Towards automated, integrated and unsupervised diagnosis of rolling element bearings
Mechanical Systems and Signal Processing(2023)
摘要
In the presence of faults, vibrations of rolling element bearings show symptomatic signatures in the form of repetitive impulses. This can be seen as a non-stationary signal whose statistical properties switch intermittently between two states. Based on this observation, the paper introduces an automated diagnosis framework that integrates the successive steps of fault detection, fault identification and fault characterization. The advantage is that the complete diagnosis process is completed at once, while involving a limited number of hyperparameters. The approach relies on modeling the raw vibration signal with an explicit-duration hidden Markov model (EDHMM) and then uses the estimated model parameters for diagnosis. The detection of a fault is first achieved by means of a likelihood ratio test built on the EDHMM parameters. Posterior probabilities are then used for identifying the fault type automatically. Finally, the fault size is estimated from the duration times returned by the EDHMM. The effectiveness of the proposed method is illustrated on independent experimental datasets.
更多查看译文
关键词
Automated diagnosis,Fault detection,Fault identification,Fault characterization,Explicit duration hidden Markov model,Non-stationary vibration signal
AI 理解论文
溯源树
样例
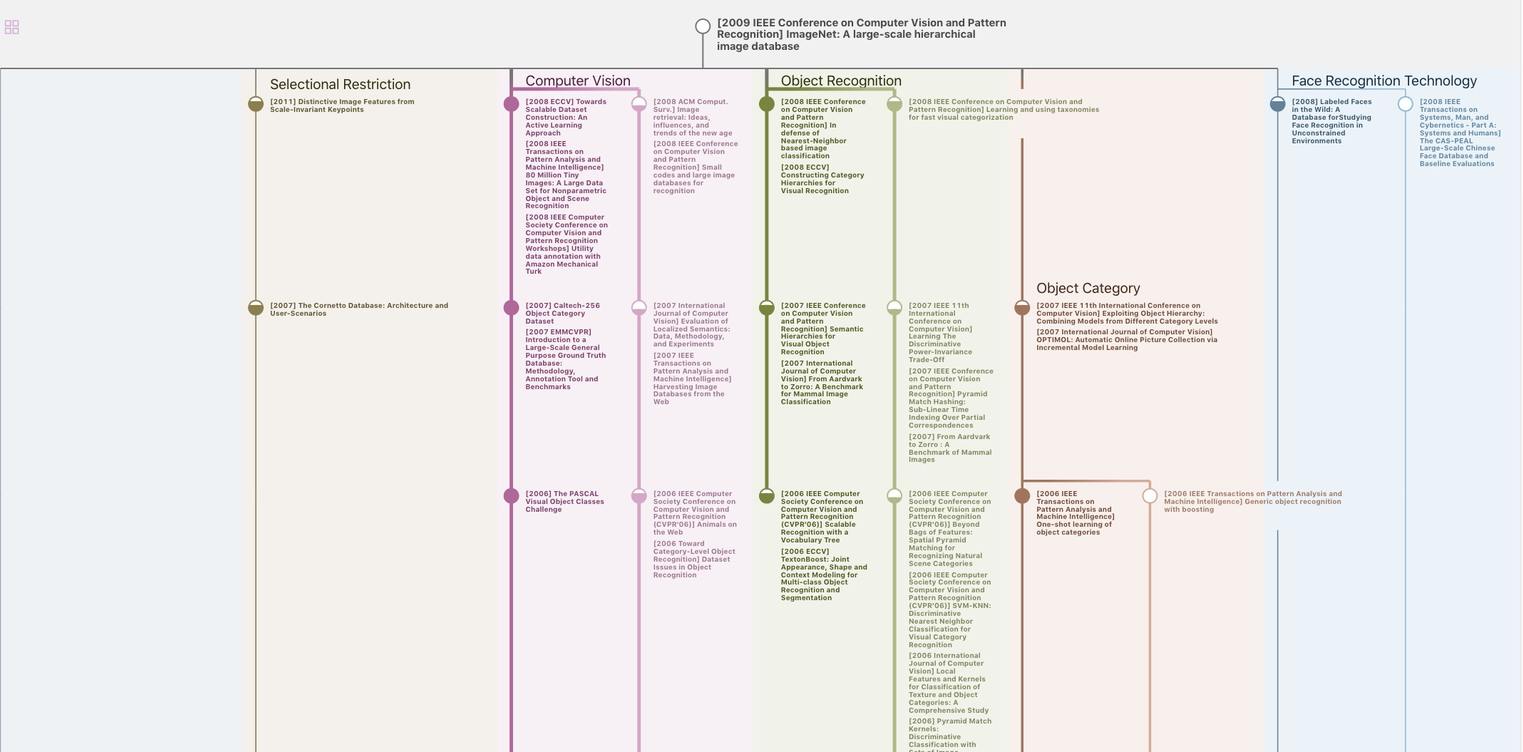
生成溯源树,研究论文发展脉络
Chat Paper
正在生成论文摘要