Compensating small data with large filters for accurate liver vessel segmentation
Research Square (Research Square)(2023)
摘要
Abstract Background: Segmenting liver vessels on computed tomography images is essential for diagnosing liver diseases, planning surgeries and delivering radiotherapy. Nevertheless, identifying vessels is a challenging task due to the tiny cross-sectional areas occupied by vessels, which has posed great challenges for vessel segmentation, such as limited features to be learned and difficult to construct high-quality as well as large-volume data. Methods: We present an approach that only requires a few labeled vessels but delivers significantly improved results. Our model starts with vessel enhancement by fading out liver intensity and generates candidate vessels by a classifier fed with a large number of image filters. Afterwards, the initial segmentation is refined using Markov random fields. Results: In experiments on the well-known dataset 3D-IRCADb, the accuracy is improved to 0.99, and the averaged Dice coefficient is lifted to 0.63. These results are significantly better than those obtained from existing machine-learning approaches and comparable to those generated from deep-learning models. Conclusion: Sophisticated integration of large number filters is able to pinpoint effective features from liver images that are sufficient to distinguish vessels from other liver tissues under a scarcity of large-volume labeled data. The study can shed light on medical image segmentation, especially for those without sufficient data.
更多查看译文
关键词
large filters,segmentation,small data,liver
AI 理解论文
溯源树
样例
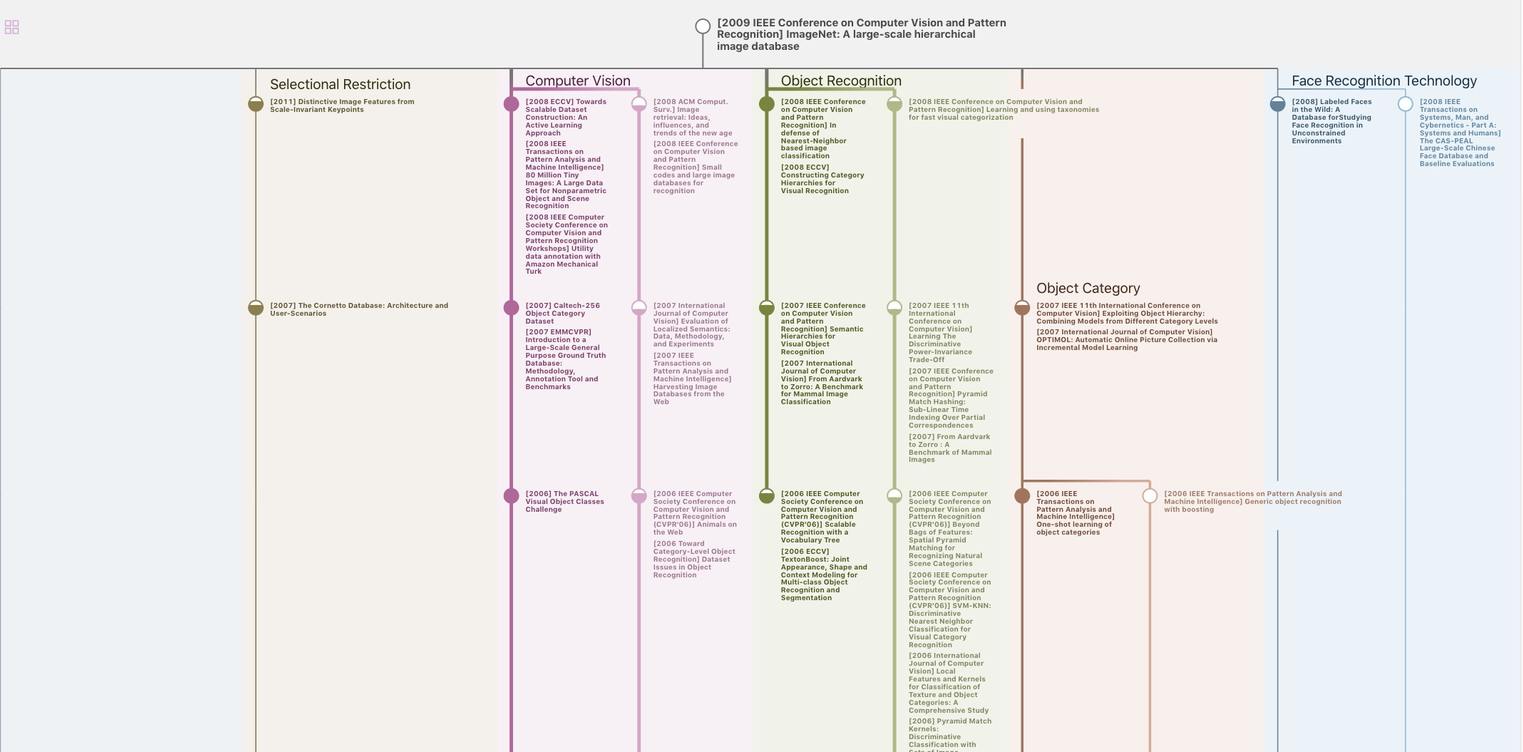
生成溯源树,研究论文发展脉络
Chat Paper
正在生成论文摘要