Predicting 24-hour Intraocular Pressure Fluctuations using Machine Learning: A Retrospective Analysis
Research Square (Research Square)(2023)
摘要
Abstract 24-hour intraocular pressure fluctuation is at a risk of glaucomatous progression. We thus aimed to develop a machine learning-based predictive model for 24-hour intraocular pressure (IOP) fluctuation. It was a retrospective analysis of electronic medical records from 978 eyes, focusing on 24-hour IOP monitoring data collected at Shanghai Fifth People's Hospital between January 2014 and July 2023. The dataset included various parameters such as blood pressure and corneal thickness. We divided the data into training (80%) and validation (20%) sets. Employing algorithms such as Support Vector Machine and Random Forest, our model predicted whether fluctuations in 24-hour intraocular pressure exceeded 8 mmHg. The Random Forest algorithm demonstrated the best performance in validation. The model achieved accuracy, specificity, precision, sensitivity, f1-score, and AUC area of 0.899, 0.965, 0.884, 0.718, 0.793, and 0.89. We eventually established a predictive model, demonstrating the potential to rectify intraocular pressure measurements using the Random Forest algorithm.
更多查看译文
关键词
machine learning,pressure
AI 理解论文
溯源树
样例
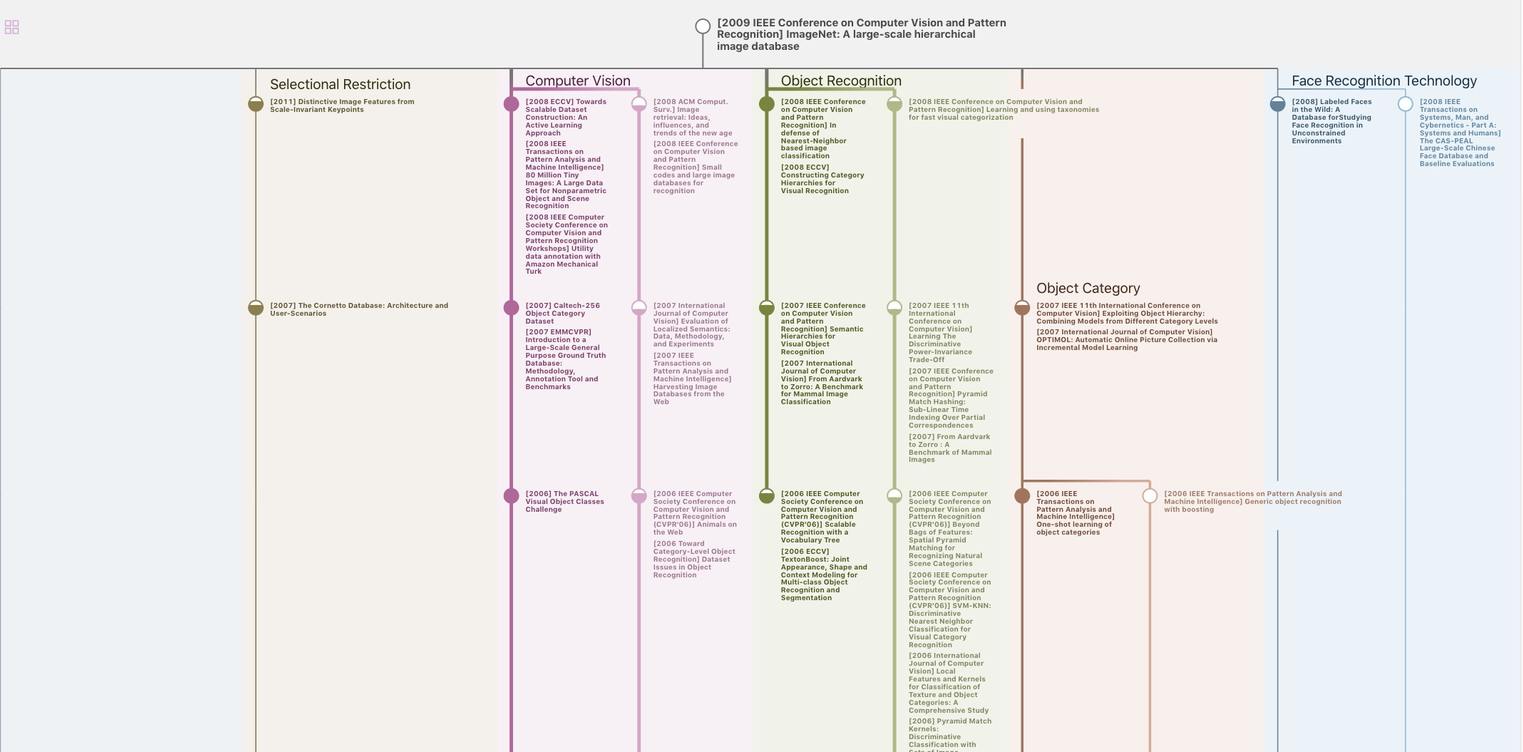
生成溯源树,研究论文发展脉络
Chat Paper
正在生成论文摘要