Understanding of Convolutional Neural Network (CNN): A Review
International Journal of Robotics and Control Systems(2023)
摘要
The application of deep learning technology has increased rapidly in recent years. Technologies in deep learning increasingly emulate natural human abilities, such as knowledge learning, problem-solving, and decision-making. In general, deep learning can carry out self-training without repetitive programming by humans. Convolutional neural networks (CNNs) are deep learning algorithms commonly used in wide applications. CNN is often used for image classification, segmentation, object detection, video processing, natural language processing, and speech recognition. CNN has four layers: convolution layer, pooling layer, fully connected layer, and non-linear layer. The convolutional layer uses kernel filters to calculate the convolution of the input image by extracting the fundamental features. The pooling layer combines two successive convolutional layers. The third layer is the fully connected layer, commonly called the convolutional output layer. The activation function defines the output of a neural network, such as 'yes' or 'no'. The most common and popular CNN activation functions are Sigmoid, Tanh, ReLU, Leaky ReLU, Noisy ReLU, and Parametric Linear Units. The organization and function of the visual cortex greatly influence CNN architecture because it is designed to resemble the neuronal connections in the human brain. Some of the popular CNN architectures are LeNet, AlexNet and VGGNet.
更多查看译文
关键词
convolutional neural network,cnn,understanding
AI 理解论文
溯源树
样例
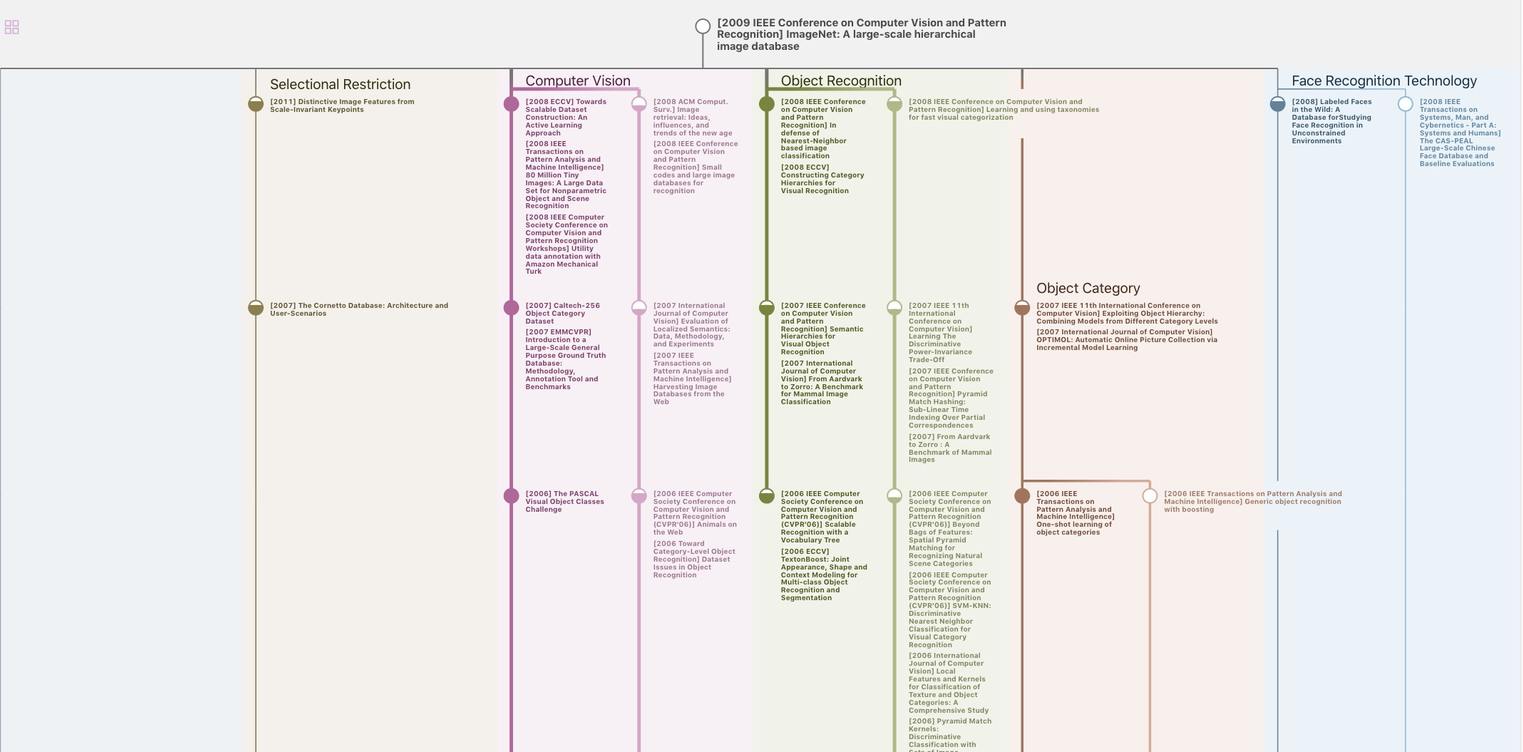
生成溯源树,研究论文发展脉络
Chat Paper
正在生成论文摘要