Innv-24. machine learning-based spectroscopic tissue differentiation in fluorescence-guided neurosurgery
Neuro-oncology(2023)
摘要
Abstract Maximal resection of malignant gliomas is hindered by difficulty in distinguishing tumor margins. Fluorescence-guided resection with 5-ALA assists in reaching this goal. Previously, we characterized five fluorophore emissions that accurately represent any spectrum measured from human brain tumor biopsies with a wide-field hyperspectral device. In this study, the effectiveness of these five spectra was explored for different tumor classification tasks in 891 hyperspectral widefield measurements of 184 patients harboring low- (n=30) and high-grade gliomas (n=115), non-glial primary brain tumors (n=19), radiation necrosis (n=2), miscellaneous (n=10) and metastases (n=8), which corresponds to up to 15000 spectra for a given test. The statistical differences in fluorophore abundances between classes were determined and visualized using dimensionality reduction techniques, including principal component analysis (PCA) and t-distributed stochastic neighbor embedding (t-SNE). Three machine-learning models were trained to classify tumor type (12 classes), grade (3 classes), and glioma margins (3 classes). Five algorithms were tested with varying hyperparameters for each classification task. We explored whether PCA projection onto five different axes than fluorophore abundances can provide more information for visualization and classification. The abundances of the five a priori fluorophore spectra matched or outperformed the five optimal PCA components for all classification tasks. These five axes capture 96-99% of the variance in the dataset. Using random forests and multilayer perceptrons, the classifiers achieved average test accuracies of 74-82%, 79%, and 81%, respectively. All five fluorophore abundances varied between tumor margin type as well as between grades (p < 0.01). For tissue type, at least four of five fluorophore abundances were found to be significantly different (p < 0.01) between all classes. These results demonstrate the differing contribution of fluorophores in different tissue classes tissue, as well as the five fluorophores value as potential optical biomarkers, opening new opportunities for intraoperative classification systems in fluorescence-guided neurosurgery.
更多查看译文
关键词
differentiation,spectroscopic,learning-based,fluorescence-guided
AI 理解论文
溯源树
样例
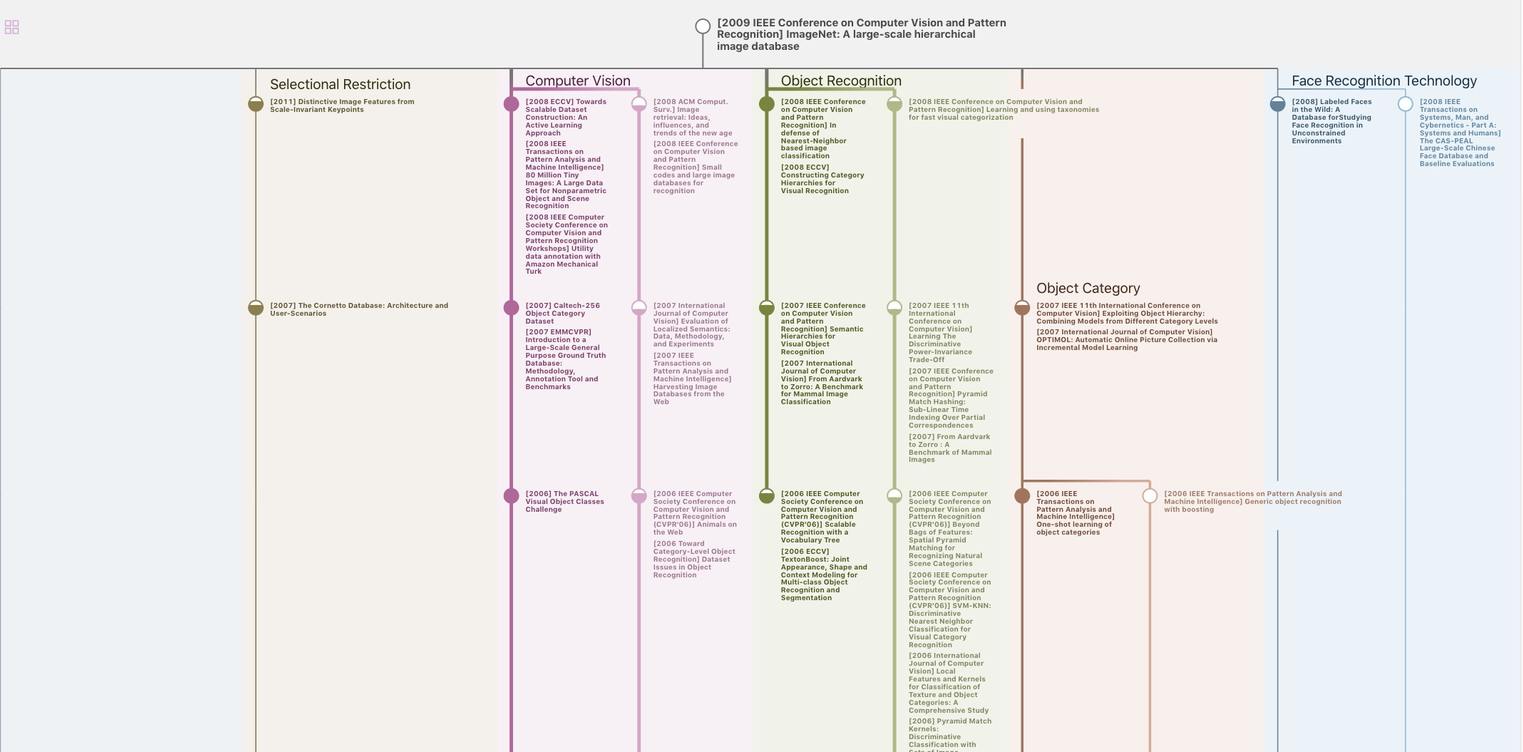
生成溯源树,研究论文发展脉络
Chat Paper
正在生成论文摘要