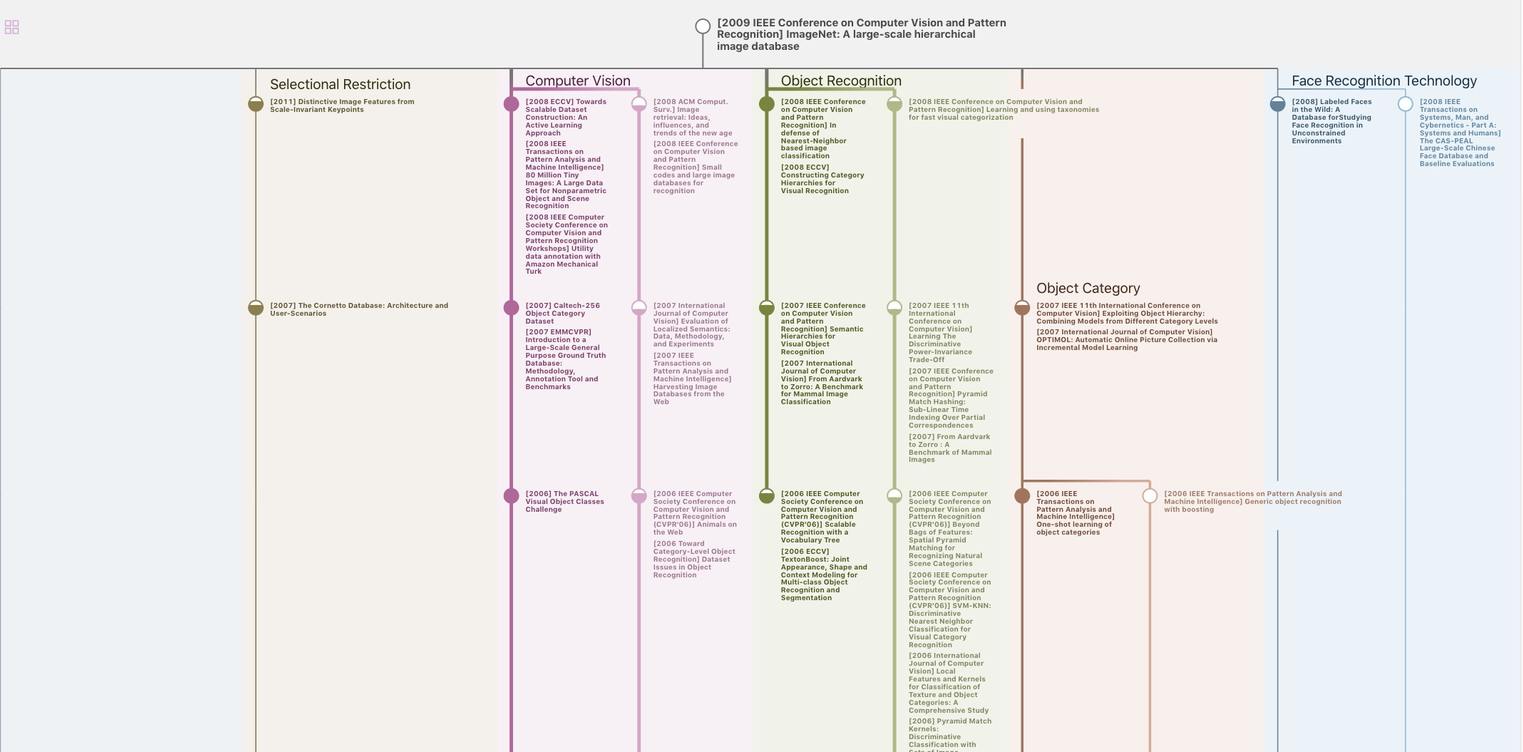
Generate MRT to find the research sequence of this paper
Chat Paper
Summary is being generated by the instructions you defined