StomataTracker: Revealing circadian rhythms of wheat stomata with in-situ video and deep learning
COMPUTERS AND ELECTRONICS IN AGRICULTURE(2023)
摘要
Plant stomata are essential channels for gas exchange between plants and the environment. The infrared gasexchange system has greatly accelerated the studies of stomatal conductance (gs). Nevertheless, due to the lack of in-situ monitoring techniques, the behavior of stomata themselves remains poorly understood, especially in nocturnal environmental conditions. Here, a deep-learning-based stoma tracking pipeline (StomataTracker) was first proposed to continuously monitor stoma traits from unprecedentedly long-term, continuous, and nondestructive video data. Compared to the semi-automatic method (ImageJ), the open-source StomataTracker could greatly improve the extraction efficiency from 207 s to 1.47 s of stomatal traits, including stomatal area, perimeter, length, and width. The R-2 adjusted of the four stomatal traits ranged from 0.620 to 0.752. In addition, the rhythm of wheat stomata opening in a completely dark environment was first reported from long-term video data. The closed time of stoma at night was negatively correlated with stomatal traits, and the R ranged from -0.583 to -0.855. The heterogeneity of stomatal behavior also highlighted that smaller stomata have the rhythm pattern of longer closure time at night. Overall, our study provides a novel perspective for stomatal study, and it is conducive to accelerating the application of stomatal circadian rhythm in wheat breeding.
更多查看译文
关键词
Wheat,Stoma,Circadian rhythm,Deep learning,Nocturnal stomatal behaviors,In -situ monitoring
AI 理解论文
溯源树
样例
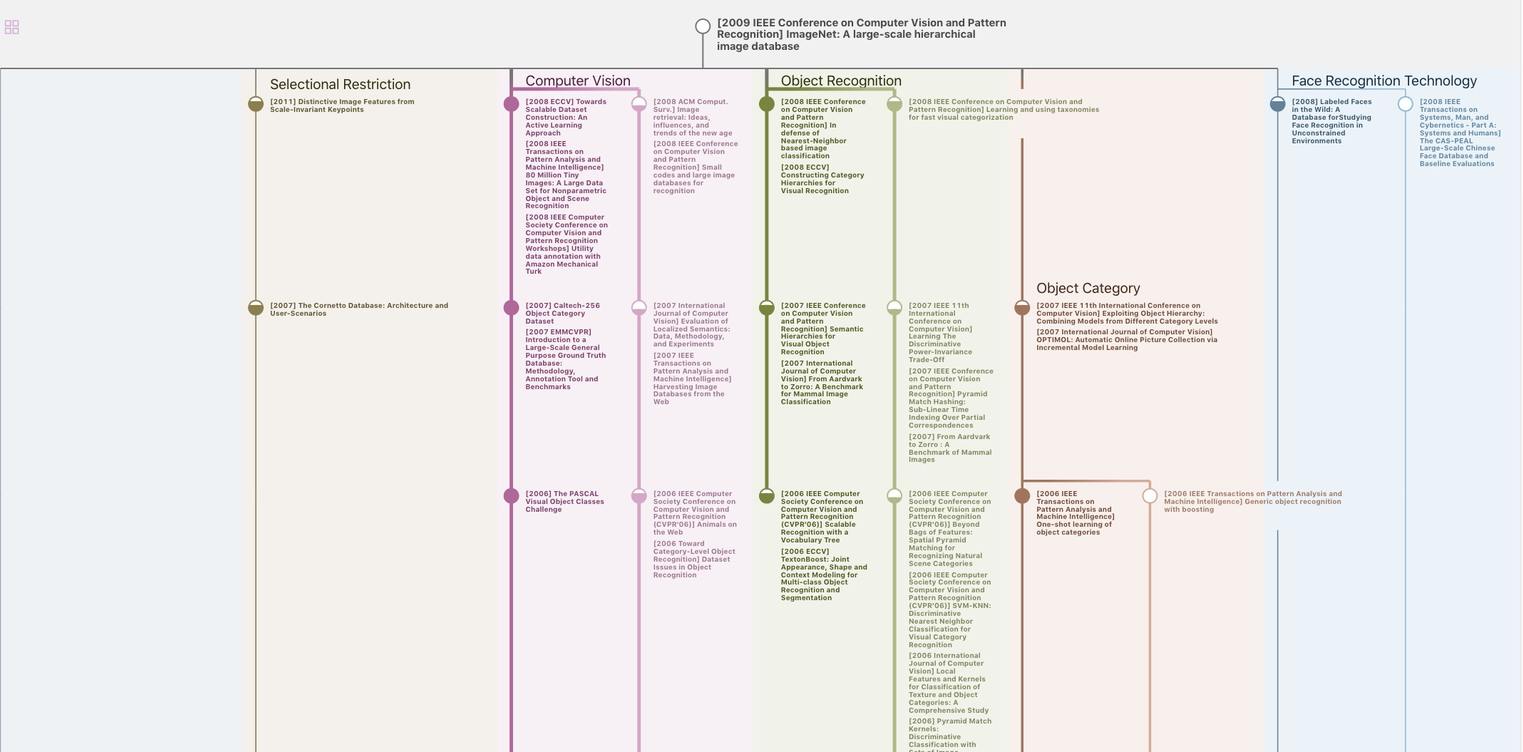
生成溯源树,研究论文发展脉络
Chat Paper
正在生成论文摘要