Nimg-50. total tumor cellularity and cellular growth rates estimated using a radio-pathomic ai model predicts prognosis and response to cytotoxic therapy in recurrent gbm
Neuro-oncology(2023)
摘要
Abstract PURPOSE A radio-pathomic machine learning model trained with postmortem tissue samples has recently been developed to estimate tumor cellularity from standard anatomic MR images (Bobholz SA et al. AJNR 2022). The aim of this study was to use this externally developed, machine learning-based model to assess the impact of tumor cellularity and changes in cellularity on survival in recurrent glioblastoma (rGBM) treated with standard cytotoxic therapies. METHODS We retrospectively evaluated 22 cases of rGBM treated with chemotherapy. Radio-pathomic maps of predicted tumor cellularity were generated using the radio-pathomic model using pre- and post-contrast T1-weighted MR images, T2-weighted FLAIR images and apparent diffusion coefficient calculated from diffusion-weighted imaging. Total cellularity was estimated by integrating the cellularity measures within a contrast-enhancing tumor volume of interest. Cellularity growth rates (CGRs) were computed using three pre-treatment scans and available post-treatment scans. Tumor cellularity and CGRs were assessed in terms of their impact on overall survival (OS) using log-rank analysis. RESULTS Patients with baseline tumor cellularity < 8.89x108 cells and those with pre-treatment CGR < 7.55x106 cells/day showed significantly longer OS than those with higher cellularity or CGRs (P = 0.001 and 0.008, respectively). Patients with cellularity < 8.90x108 cells at the 1st follow-up (one month after treatment), those with cellularity at 2nd follow-up < 8.89x108 cells (two months after treatment), post-treatment CGR < 1.51x107 cells/day, and change in CGR after treatment < +5.91x106 cells/day showed significantly longer OS than those with higher values (P = 0.03, < 0.001, < 0.001, = 0.02, respectively). CONCLUSION These preliminary results suggest the potential value of estimating total cellularity within the enhancing tumor as a biomarker for prognosis prediction and cytotoxic therapy effect evaluation in rGBM. Additional analyses with more cases and treatments are warranted.
更多查看译文
关键词
total tumor cellularity,cellularity growth rates,cytotoxic therapy,radio-pathomic
AI 理解论文
溯源树
样例
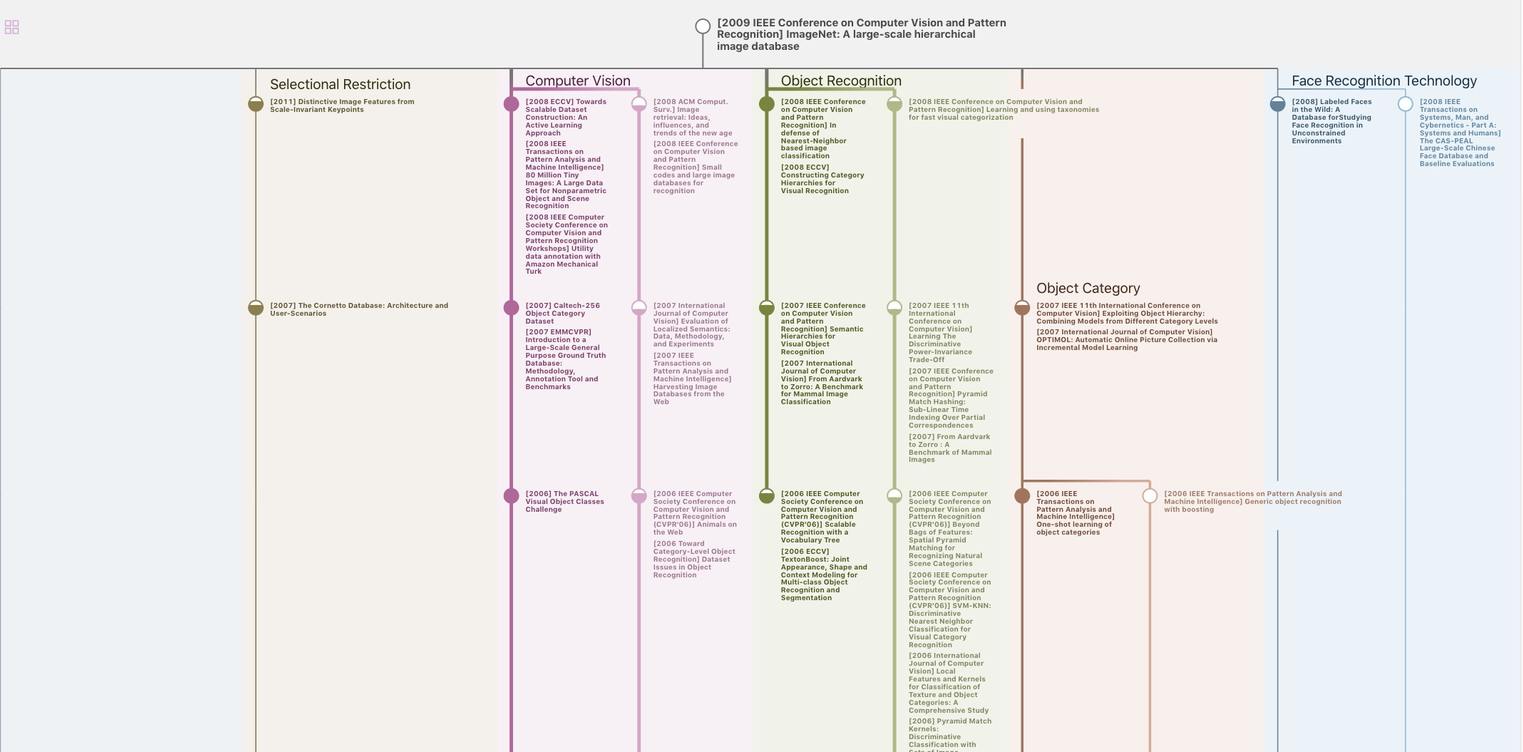
生成溯源树,研究论文发展脉络
Chat Paper
正在生成论文摘要