Abstract 6037: Identification of collateral lethal targets in cancers using integrated machine learning and flux analysis platform for personalized metabolic therapy
Cancer Research(2023)
摘要
Abstract Chromosomal alterations that occur frequently in cancers confer selective advantages for tumor progression by deleting tumor-suppressing genes or amplifying oncogenic drivers. However, the collateral effect of these, i.e., deletion of essential genes or upregulation of metabolic regulators, exposes cancers to biological pressures by suppressing essential metabolic pathways. Cancer cells rely on paralogous metabolic pathways to compensate for this loss of function, which in turn opens the door to exploit metabolic vulnerabilities arising as a direct consequence of chromosomal alterations. These pathways are collateral lethal (CL) targets and offer a unique precision medicine approach for metabolic therapeutics. We have developed an integrated machine learning and systems biology platform, collateral lethal gene identification via metabolic fluxes (CLIM) to identify metabolic targets across multiple cancers. Results: In ovarian cancers with 19p13.3 deletion, a loss of Complex III subunit-encoding gene UQCR11 occurs, leading to suppressed electron transport chain (ETC) activity. CLIM predicted that MTHFD2 acts noncanonically to oxidize NADH to NAD+ to compensate for reduced NAD+ recycling via the ETC. We demonstrate selective death of ETC deficient ovarian cancers by targeting MTHFD2 and provide mechanistic validation of oxidative MTHFD2 flux. We observed significant shrinkage of ovarian tumors with ETC deficiency upon MTHFD2 knockdown in mouse models. In triple negative breast cancers (TNBC), we discovered a chromosomal amplification leading to reprogramming of the serine biosynthesis pathway. CLIM predicts a target in the tryptophan metabolism. Methods: Patients are stratified using machine learning to identify tumors with distinct chromosomal alterations. Multiobjective metabolic flux analysis is employed to predict CL pathways in curated genome-scale metabolic models. Validation of metabolic rewiring predicted by CLIM were examined using 3-2H-glucose, 4-2H-glucose and 2,3,3-2H-serine to probe the one carbon metabolism. Oxidative MTHFD2 flux was quantified using innovative combinatorial tracer study with 2H-formate and 4-2H-glucose. We utilized gain- and loss-of-function assays in multiple cell-lines to show utility of the predicted CL targets in TNBCs with chromosomal amplifications. Conclusion: Our integrated platform successfully identifies CL gene pairs to decipher novel metabolic targets specific to chromosomal alterations across many cancer types. Importantly, targets identified by CLIM are based on quantitative flux analysis. This approach reveals functional connection between CL pairs, thereby providing a mechanistic understanding of emerging lethality. This is critical in developing therapeutic interventions that selectively target cancer cells, with minimal off-target or side effects. Citation Format: Abhinav Achreja, Jin Heon Jeon, Mark Slayton, Tao Yu, Olamide Animasahun, Minal Nenwani, Fulei Wuchu, Anjali Mittal, Xiongbin Lu, Sofia D. Merajver, Deepak Nagrath. Identification of collateral lethal targets in cancers using integrated machine learning and flux analysis platform for personalized metabolic therapy. [abstract]. In: Proceedings of the American Association for Cancer Research Annual Meeting 2023; Part 1 (Regular and Invited Abstracts); 2023 Apr 14-19; Orlando, FL. Philadelphia (PA): AACR; Cancer Res 2023;83(7_Suppl):Abstract nr 6037.
更多查看译文
关键词
machine learning,flux analysis platform,cancers,collateral lethal targets
AI 理解论文
溯源树
样例
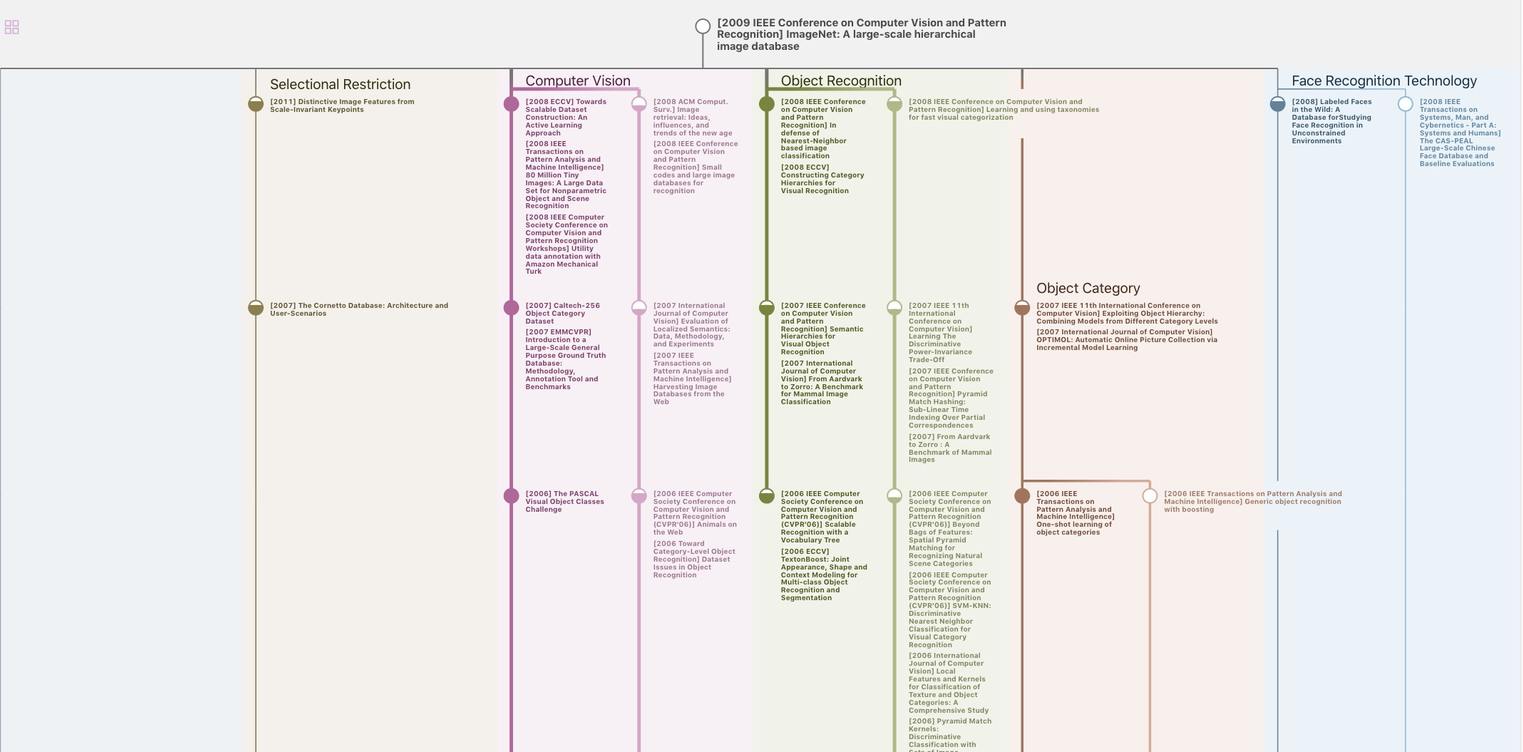
生成溯源树,研究论文发展脉络
Chat Paper
正在生成论文摘要