Deep-Learning-Based Multi-Timestamp Multi-Location PM Prediction: Verification by Using a Mobile Monitoring System With an IoT Framework Deployed in the Urban Zone of a Metropolitan Area.
IEEE Internet of Things Journal(2024)
摘要
The issue of air pollution in urban areas is gaining attention due to the rise of environmental and health concerns, especially for the particulate matter 2.5 (PM2.5), which poses the greatest health risk to humans. Accurate air quality prediction data allows government officials and the public to take preventive measures in advance. Recently, many air quality prediction studies have used machine learning techniques to identify patterns and rules in air quality data. However, these studies generally adopted under-represented background levels, and the prediction intervals were often in hours, which may not be suitable for residents who needed accurate air quality forecasts. Therefore, this study proposes a deep learning-based multi-timestamp multi-location PM2.5 prediction system built on two types of recurrent neural network models: long short-term memory (LSTM) and gated recurrent unit (GRU). Airbox data for the Taipei metropolitan area serves as the main source of training data to develop a forecasting model that can predict changes of PM2.5 levels within the next six to thirty minutes in different locations. The prediction results are verified by comparing them with the PM2.5 measuring results from an internet of things (IoT)-based on-vehicle monitoring system, which enables real-time data sensing and collection, and wireless transmission. The error and accuracy are 0.922 μ/m3 and 100% for the LSTM-based prediction model, and 0.940 μ/m3 and 95.7% for the GRU-based prediction model, respectively. These results can be sent out as warning messages to elderly and asthmatic patients, or serve as important information for route recommendations and policy formulation.
更多查看译文
关键词
Airbox,PM2.5 prediction,Long short-term memory,Gated recurrent unit,Internet of things framework
AI 理解论文
溯源树
样例
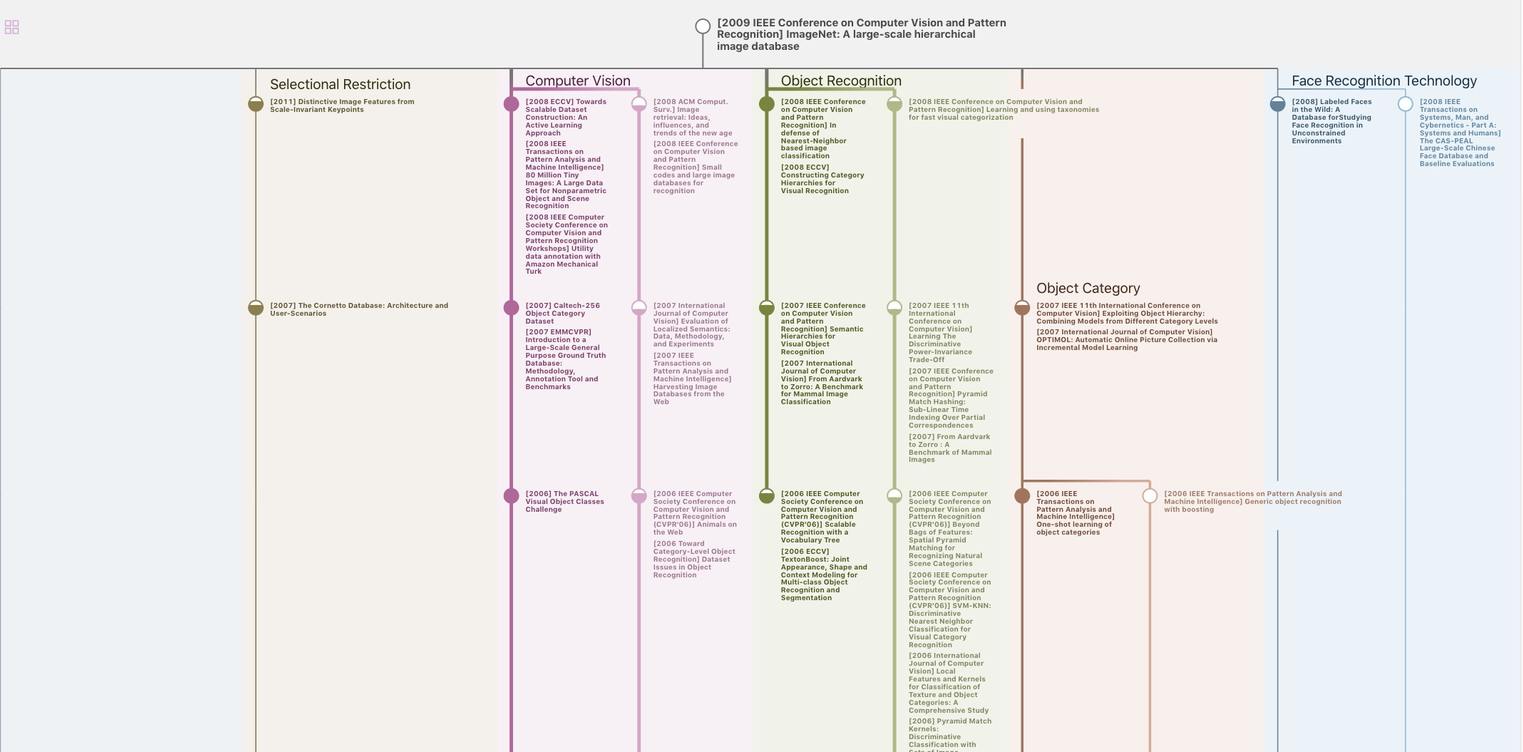
生成溯源树,研究论文发展脉络
Chat Paper
正在生成论文摘要