Low-Cost Federated Broad Learning for Privacy-Preserved Knowledge Sharing in the RIS-Aided Internet of Vehicles
Engineering(2023)
摘要
High-efficiency and low-cost knowledge sharing can improve the decision-making ability of autonomous vehicles by mining knowledge from the Internet of Vehicles (IoVs). However, it is challenging to ensure high efficiency of local data learning models while preventing privacy leakage in a high mobility environment. In order to protect data privacy and improve data learning efficiency in knowledge sharing, we propose an asynchronous federated broad learning (FBL) framework that integrates broad learning (BL) into federated learning (FL). In FBL, we design a broad fully connected model (BFCM) as a local model for training client data. To enhance the wireless channel quality for knowledge sharing and reduce the communication and computation cost of participating clients, we construct a joint resource allocation and reconfigurable intelligent surface (RIS) configuration optimization framework for FBL. The problem is decoupled into two convex subproblems. Aiming to improve the resource scheduling efficiency in FBL, a double Davidon–Fletcher–Powell (DDFP) algorithm is presented to solve the time slot allocation and RIS configuration problem. Based on the results of resource scheduling, we design a reward-allocation algorithm based on federated incentive learning (FIL) in FBL to compensate clients for their costs. The simulation results show that the proposed FBL framework achieves better performance than the comparison models in terms of efficiency, accuracy, and cost for knowledge sharing in the IoV.
更多查看译文
关键词
Knowledge sharing,Internet of Vehicles,Federated learning,Broad learning,Reconfigurable intelligent surfaces,Resource allocation
AI 理解论文
溯源树
样例
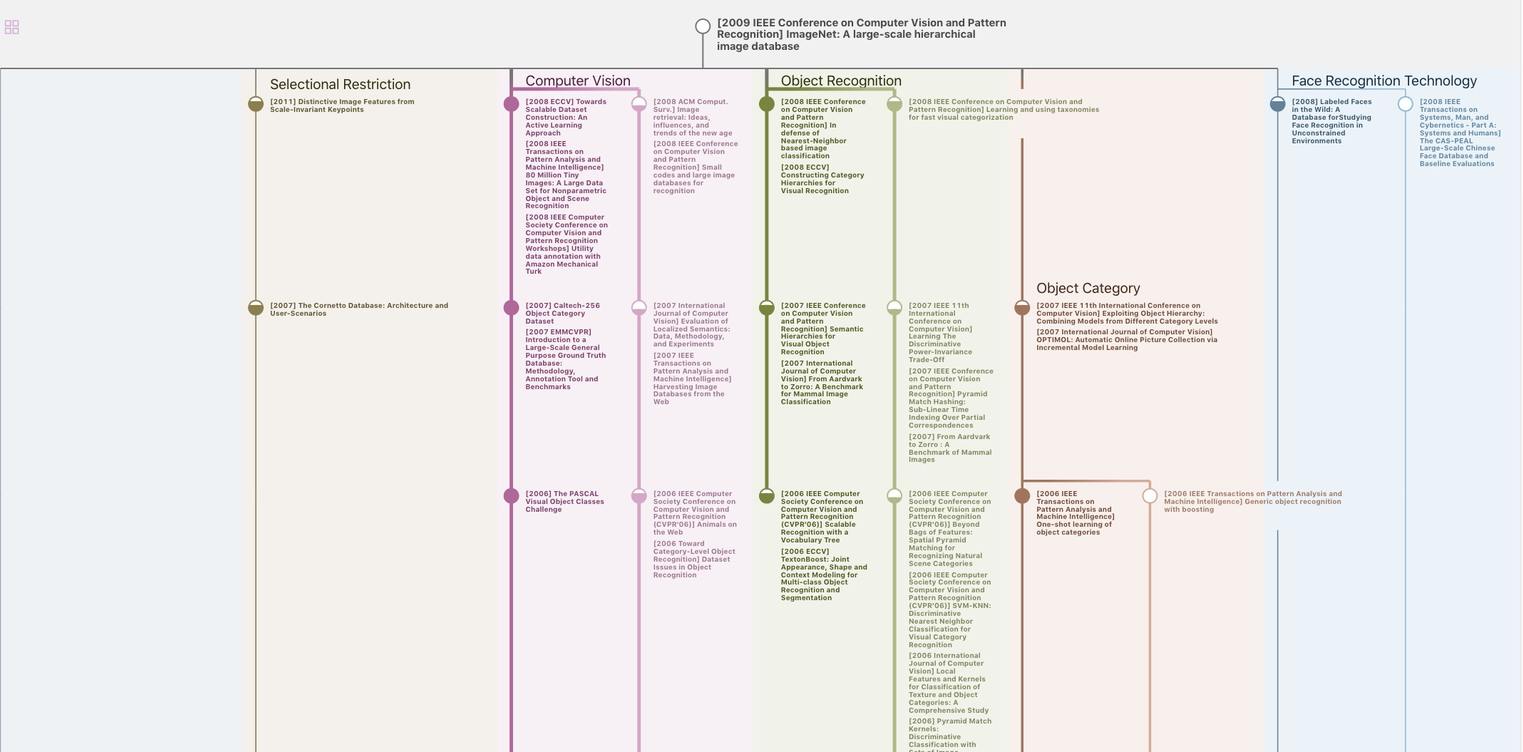
生成溯源树,研究论文发展脉络
Chat Paper
正在生成论文摘要