Traffic Flow Prediction on Graph with Node-level Curriculum Scheduler
Research Square (Research Square)(2023)
摘要
Abstract Traffic flow prediction is a critical part of the intelligent transportation system , and many schemes have been proposed for accurate flow prediction based on traffic sensors. However, existing approaches mainly capture spatial-temporal (ST) dependencies from the traffic graph and train their models using randomly ordered data. This approach ignores the fact that the modeling difficulty of each sensor/node from the traffic graph can vary significantly due to its spatial dependencies and temporal trends, leading to unreliable and unstable predictions for each traffic sensor in real-world applications. These issues motivated us to design a more effective training process for sensors with different levels of difficulty. The ST modeling difficulty stems from two factors: (I) deviations between the normal component and abnormal component in the time sequences; and (II) deviations between the learned relation and the explicit relation of a predetermined graph in the spatial dependencies. In this case, we argue that a well-designed curriculum with an easy-to-difficult order can benefit the training of ST models. This approach draws inspiration from the human learning principle that models should first learn straightforward concepts before tackling more difficult ones. To implement this approach, this paper proposes a method to measure the ST difficulty at the node level of traffic graphs from both spatial and temporal aspects. Then, it conducts a curriculum during the ST model training procedure. Specifically, 1 based on the ST difficulty score, the model training starts from a subgraph whose nodes are considered ”easy” because of their relatively consistent spatial relationships and regular temporal patterns. After that, more difficult nodes are gradually added to the subgraph and participate in the following training stages. We have conducted extensive experiments on two public traffic datasets, which show the superiority of our proposed curriculum scheduler over multiple state-of-the-art ST models.
更多查看译文
关键词
graph,curriculum,flow,node-level
AI 理解论文
溯源树
样例
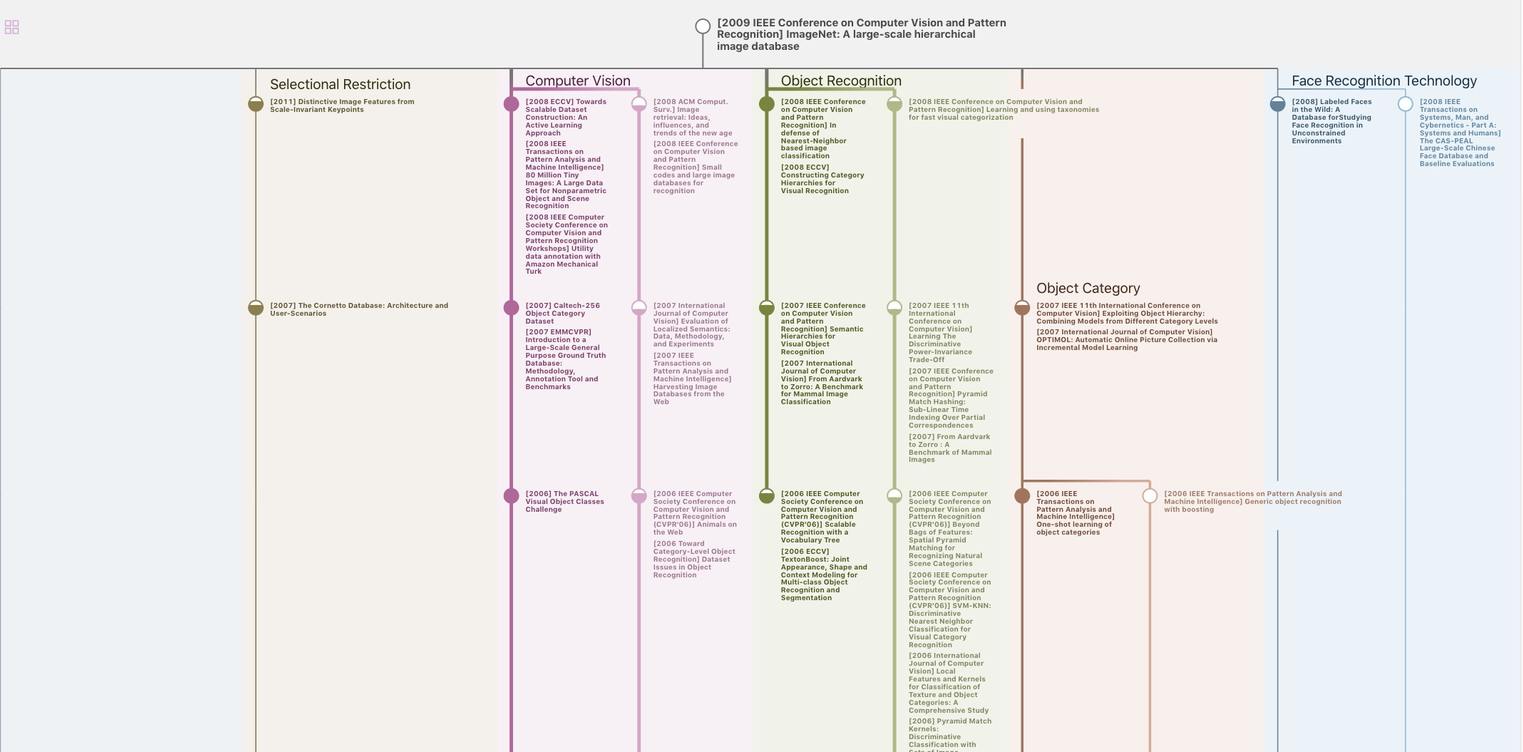
生成溯源树,研究论文发展脉络
Chat Paper
正在生成论文摘要