Using Proportional Jaccard Indices to Identify Comorbidity Patterns of Heart Failure
Research Square (Research Square)(2023)
摘要
Abstract Remote diagnosis and precision preventive medicine have become some of the most important clinical medicine applications in the post-COVID-19 era. This study aims to develop a digital health monitoring tool using electronic medical records (EMRs) as the basis for conducting non-random correlation analysis among different comorbidity patterns for heart failure (HF). Novel similarity indices, including the multiplication of the odds ratio, proportional Jaccard index (OPJI), and alpha proportional Jaccard index (APJI), were proposed and used as key indicators to build various machine learning models for predicting HF risk conditions. Multiple prediction models were constructed for high-risk HF predictions according to stratified subjects in different age groups and sexes. The results showed that the best prediction model achieved an accuracy of 82.1% and an AUC of 0.87. A noninvasive prediction system for HF risk conditions was proposed using historical EMRs. The proposed indices provide simple and straightforward comparative indicators for comorbidity pattern-matching based on personal EMRs. All of the developed source codes for the noninvasive prediction models can be retrieved from GitHub 1 .
更多查看译文
关键词
heart failure,proportional jaccard indices,comorbidity patterns
AI 理解论文
溯源树
样例
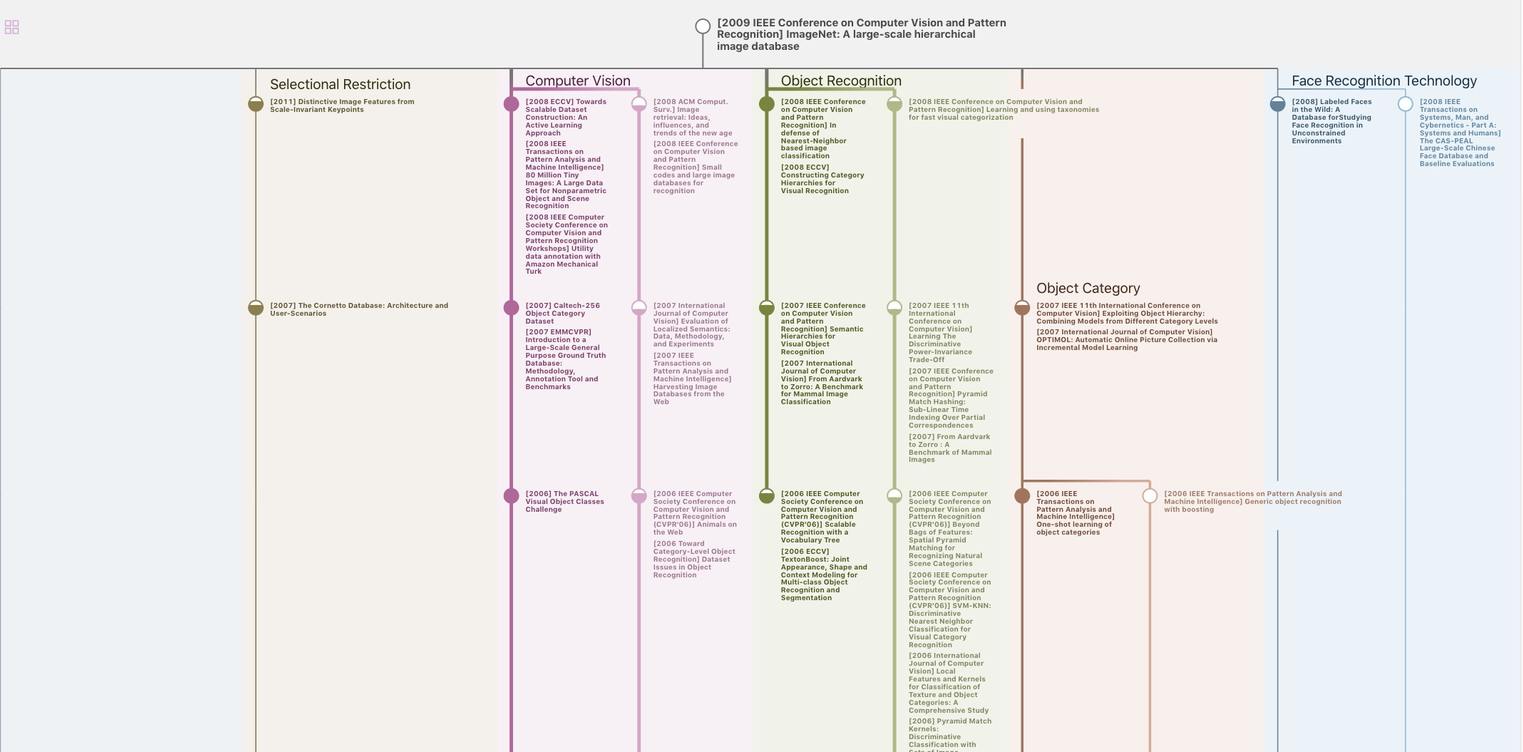
生成溯源树,研究论文发展脉络
Chat Paper
正在生成论文摘要