Graph Autoencoder-Based Anomaly Detection for Chemical Mechanical Planarization
Lecture notes in electrical engineering(2023)
摘要
The chemical mechanical planarization is a critical step in semiconductor manufacturing process, and anomaly detection can help improve yields and reduce costs. However, the CMP process is typically a batch process, and the data has the three-way structure that cannot be directly modeled. Moreover, most machine learning methods analyze samples in isolation for anomaly detection, without considering the wafer-to-wafer correlation in the process. In this paper, we propose a graph autoencoder-based framework for anomaly detection in CMP process. We first extract statistical and stable features from process variables, then generate a wafer graph by processing time and similarity, finally use graph autoencoder for anomaly detection. Experimental results on the 2016 PHM challenge dataset compared with four popular algorithms show that the proposed method achieves highest AUC, Precision@K and Recall@K.
更多查看译文
关键词
anomaly detection,graph,autoencoder-based
AI 理解论文
溯源树
样例
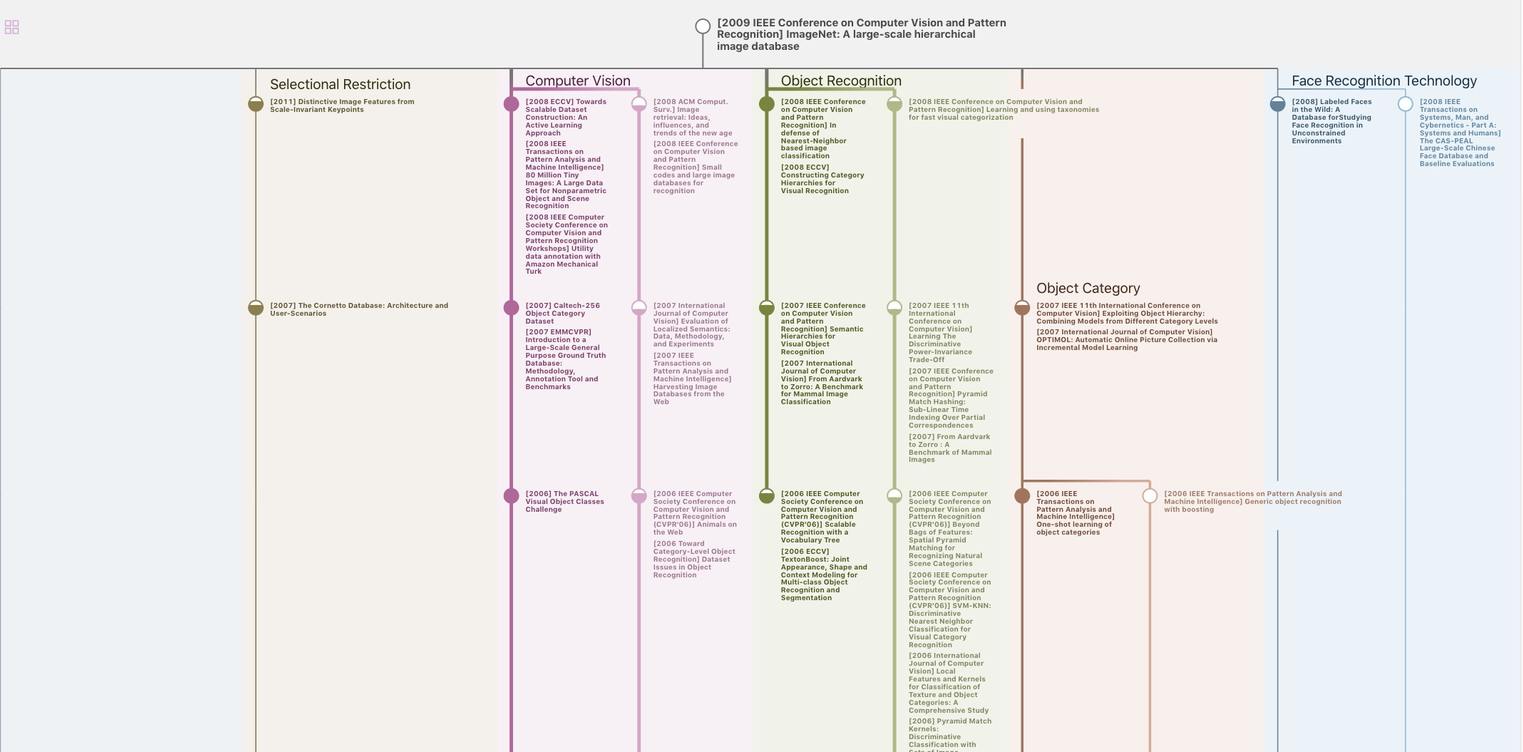
生成溯源树,研究论文发展脉络
Chat Paper
正在生成论文摘要