Development of an AI-based IT tool to support medical device nomenclature standardization for post-market surveillance by automated mapping from GMDN to EMDN standards
European Heart Journal(2023)
摘要
Abstract Introduction The EU Medical Device Regulation 2017/745 has defined new rules for medical device (MD) certification and post-market surveillance (PMS). In particular, a centralized database (Eudamed) has been established to collect and share information about the lifecycle of MD in EU market. To become effective for PMS, the European MD Nomenclature (EMDN), a 7-levels hierarchical system, has been adopted as a free standard among EU to describe MDs to this aim. However, Global MD Nomenclature (GMDN), a poly-hierarchical not-for-free system, was previously used in several EU Member States, and it is still adopted in other countries (i.e., UK, Australia, Brazil). A complete mapping between these nomenclatures is still missing, or not publicly and freely available, and this multiplicity of nomenclatures is going to affect the traceability of MD in PMS, ultimately generating an adverse impact on the patient. Purpose 1) To develop an IT tool to automatically map a given GMDN description to the most appropriate EMDN code, to facilitate and accelerate the uptake of EMDN and the mapping process between nomenclatures. 2) To introduce a user-science approach towards a continuous improvement of the tool, allowing user interaction to highlight potentially incorrect mapping and suggest the most appropriate one, to be further included in the database after manual verification. Methods By text analytics methods, the freely available list of MD on the Italian market, described by EMDN code, was compared with the same MD on the USA market, described by GMDN. As a result, 379317 devices were found present in both the Italian and USA markets, and 9683 different mappings between GMDN and EMDN are used to define an initial mapping from GMDN to EMDN. Then, given in input a GMDN description, semantic similarity was searched in the vocabulary for the proper GMDN with the corresponding EMDN, as well as for all EMDN descriptions, using a pre-trained AI transformer model (all-mpnet-base-v2). As a result, two possible EMDN codes with the respective reliability were given as output. The mapping performance at different EMDN levels was tested using a leave-one-out approach only on 2863 unique GMDN descriptions. Results From the GMDN descriptions, the correct EMDN code at level 1 (22 categories available) was given in 2343 cases (81.84%), at level 2 in 2088 cases (72.93%) and at level 3 in 1605 cases (56.06%). In Figure 1, an example of the developed user interface with the resulting two possible EMDN codes and their reliability, given a GMDN description of interest, is shown. The ability to select the most appropriate mapping, or to suggest a different one is given to the user, thus indirectly giving information for improving the tool. Conclusions The proposed approach provides with good accuracy the automatic mapping between GMDN and EMDN, thus potentially accelerating the nomenclature standardization process and improving effectiveness of MD PMS in EU and beyond.Figure 1
更多查看译文
关键词
medical device nomenclature standardization,gmdn,ai-based,post-market
AI 理解论文
溯源树
样例
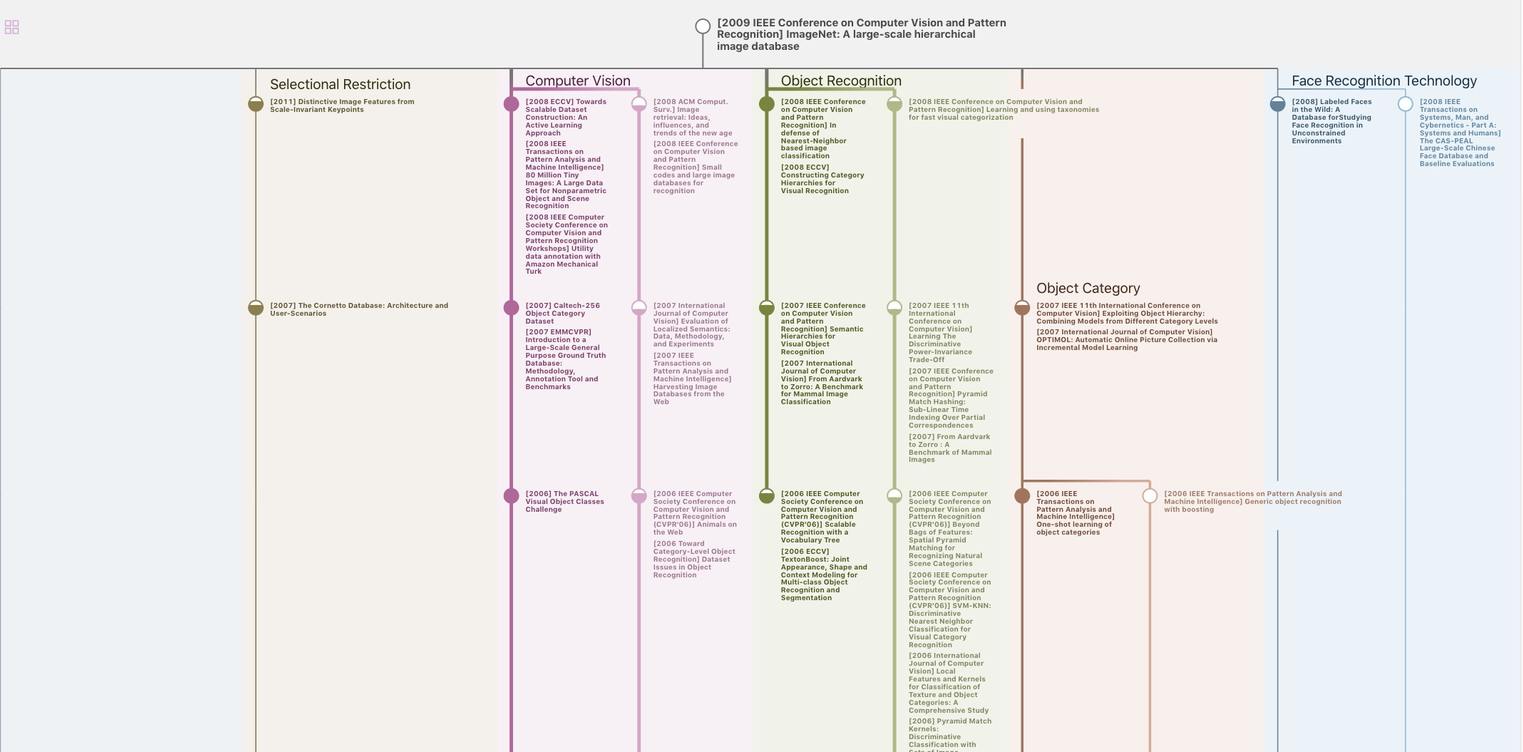
生成溯源树,研究论文发展脉络
Chat Paper
正在生成论文摘要