Efficient parameter estimation for missing data when many features are fully observed
Research Square (Research Square)(2023)
摘要
Abstract Parameters (mean and covariance matrix) estimation is often a problem of interest since it provides information about the location and variation of the data and correlation between features and can be used for hypothesis testing, principle component analysis, etc. However, it is also common that values in some features of a dataset are missing. A popular way to deal with this problem is to use an Expectation-Maximization algorithm or to impute the missing values and then estimate the parameters based on imputed data. However, the first approach is a local optimization approach that may not converge under a fixed number of iterations. The second one, a two-step approach of imputation and analysis, is computationally inefficient. Therefore, we follow the recent trends of estimating the parameters directly from the data and propose PMF (Parameter estimation for Missing data in some Features) to deal with the aforementioned problems. The experiments show that our approach achieves better performance than other methods under comparison in performance and speed. Moreover, our estimates are asymptotic.
更多查看译文
关键词
efficient parameter estimation,many features
AI 理解论文
溯源树
样例
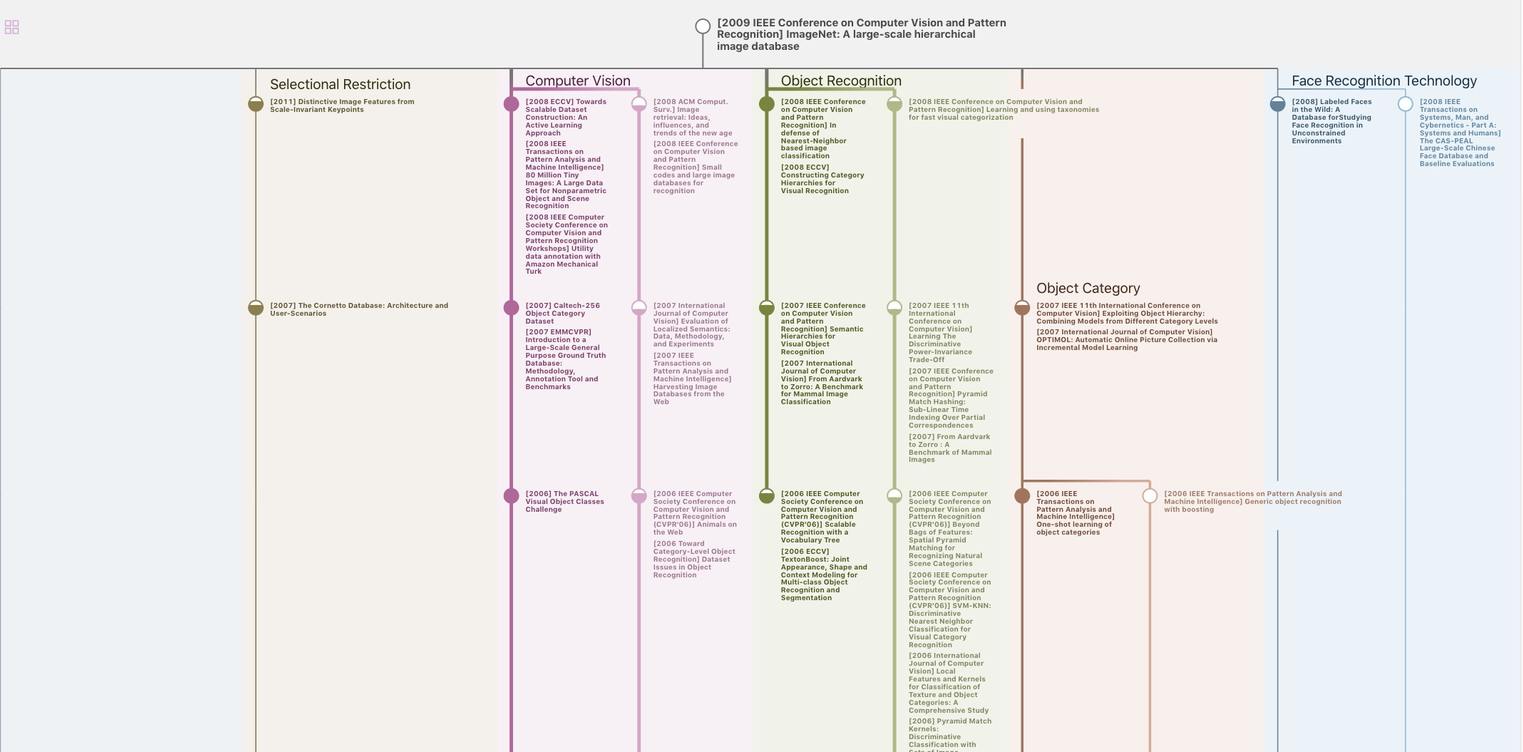
生成溯源树,研究论文发展脉络
Chat Paper
正在生成论文摘要