DeepSWI : Using Deep Learning to Enhance Susceptibility Contrast on T2 *‐Weighted MRI
Journal of Magnetic Resonance Imaging(2023)
摘要
Background Although susceptibility‐weighted imaging (SWI) is the gold standard for visualizing cerebral microbleeds (CMBs) in the brain, the required phase data are not always available clinically. Having a postprocessing tool for generating SWI contrast from T2*‐weighted magnitude images is therefore advantageous. Purpose To create synthetic SWI images from clinical T2*‐weighted magnitude images using deep learning and evaluate the resulting images in terms of similarity to conventional SWI images and ability to detect radiation‐associated CMBs. Study Type Retrospective. Population A total of 145 adults (87 males/58 females; 43.9 years old) with radiation‐associated CMBs were used to train (16,093 patches/121 patients), validate (484 patches/4 patients), and test (2420 patches/20 patients) our networks. Field Strength/Sequence 3D T2*‐weighted, gradient‐echo acquired at 3 T. Assessment Structural similarity index (SSIM), peak signal‐to‐noise‐ratio (PSNR), normalized mean‐squared‐error (nMSE), CMB counts, and line profiles were compared among magnitude, original SWI, and synthetic SWI images. Three blinded raters (J.E.V.M., M.A.M., B.B. with 8‐, 6‐, and 4‐years of experience, respectively) independently rated and classified test‐set images. Statistical Tests Kruskall–Wallis and Wilcoxon signed‐rank tests were used to compare SSIM, PSNR, nMSE, and CMB counts among magnitude, original SWI, and predicted synthetic SWI images. Intraclass correlation assessed interrater variability. P values <0.005 were considered statistically significant. Results SSIM values of the predicted vs. original SWI (0.972, 0.995, 0.9864) were statistically significantly higher than that of the magnitude vs. original SWI (0.970, 0.994, 0.9861) for whole brain, vascular structures, and brain tissue regions, respectively; 67% (19/28) CMBs detected on original SWI images were also detected on the predicted SWI, whereas only 10 (36%) were detected on magnitude images. Overall image quality was similar between the synthetic and original SWI images, with less artifacts on the former. Conclusions This study demonstrated that deep learning can increase the susceptibility contrast present in neurovasculature and CMBs on T2*‐weighted magnitude images, without residual susceptibility‐induced artifacts. This may be useful for more accurately estimating CMB burden from magnitude images alone. Evidence Level 3. Technical Efficacy Stage 2.
更多查看译文
关键词
susceptibility contrast,<scp>mri</scp>,<scp>deepswi</scp> learning
AI 理解论文
溯源树
样例
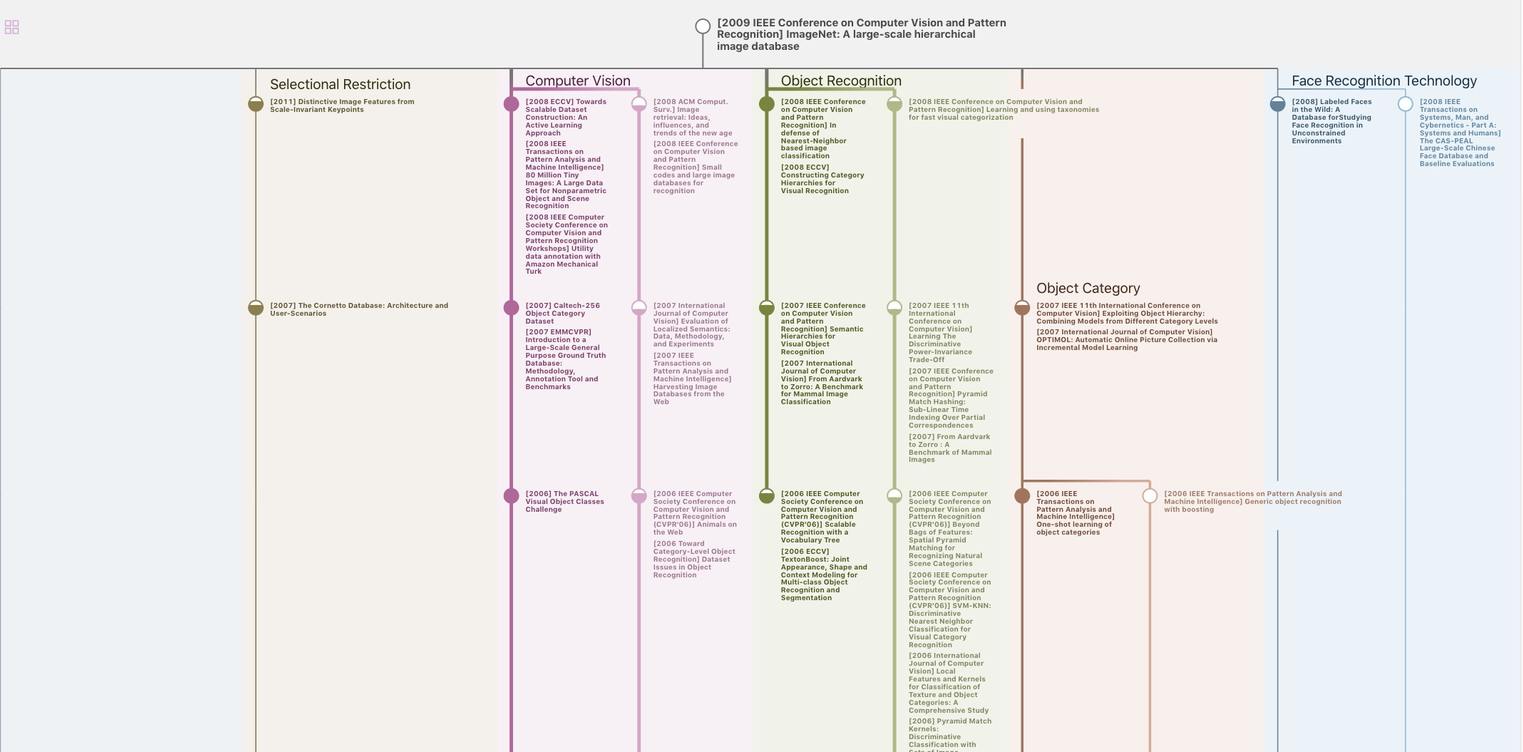
生成溯源树,研究论文发展脉络
Chat Paper
正在生成论文摘要