Progressive Fourier Adversarial Domain Adaptation for Object Classification and Retrieval
IEEE TRANSACTIONS ON MULTIMEDIA(2024)
摘要
Domain adaptation has been extensively explored as a means of transferring knowledge from the labeled source domain to the unlabeled target domain with disparate data distributions. However, the absence of target annotations and significant domain discrepancies pose a great challenge to transfer knowledge directly from source domain to target domain. To address this challenge, we propose a Progressive Fourier Adversarial Domain Adaptation (PFADA) framework, an effective and versatile framework which can generalize across multiple domain adaptation tasks. Firstly, we propose a Fourier-based style transfer strategy to generate a Fourier intermediate domain that incorporates source images with target domain-specific styles, while preserving the domain-invariant representations of the source data. Secondly, we introduce a progressive adversarial domain adaptation approach that utilizes the Fourier intermediate domain to facilitate the learning of domain-invariant representations. Finally, we present cross-domain semantic alignment and discriminative enhancement approach, which effectively guides the learning of discriminative cross-domain representations utilizing labeled source and intermediate domain data. Extensive experimental evaluations consistently validate the superior performance of the proposed method across diverse visual tasks, encompassing multiple domain adaptive image classification and retrieval scenarios.
更多查看译文
关键词
Domain adaptation,metric learning,domain adaptive image classification,cross-domain 3D model retrieval
AI 理解论文
溯源树
样例
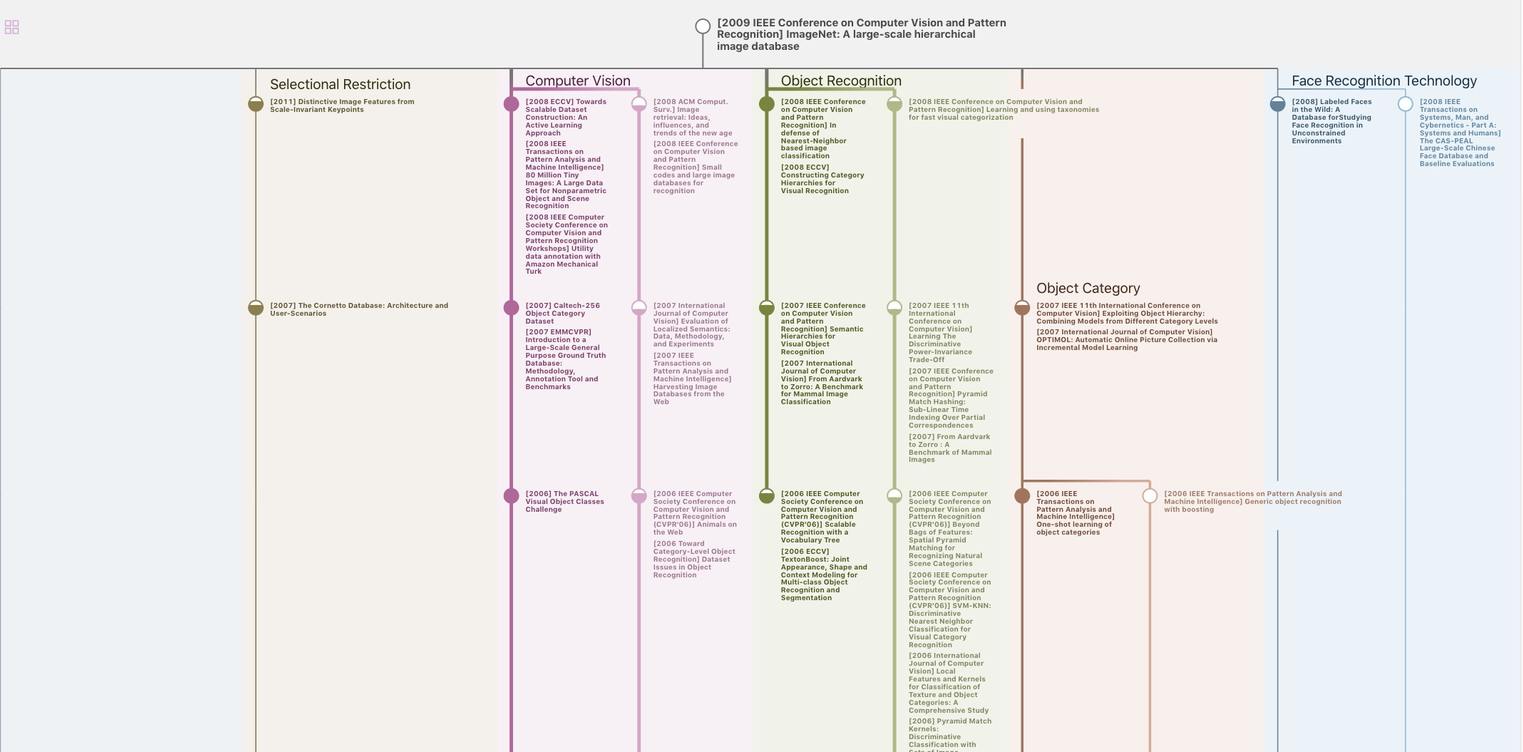
生成溯源树,研究论文发展脉络
Chat Paper
正在生成论文摘要