Predicting physiological traits of rice from hyperspectral data under CO2 and drought treatments
Authorea (Authorea)(2023)
摘要
Using hyperspectral technology for high-throughput plant phenotyping is a potentially useful method in crop sciences. To examine its effectiveness, we collected leaf-level hyperspectral and ground-reference data from rice plants grown in controlled-environments under drought and CO treatments at Ag Alumni Seed Phenotyping Facility at Purdue University. By applying RReliefF, we found that short-wave infrared region (SWIR) was important for leaf water potential (LWP), near-infrared region was linked with specific leaf area (SLA) and both red-edge and SWIR regions were related to gas exchange traits (net assimilation [A], stomatal conductance to water vapor [gsw] and transpiration [E]). Based on those results, we found that LWP and SLA were moderately predictable and gas exchange traits were predictable (R ≥ 0.60 and root mean squared error of prediction for A, gsw and Ewere 7.706 μ molms, 0.282 molms, and 3.906 mmolms in validation datasets, respectively) by using partial least squares regression. Furthermore, treatment effect on A from cross-validation predictions agreed with ground-reference data. In contrast, photosynthetic parameters (V and J) could not be estimated from hyperspectral data. Hyperspectral data can provide potential insights about plant growth and water status. When the effect of treatments is pronounced, model predictions are consistent with ground-reference data.
更多查看译文
关键词
hyperspectral data,physiological traits,drought treatments,rice
AI 理解论文
溯源树
样例
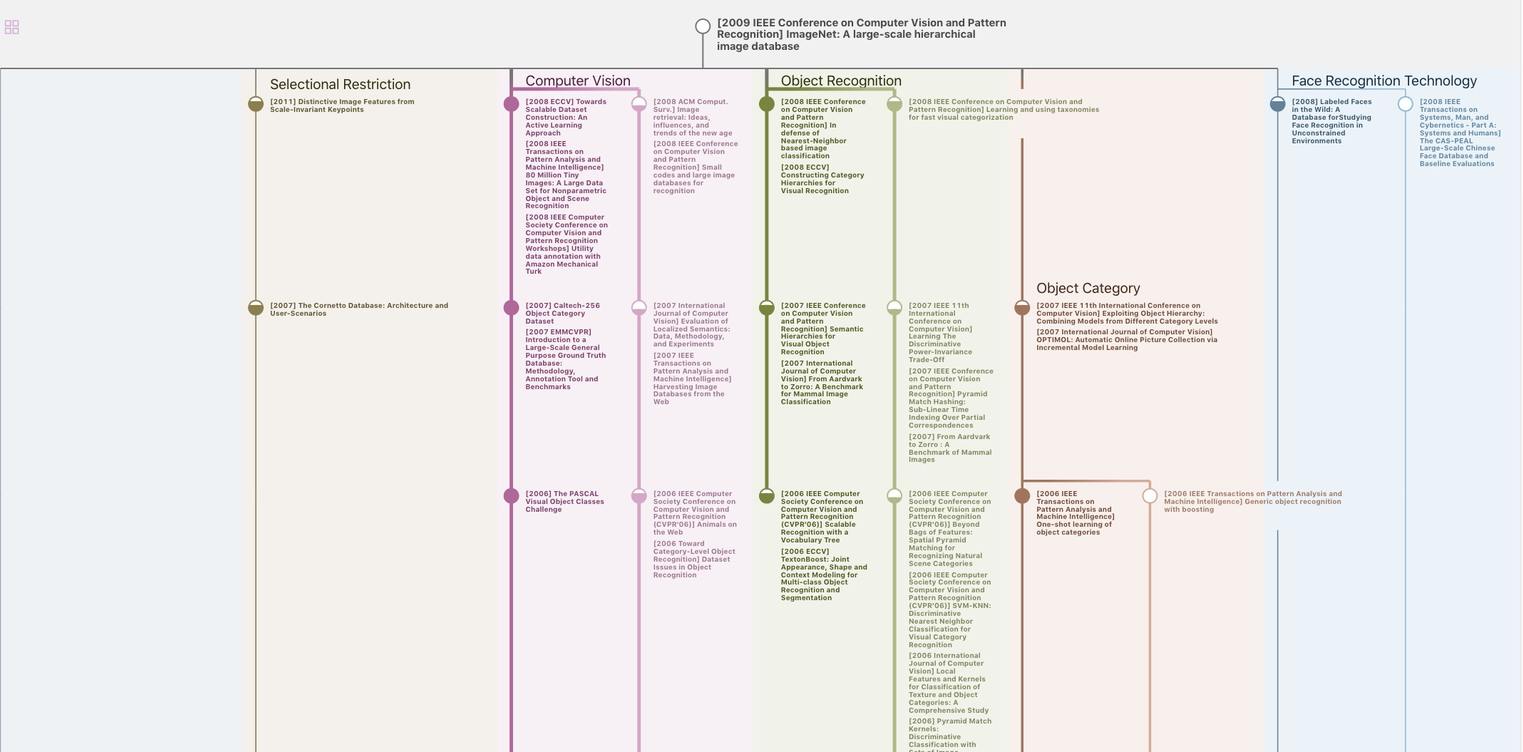
生成溯源树,研究论文发展脉络
Chat Paper
正在生成论文摘要