Deep Learning Framework for Automated Goblet Cell Density Analysis in In-Vivo Rabbit Conjunctiva
Research Square (Research Square)(2023)
摘要
Abstract Goblet cells (GCs) in the conjunctiva are specialized epithelial cells secreting mucins on the ocular surface and play important roles for ocular surface health. Because GC loss is observed in various ocular surface diseases, GC examination is important. A non-invasive GC imaging method was recently developed, and a robust analysis is needed to make GC information available. In this study, we developed a deep learning framework for GC image analysis. Dual-channel convolution was used to extract the overall texture of image and to acquire the morphological characteristics of GCs. A global channel attention module was adopted by combining attention algorithms and channel-wise pooling. The module generated an adaptive attention map through matrix multiplication with channel-wise weights and propagated information to strengthen low-level features. DCAU-Net showed 93.1% GC segmentation accuracy and 94.3% GC density estimation accuracy. Further application, both normal and ocular surface damage rabbit models revealed the spatial variations of both GC density and size and the decreases of both GC density and size in damage rabbit models during recovery after acute damage. GC image analysis results were consistent with histology. Together with the non-invasive imaging method, DCAU-Net would provide GC information for the diagnosis of ocular surface diseases.
更多查看译文
关键词
cell,deep learning,in-vivo
AI 理解论文
溯源树
样例
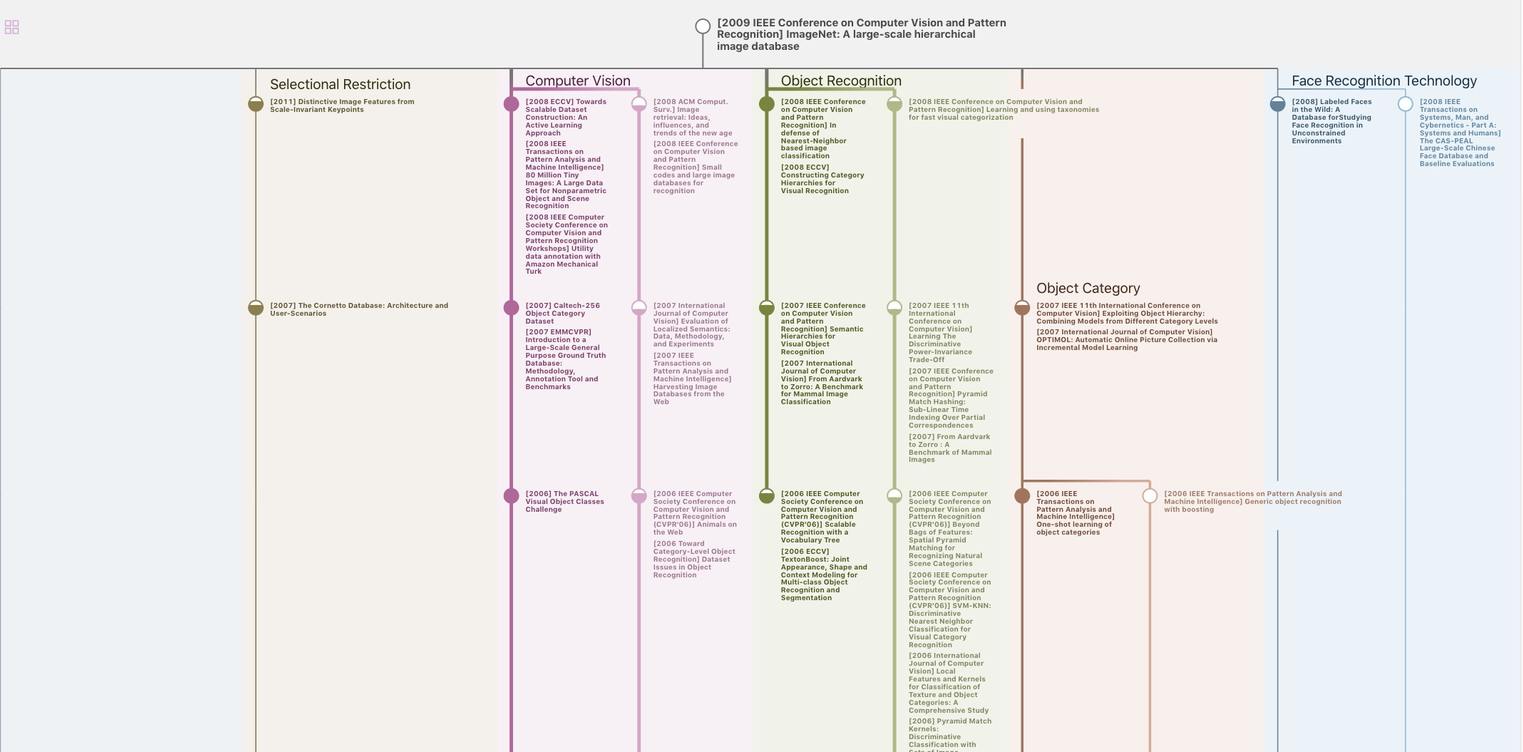
生成溯源树,研究论文发展脉络
Chat Paper
正在生成论文摘要