Abstract 5422: Machine learning models identify key histological features of renal cell carcinoma subtypes
Cancer Research(2023)
摘要
Abstract Renal cell carcinoma (RCC) is a heterogeneous disease with 16 different subtypes identified and diagnosed by assessment of tumor histology, and specific molecular and genetic markers. Three major subtypes - clear cell, papillary, and chromophobe carcinoma - have different prognoses and treatment regimens. Treatment response has been associated with mutations that may affect the tumor microenvironment (TME). Here, machine learning (ML) models quantified histologic features of the TME directly from RCC hematoxylin and eosin (H&E)-stained whole slide images (WSI). The potential for model outputs to predict clinically-relevant biomarkers was investigated. Machine learning models based on convolutional neural networks were trained using RCC and non-RCC kidney WSI of biopsies and resections from the cancer genome atlas (TCGA), proprietary, and commercial sources (tissue model N=3208; cell model N=789; vessel model N=839) to classify and quantify histologic features of the TME including cells, tissues, and blood vessels. Thousands of human interpretable features (HIFs) were extracted from model predictions that precisely describe the TME across each WSI (e.g., cell density within a tissue region). Associations between HIFs and PBRM1 loss of function (LOF) mutations and VEGFA mRNAseq expression in clear cell RCC were determined using univariate logistic regressions and Spearman correlations, respectively. False discovery rate in multiple hypothesis testing was controlled using an Empirical Brown’s Method and the Benjamini-Hochberg procedure. ML-models generated 3390 HIFs from 692 TCGA RCC WSI. After quality control to remove features with missing values, outlier slides and de-duplication, 237 HIFs (657 WSI) remained. Major RCC subtypes could be extracted directly from these HIFs using hierarchical clustering (p<10-6, chi-squared test). Individual RCC subtypes were distinguished by features describing immune cells and vascularization. A mixed subcluster enriched for higher stage (p<10-6, chi-squared test) papillary and clear cell RCC tumors was associated with increased prevalence of sarcomatoid regions and immune cells. In clear cell RCC, significant associations were found between HIFs describing a spatially specific increase in lymphocytes in the cancer epithelium (FDR-corrected p=0.004) and decrease in macrophages (FDR-corrected p=0.004) in the entire tumor area and PBRM1MUT, a mutation associated with response to immunotherapy; VEGFA expression, predictive of angiogenesis, positively associated with an increased abundance of lymphocytes near erythrocytes (Spearman r=0.37). ML model quantified RCC TME histology allowed identification of spatially specific differences that correlate with histological subtypes, mutations, and vascularization. Complementary ML-based TME assessment and genomic analyses may be used, after further validation, to explore novel biomarkers. Citation Format: Samuel Vilchez, Isaac Finberg, Miles Markey, Shima Nofallah, Kathleen Sucpito, Fedaa Najdawi, Geetika Singh, Ben Trotter, Victoria Mountain, Jake Conway, Robert Egger, Chintan Parmar, Ilan Wapinski, Stephanie Hennek, Jonathan Glickman. Machine learning models identify key histological features of renal cell carcinoma subtypes. [abstract]. In: Proceedings of the American Association for Cancer Research Annual Meeting 2023; Part 1 (Regular and Invited Abstracts); 2023 Apr 14-19; Orlando, FL. Philadelphia (PA): AACR; Cancer Res 2023;83(7_Suppl):Abstract nr 5422.
更多查看译文
关键词
machine learning models,machine learning,renal,key histological features
AI 理解论文
溯源树
样例
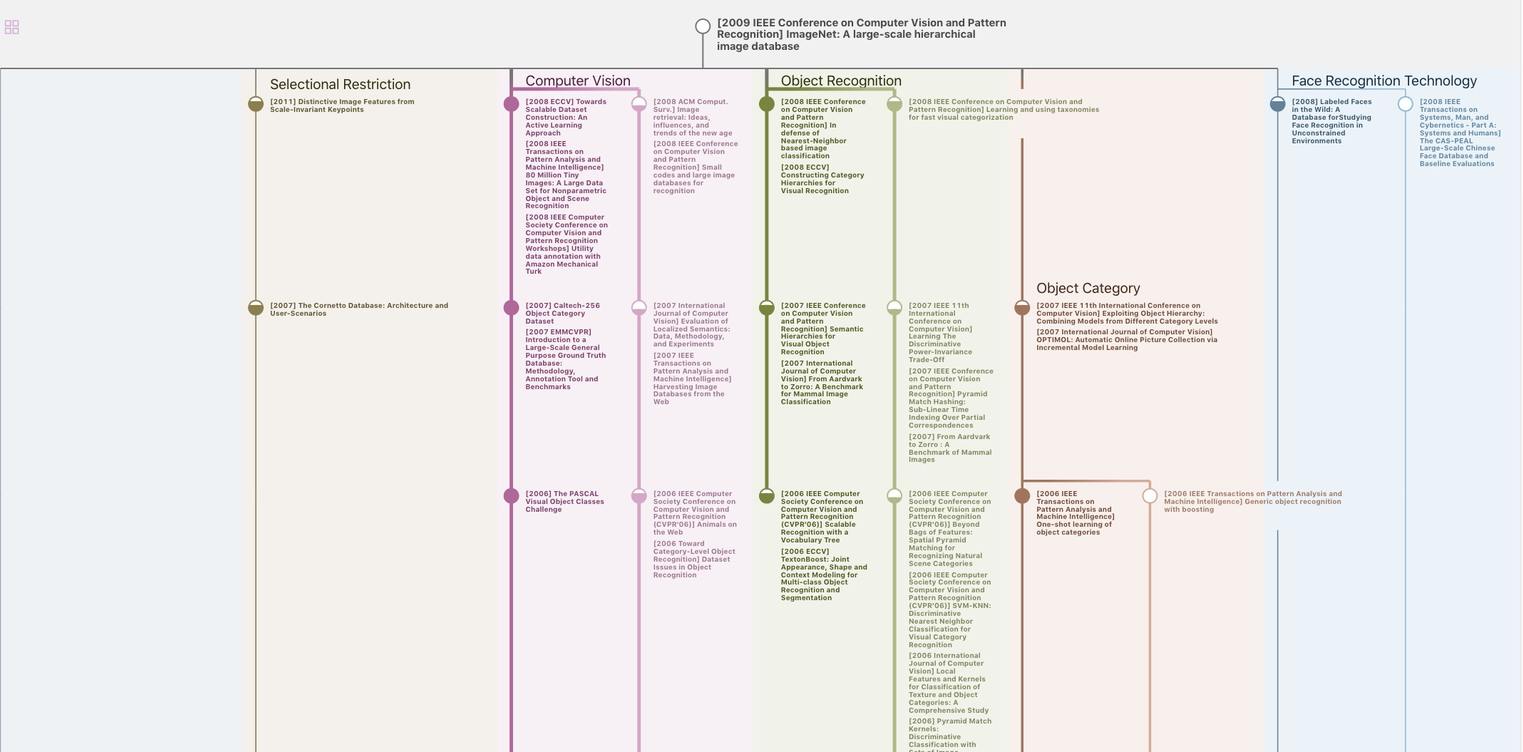
生成溯源树,研究论文发展脉络
Chat Paper
正在生成论文摘要