Convolutional Neural Networks in Auto-Segmentation of Nasopharyngeal Carcinoma Tumor — a Systematic Review and Meta-Analysis
Oncology in Clinical Practice(2023)
摘要
Introduction. Segmentation is one of the main stages of the treatment planning system (TPS), especially in nasopharyngeal carcinoma (NPC), because it is very heterogeneous and penetrates the skull bone tissue. An automated method to reduce the workload and human error caused by the lack of expertise and perspective would be very helpful. This meta-analysis evaluated the ability of convolutional neural networks (CNNs) to plan auto-segmentation computed tomography (CT) and magnetic resonance imaging (MRI) modalities. Material and methods. Articles published in PubMed, Scholar, and Cochrane databases were examined. The risk of bias was evaluated by the QUADAS-2 tool. The dice similarity coefficient (DSC) as the effect size and standard error (SE) as the precision index were analyzed by random effects. To calculate the degree of heterogeneity and its agent, we used ( I 2 and τ2) and meta-regression analysis (p < 0.05). A funnel plot was used to check for publication bias. Results. In general, eight studies on CT and 12 on MRI modalities were selected from 3601 studies. The heterogeneity based on ( I 2 and τ 2) and DSC values (with a 95% confidence interval) for CT and MRI modalities were 88.7% ( τ 2 = 0.011), 0.67 (0.62–0.72), and 81.42% ( τ 2 =0.01), 0.76 (0.72–0.80), respectively. Conclusions. CNNs’ ability to segment both CT and MRI modalities is at a medium level, and its improvement can make it more suitable for clinical use.
更多查看译文
关键词
Image Segmentation,Transfer Learning,Convolutional Neural Networks,Deep Learning,Feature Extraction
AI 理解论文
溯源树
样例
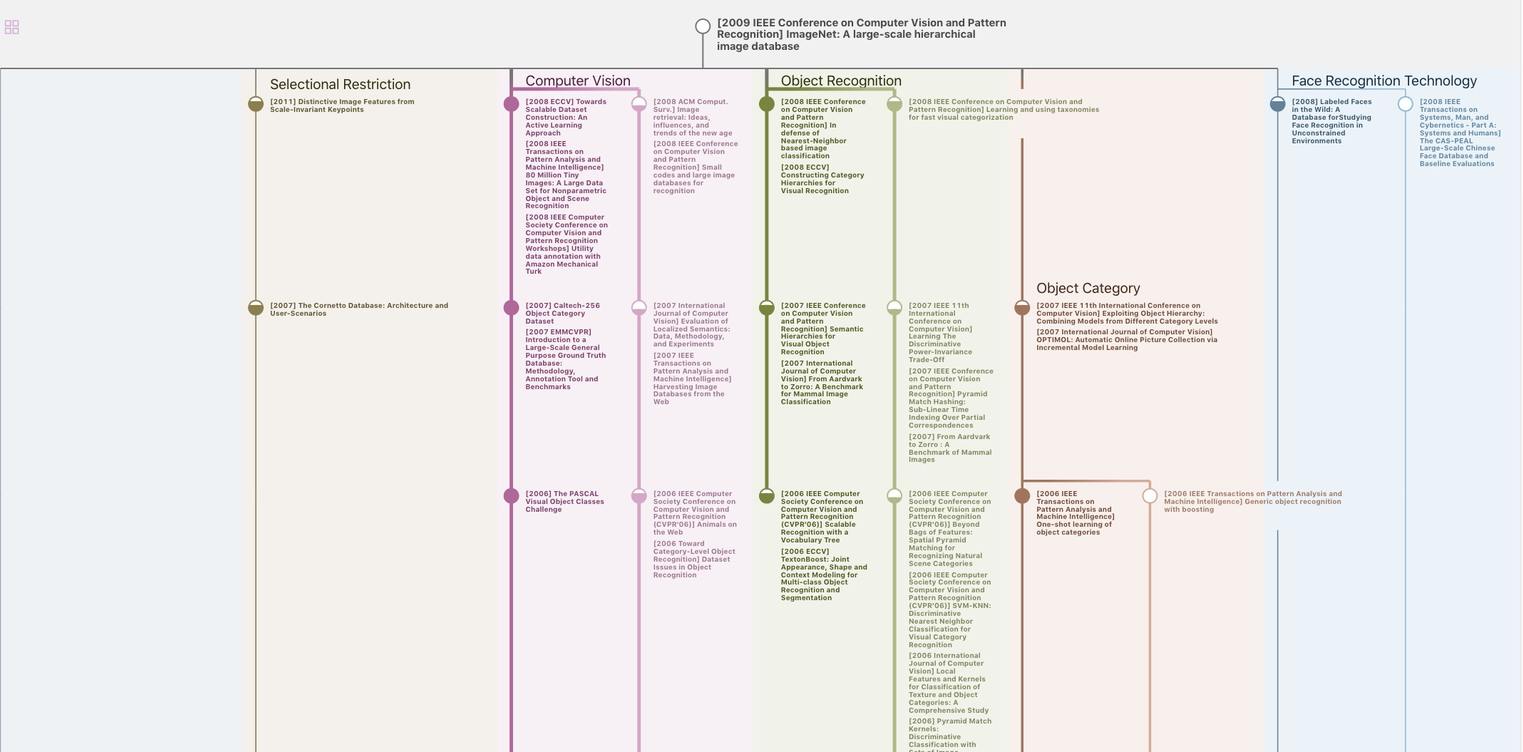
生成溯源树,研究论文发展脉络
Chat Paper
正在生成论文摘要