BadDet: Backdoor Attacks on Object Detection
Lecture Notes in Computer Science(2023)
摘要
Backdoor attack is a severe security threat which injects a backdoor trigger into a small portion of training data such that the trained model gives incorrect predictions when the specific trigger appears. While most research in backdoor attacks focuses on image classification, backdoor attacks on object detection have not been explored but are equally important. Object detection has been adopted as an essential module in various security-sensitive applications such as autonomous driving. Therefore, backdoor attacks on object detection could pose severe threats to human lives and properties. We propose four backdoor attacks and a backdoor defense method for object detection tasks. These four kinds of attacks can achieve different goals attacking: 1) Object Generation Attack: a trigger can falsely generate an object of the target class; 2) Regional Misclassification Attack: a trigger can change the prediction of a surrounding object to the target class; 3) Global Misclassification Attack: a single trigger can change the predictions of all objects in an image to the target class; and 4) Object Disappearance Attack: a trigger can make the detector fail to detect the object of the target class. We develop appropriate metrics to evaluate the four backdoor attacks on object detection. We perform experiments using two typical object detection models - Faster-RCNN and YOLOv3 on different datasets. Empirical results demonstrate the vulnerability of object detection models against backdoor attacks. We show that even fine-tuning on another benign dataset cannot remove the backdoor hidden in the object detection model. To defend against these backdoor attacks, we propose Detector Cleanse, an entropy-based run-time detection framework to identify poisoned testing samples for any deployed object detector.
更多查看译文
关键词
backdoor attacks,detection,baddet,object
AI 理解论文
溯源树
样例
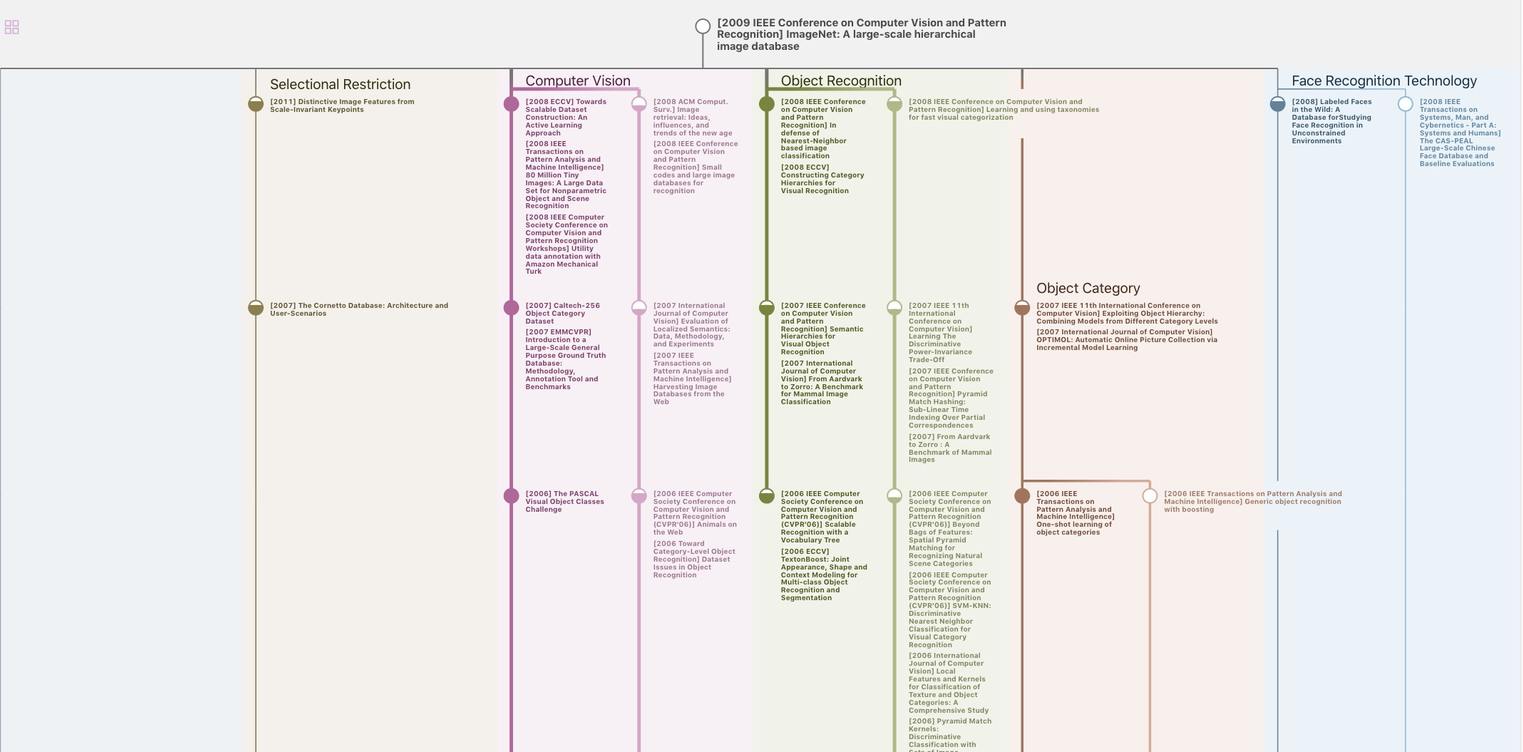
生成溯源树,研究论文发展脉络
Chat Paper
正在生成论文摘要