A novel automated feature selection based approach to recognize cauliflower disease
Bulletin of Electrical Engineering and Informatics(2023)
摘要
Cauliflower disease is a primary cause of reduced cauliflower yield. Preventing cauliflower disease requires early diagnosis. In the scope of this study, we suggested an agro-medical expert system that would make it easier to diagnose cauliflower disease. In this method, a digital image must be taken off the phone or handled device to diagnose cauliflower sickness. A data augmentation technique was initially used to construct a vast data set. The disease-affected parts of the cauliflower were then segmented using k-means clustering. Following that, ten statistical and gray-level co-occurrence matrix (GLCM) features were retrieved from the segmented pictures. After choosing the top n features (N ranged from 5 to 10), the synthetic minority oversampling technique (SMOTE) approach was used to handle training datasets with different amounts of each feature. After that, we utilized five machine learning (ML) algorithms and evaluated their performance using seven performance evaluation matrices for both augmented and non-augmented datasets. The same procedure was performed on both datasets. Then, we use both datasets to test how well the classifier works. Logistic regression (LR) is the most accurate method for the top nine features in the augmented dataset (90.77%).
更多查看译文
关键词
cauliflower disease,feature selection
AI 理解论文
溯源树
样例
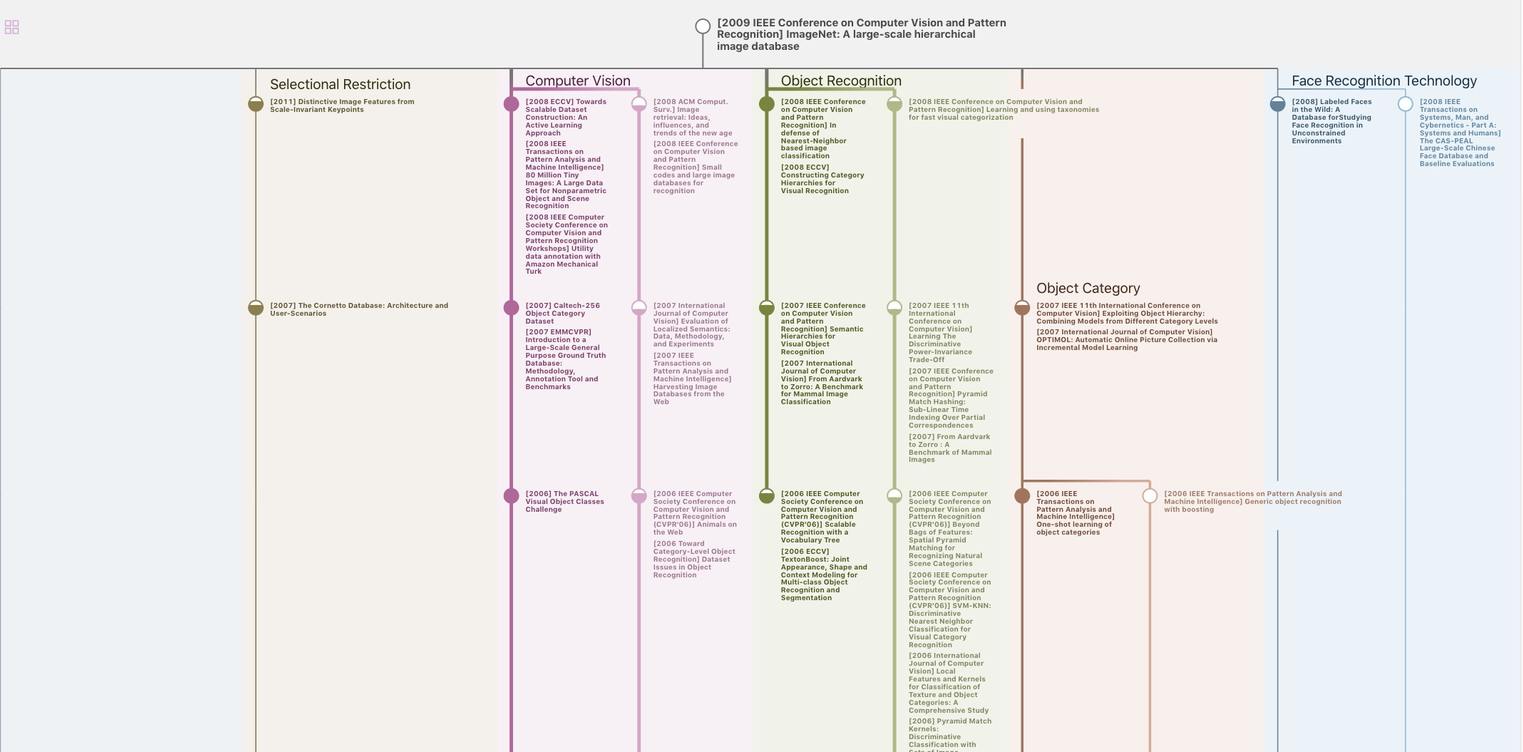
生成溯源树,研究论文发展脉络
Chat Paper
正在生成论文摘要