Automatic Multi-Camera Calibration and Refinement Method in Road Scene for Self-Driving Car.
IEEE Trans. Intell. Veh.(2024)
摘要
In autonomous driving applications, the calibration accuracy of the camera can directly affect the accuracy of perception or depth estimation. For online calibration of surround-view cameras, traditional feature extraction-based methods will suffer from strong distortion when the initial extrinsic parameters error is large, making these methods less robust and inaccurate. More existing methods use the sparse direct method to calibrate multi-cameras, which can ensure both accuracy and real-time performance and is theoretically achievable. However, this method requires a better initial value, and the initial estimate with a large error is often stuck in a local optimum. To this end, we introduce a robust automatic multi-cameras (pinhole or fisheye cameras) calibration and refinement method in the road scene. We utilize the deviable coarse-to-fine random-search strategy, and it can solve large disturbances of initial extrinsic parameters, which can make up for falling into local optimal domainin nonlinear optimization methods. In the photometric optimization stage, we add the weight of image adaptive binarization to solve the error caused by different camera exposure problems. To address the impact of non-roads on optimization, we use a road segmentation module instead of artificially specified fixed optimization regions. In the end, quantitative and qualitative experiments are conducted in actual and simulated environments, and the result shows the proposed method can achieve accuracy and robustness performance. The open-source code is available at
https://github.com/OpenCalib/SurroundCameraCalib
.
更多查看译文
关键词
Autonomous Vehicles,multi-sensor data fusion,computer vision
AI 理解论文
溯源树
样例
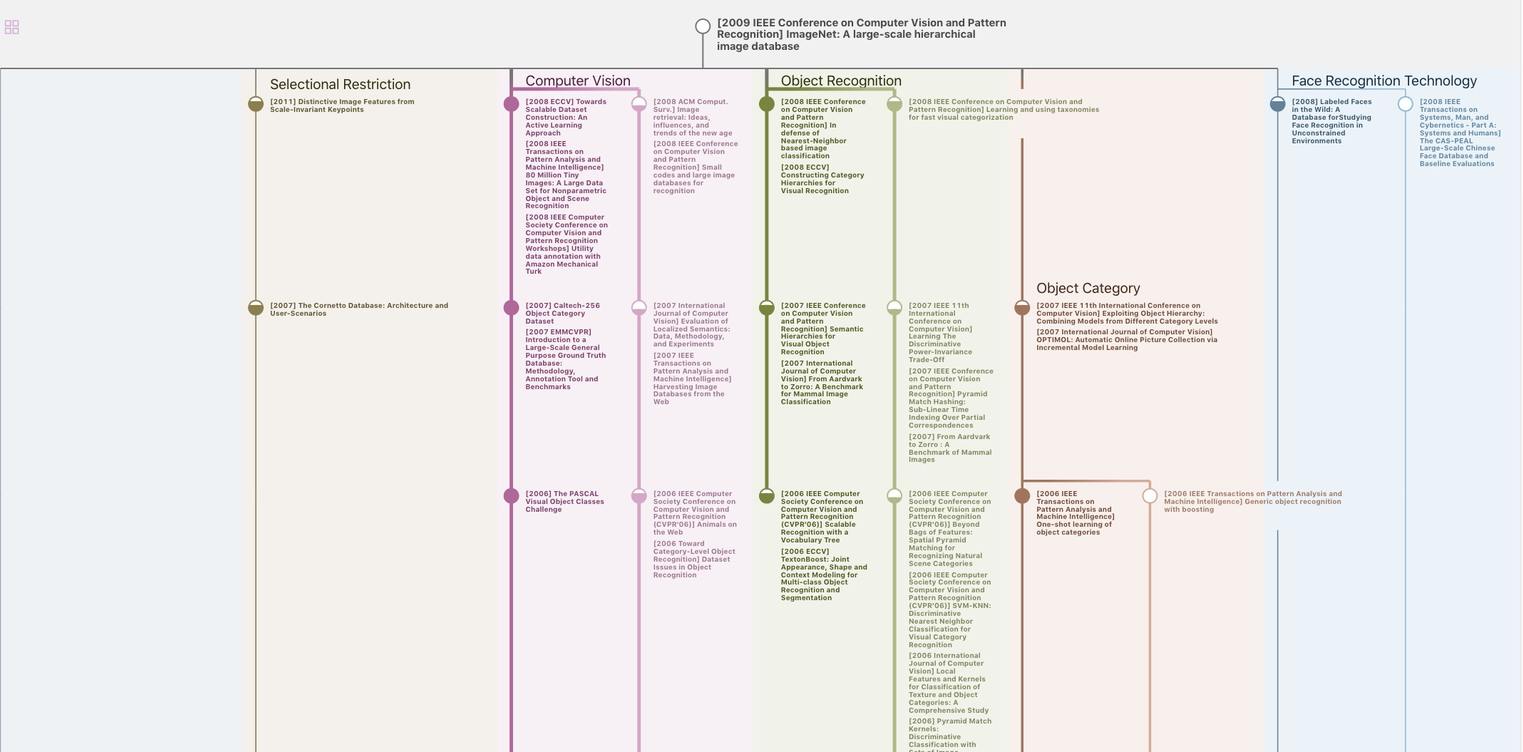
生成溯源树,研究论文发展脉络
Chat Paper
正在生成论文摘要