Instrumental variables matter: towards causal inference using deep learning
Journal of physics(2023)
摘要
Abstract Causal inference requires knowing causal connections between treatment and outcome variables, and DeepIV, a causal inference framework, is the pioneer work to predict such connections by crossing deep learning with causal inference in applying instrumental variables(IVs) to deep neural network. DeepIV has been proved to be one of the best methods in this field theoretically, but how the framework performs on real-life problems still remains unclear. This paper provides an implementation of DeepIV, and use the framework to predict causal effect from people’s educational background on their annual income. DeepIV framework allows us to take advantage of neural network to estimate causal effect by adjusting loss function. To evaluate the performace of DeepIV in solving real-life problems, our experiment is based on real datasets. The result of our experiment shows that DeepIV’s ability to predict causal effect on real data is at least as good as those of other casual inference models’ whose reliability has been verified in practice. Meanwhile, DeepIV does not have obvious shortcoming in predicting outcomes compared with other supervised learning methods.
更多查看译文
关键词
instrumental variables,causal inference,deep learning
AI 理解论文
溯源树
样例
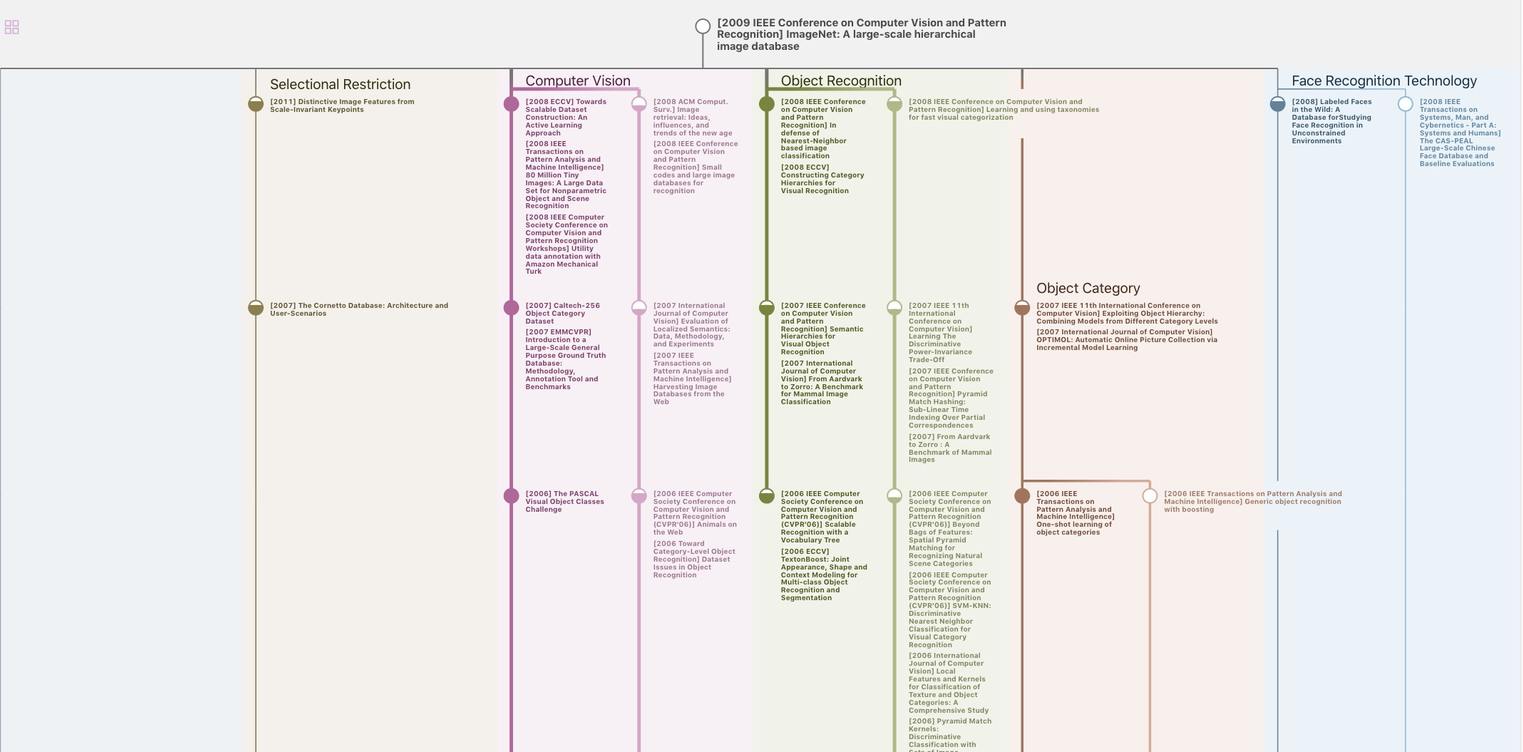
生成溯源树,研究论文发展脉络
Chat Paper
正在生成论文摘要