Sensing-enabled Predictive Beamforming Design for RIS-assisted V2I Systems: A Deep Learning Approach
IEEE Transactions on Wireless Communications(2023)
摘要
Vehicle-to-infrastructure (V2I) communications have been regarded as an emerging application in next-generation wireless networks. However, guaranteeing high-quality wireless communications in high-mobility scenarios remains a major challenge. In this paper, we investigate the deployment of reconfigurable intelligent surface (RIS) for improving the communication performance of V2I systems. In particular, integrated sensing and communication (ISAC) signals are exploited to facilitate sensing-assisted beamforming. Aiming at maximizing the achievable rate, two deep learning-based predictive beamforming mechanisms are proposed. First, a two-stage beamforming design is devised, where the channel state information (CSI) is estimated based on the echo signals and predicted by a dedicated neural network for time-varying channels. Then, the transmit beamforming vector at the base station (BS) and the reflect beamforming matrix at the RIS are jointly optimized. To further reduce the computational complexities, we develop an end-to-end beamforming design by employing the parameter sharing mechanism and weighted loss function. Simulation results demonstrate that the proposed algorithms can achieve an outstanding data rate that approaches the upper bound exploiting perfect CSI. In particular, the end-to-end design exhibits remarkable robustness against the impact of noise and achieves outstanding sensing-assisted beamforming performance, especially at the low signal-to-noise ratio region.
更多查看译文
关键词
Vehicular networks,reconfigurable intelligent surface,integrated sensing and communication,beamforming design,deep learning
AI 理解论文
溯源树
样例
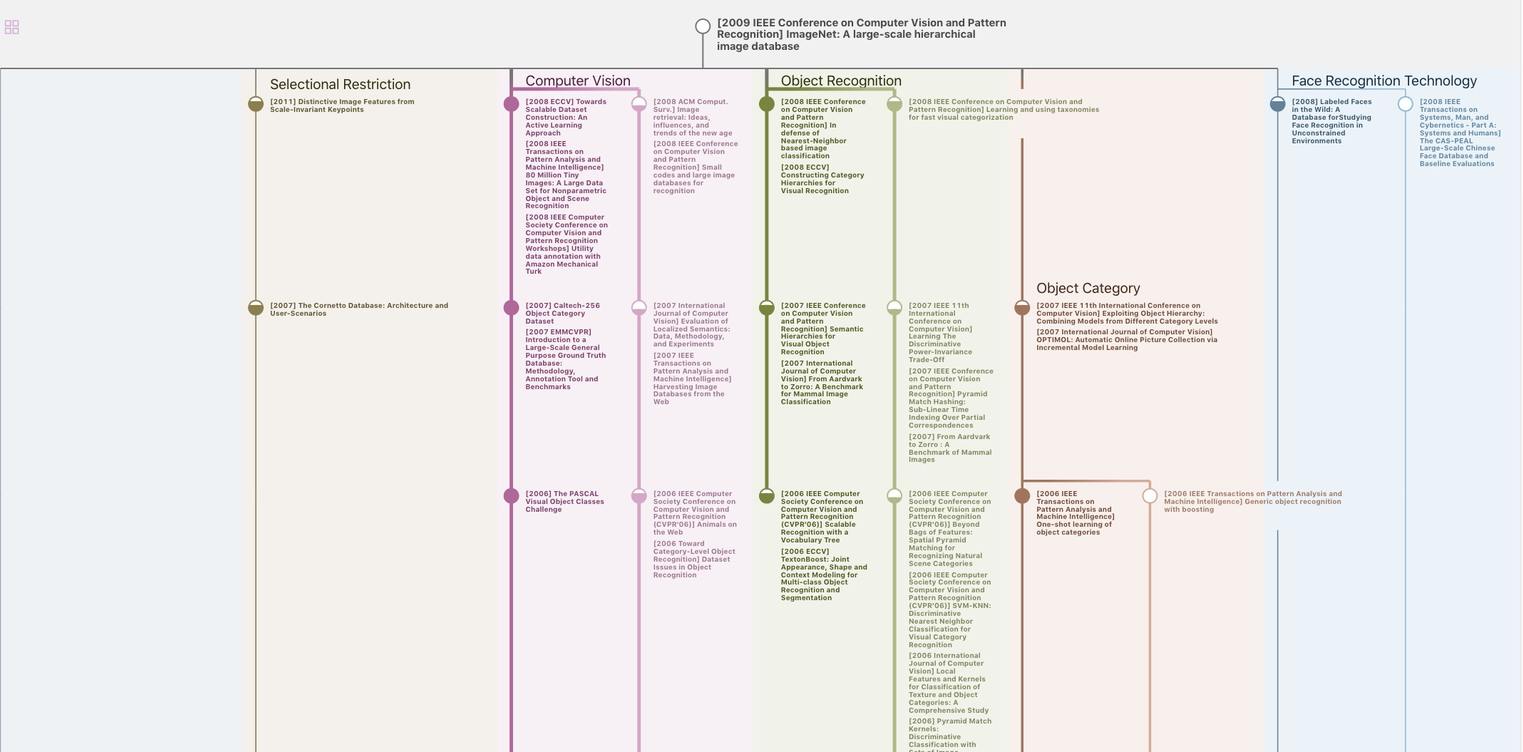
生成溯源树,研究论文发展脉络
Chat Paper
正在生成论文摘要