An Improved Siamese Capsule Networks Classification Method for Few Sample Remote Sensing Images
Lecture notes in electrical engineering(2023)
摘要
To address the problems of insufficient data and blurred details in remote sensing images under different scenarios, we propose a method to improve Siamese Capsule Networks for classification of remote sensing images with few samples. First, a pre-trained convolutional neural network is used for feature extraction and display of the input data. Second, a residual network block is added to the convolutional neural network to improve the depth and robustness of the block. Then, an attention mechanism is added to each residual block to improve the feature extraction capability of the model on the input data. Next, the output of each residual block is fed into the capsule neural network to learn the spatial structure and static information of the input data. The capsule neural network can be used for feature extraction and representation of the input data through capsule layers and dynamic routing algorithms to improve the representation capability of the model. Finally, the outputs of the two capsule neural networks are input into the twin neural network, and the similarity measure of the two capsule vectors is performed by the loss function to determine whether the two remote sensing images belong to the same kind. The experimental results show that the model can achieve 66 and 83% detection rates at 1 shot and 5 shot under the condition of few samples, and the comprehensive performance of the method is better than the existing methods.
更多查看译文
关键词
remote sensing,classification,networks
AI 理解论文
溯源树
样例
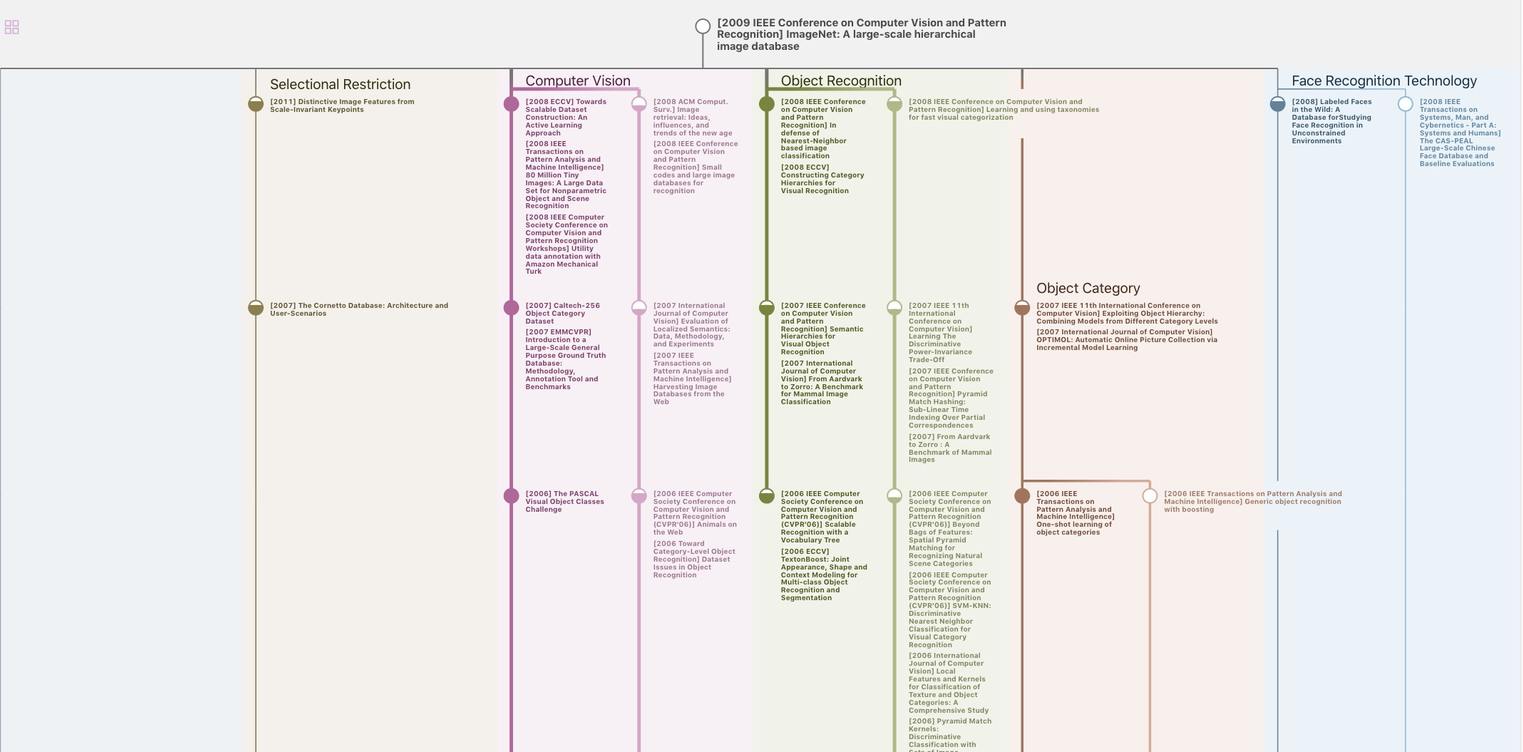
生成溯源树,研究论文发展脉络
Chat Paper
正在生成论文摘要