Deep probability learning-based release consequence estimation approach for inherently safer design of chemical plant
Methods in chemical process safety(2023)
摘要
Accidental release of hazardous material has contributed to many major accidents in chemical plant. One of the approaches to eliminate this risk is by using an inherently safe design concept. Consequence models play the significant roles in providing the consequence estimations for inherent safety design. However, prevalent Gaussian plume dispersion model has poor accuracy under certain complex conditions. This chapter introduces an advanced probabilistic deep learning-based release and dispersion consequence prediction approach, namely DPL_PlumePred. DPL_PlumePred could estimate the released plume size and concentration more accurately within chemical facilities. Three case studies are conducted to demonstrate the accuracy of our proposed DPL_PlumePred compared to prevalent prediction models. Application framework of proposed DPL_PlumePred for inherently safer design of chemical plant is also discussed. The results demonstrate our model could estimate more accurate released plume concentration, which supports more reliable information to eliminate root release cause and ensure inherently safer design of chemical plant.
更多查看译文
关键词
release consequence estimation approach,safer design,chemical,learning-based
AI 理解论文
溯源树
样例
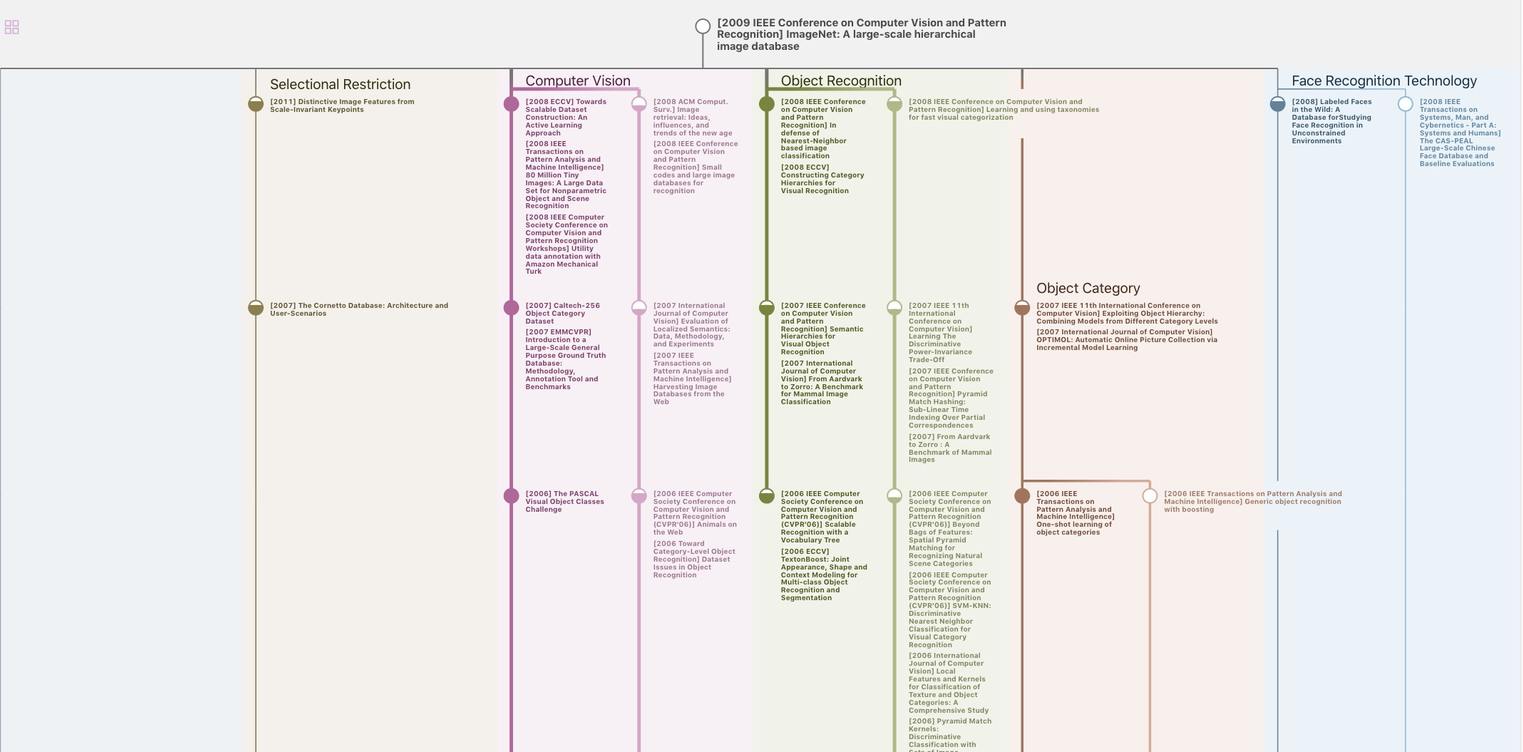
生成溯源树,研究论文发展脉络
Chat Paper
正在生成论文摘要