Deep learning-based organ classification and transit time estimation for wireless capsule endoscopy
Research Square (Research Square)(2023)
摘要
Abstract Background Deep learning-based technologies are widely used in gastroenterology, especially for the lesion detections during endoscopic examination. However, there have been limited studies on the localization of wireless capsule endoscopy (WCE). We propose an algorithm for classifying organs and predicting gastric and small bowel transit time during WCE examination. Methods We collected WCE data from 126 patients, using PillCam™ SB3 (Medtronic, Minneapolis, MN, USA) at two university-affiliated hospitals. The dataset consisted of 2,395,932 images. We developed a deep learning model that accurately classified organs (i.e., stomach, small bowel, and colon) from 44 train and 10 validation cases. Then we applied calibration using a Gaussian filter to enhance the accuracy of detecting organ boundaries. Lastly, we estimated the transit time of the capsule in the gastric and small bowel regions using a combination of a convolutional neural network and a long short-term memory designed to be aware of the sequence information of continuous videos. We evaluated our model’s performance with WCE videos from 72 patients. Results Our model demonstrated an accuracy, sensitivity, and specificity of over 95% for classifying each organ (stomach, small intestine, and colon) and 97.1% of overall accuracy and F1-score. In the estimation of gastric and small bowel transit time, the mean time difference between the ground truth and model prediction was 4.3 ± 9.7 minutes and 24.7 ± 33.8 minutes, respectively. The model’s gastric transit time predictions were within 15 minutes for 95.8% of the test data set (69/72). Conclusions Our proposed algorithm shows promising results in classifying the organs and estimating the gastric transit time for WCE, which has significant clinical implications.
更多查看译文
关键词
organ classification,endoscopy,transit time estimation,learning-based
AI 理解论文
溯源树
样例
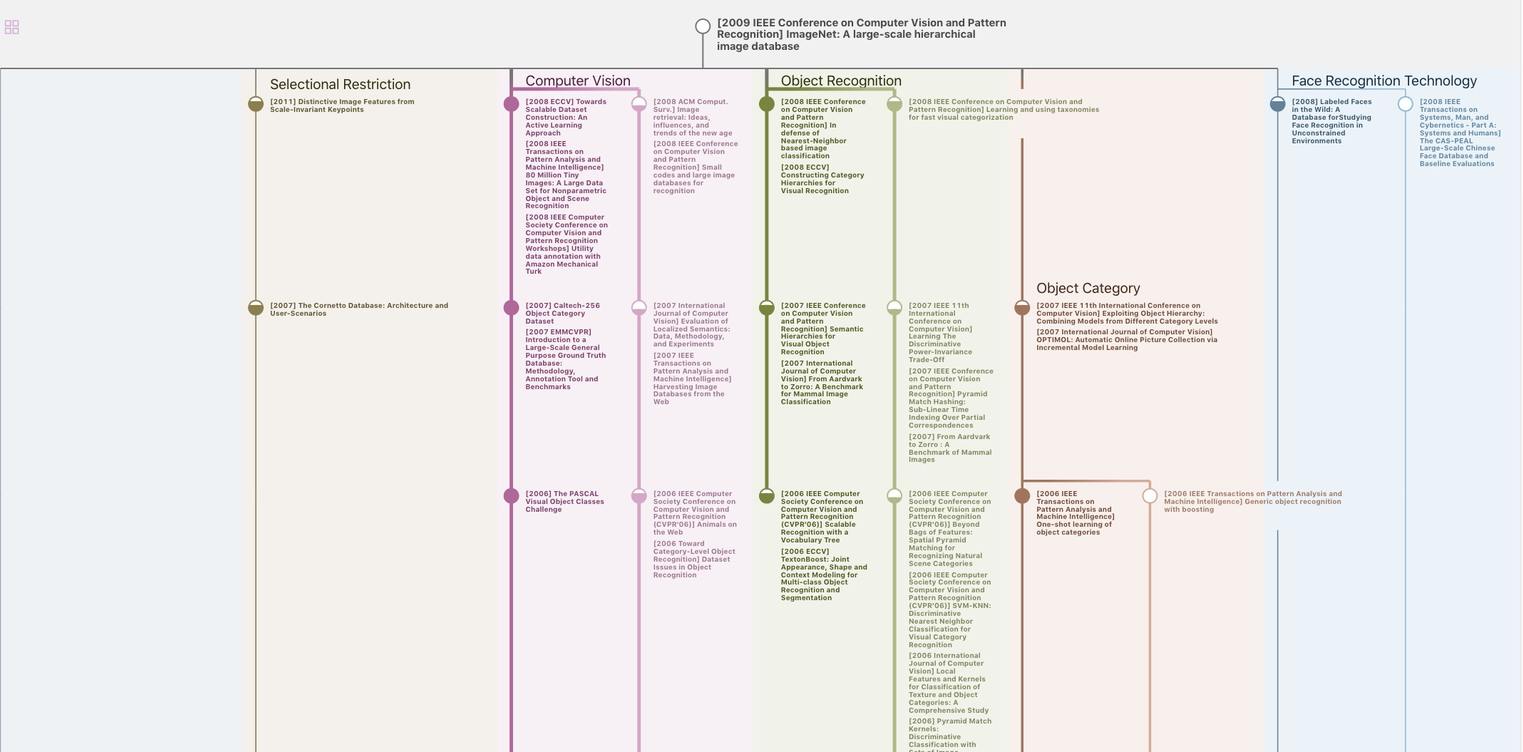
生成溯源树,研究论文发展脉络
Chat Paper
正在生成论文摘要