Multimodel ensemble analysis with neural network gaussian processes
ANNALS OF APPLIED STATISTICS(2023)
摘要
Multimodel ensemble analysis integrates information from multiple climate models into a unified projection. However, existing integration approaches, based on model averaging, can dilute fine-scale spatial information and incur bias from rescaling low-resolution climate models. We propose a statistical approach, called NN-GPR, using Gaussian process regression (GPR) with an infinitely wide deep neural network based covariance function. NN-GPR requires no assumptions about the relationships between climate models, no interpolation to a common grid, and automatically downscales as part of its prediction algorithm. Model experiments show that NN-GPR can be highly skillful at surface temperature and precipitation forecasting by preserving geospatial signals at multiple scales and capturing interannual variability. Our projections particularly show improved accuracy and uncertainty quantification skill in regions of high variability, which allows us to cheaply assess tail behavior at a 0.44 degrees/50 km spatial resolution without a regional climate model (RCM). Evaluations on reanalysis data and SSP2-4.5 forced climate models show that NN-GPR produces similar, overall climatologies to the model ensemble while better capturing fine-scale spatial patterns. Finally, we compare NN-GPR's regional predictions against two RCMs and show that NN-GPR can rival the performance of RCMs using only global model data as input.
更多查看译文
关键词
Multimodel ensembles,climate model integration,Gaussian process regression,deep learning
AI 理解论文
溯源树
样例
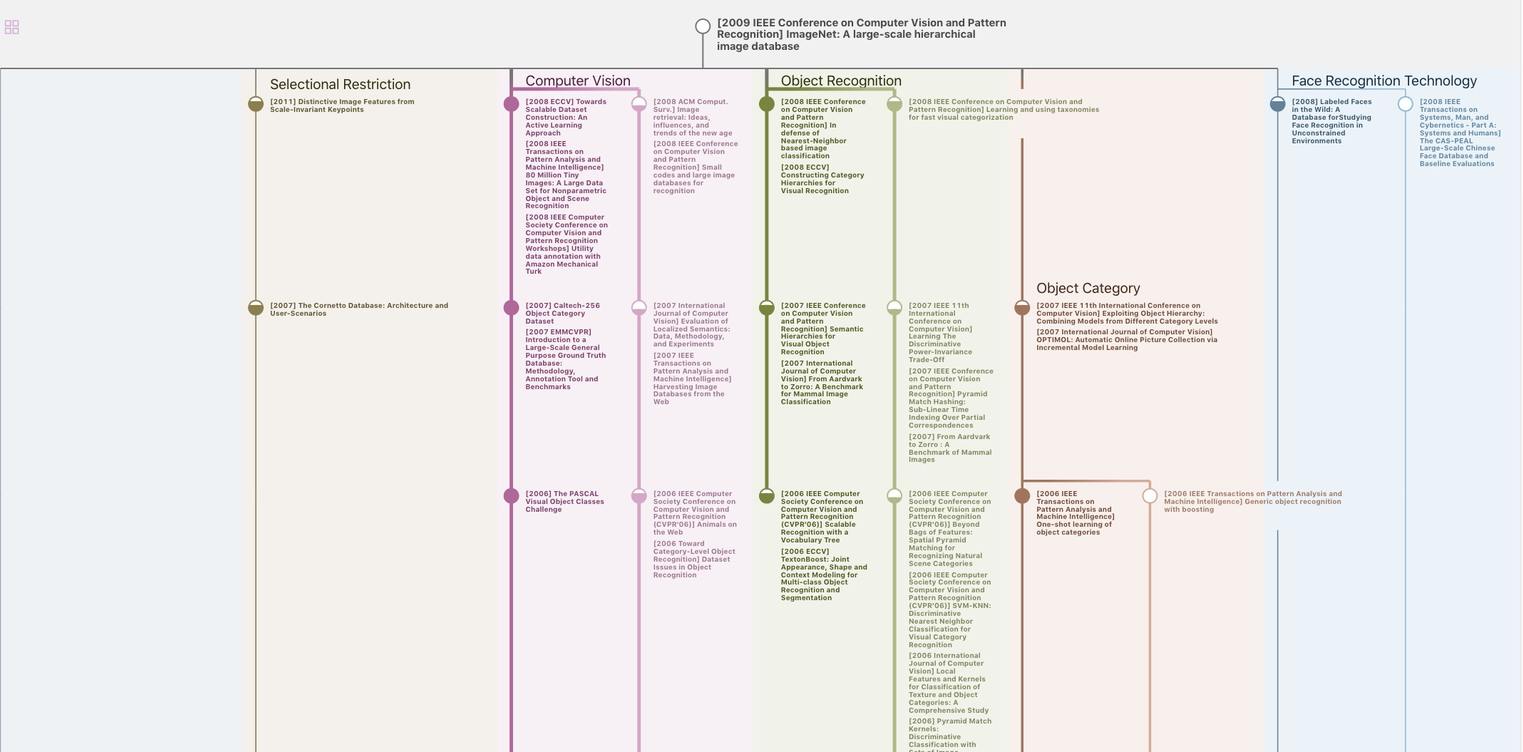
生成溯源树,研究论文发展脉络
Chat Paper
正在生成论文摘要