Combining hypothesis-driven ECG indices with machine learning improves ventricular arrhythmic risk prediction in a low-risk population
European Heart Journal(2023)
摘要
Abstract Background Life-threatening ventricular arrhythmias (LTVA) are a leading cause of mortality, but early identification of high-risk individuals remains a major challenge. An ECG index, TMV (T-wave morphology variations), predicts LTVA in 51,794 individuals without known cardiovascular disease from the UK Biobank (UKB) within a 10-year follow up (cohort 1), with an area under the ROC curve (AUC) of 0.558 and a hazard ratio (HR) of 1.57. Machine learning on the ECG has shown a high accuracy in detecting patients with cardiovascular disease, but its LTVA predictive value in a low-risk population and comparisons with hypothesis-driven risk markers, like TMV, is unknown. Purpose We tested the LTVA predictive value of a score combining a multi-layer convolutional neural network (CNN) model and TMV in individuals without known cardiovascular disease. Methods Using UKB we split the individuals from cohort 1 into training (90%) and internal test (10%) sets (Figure 1). In the training set, we applied 10-fold cross validation to train a multi-layer CNN with an attention layer, where the input was a 15-second ECG at rest (lead I), and the output was occurrence (or not) of LTVA within a 10-year follow-up. We used 10-second ECG data (lead I) from an independent cohort of 32,209 individuals without cardiovascular disease from UKB (cohort 2) as an external test set (median follow-up was 3.4 years), where we also calculated TMV. We next combined them into a score (weighted by their relative contribution to LTVA in a logistic regression). The AUC, and Cox regression HRs were calculated to estimate the performance of the CNN, TMV and the combined score. Results In cohort 1, 217 subjects had a LTVA during the follow-up period. In the internal test set, the AUC of the CNN was 0.707. In the external test set (60 LTVA events during the follow-up period), the CNN’s prediction and TMV led to AUCs of 0.610 and 0.604, respectively. Interestingly, the CNN’s prediction and TMV were not correlated (ρ = 0.005), and the combined score led to an AUC of 0.627. We set a threshold at the score value that maximised sensitivity and specificity, and survival analyses showed a HR of 3.007 (P < 0.0001) for individuals in the score+ (score > threshold) versus those in the score- (score < threshold) group, after adjusting for age and gender (Figure 2). The continuous score also predicted LTVA independently from age and gender. Conclusions A CNN-based model predicts LTVA in a low-risk population independently from TMV, a strong ECG risk predictor derived from knowledge on the underlying electrophysiology. When TMV and the CNN are combined into a score, the LTVA risk stratification improves, independently from age and gender. Our findings support the combination of hypothesis-based risk markers and CNN approaches to optimise LTVA prediction in a low-risk population.Study designSurvival curves
更多查看译文
关键词
ventricular arrhythmic risk prediction,ecg indices,risk prediction,machine learning,hypothesis-driven,low-risk
AI 理解论文
溯源树
样例
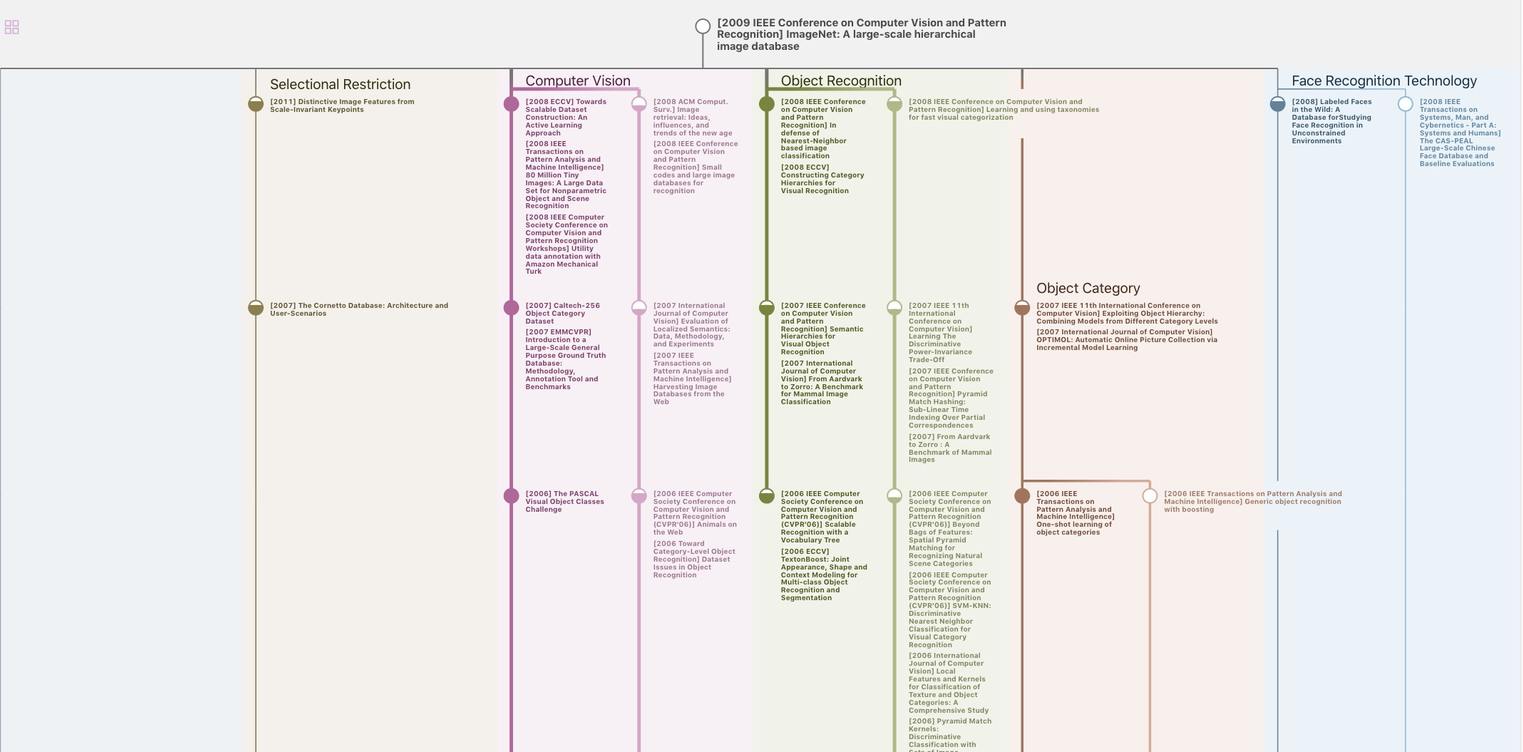
生成溯源树,研究论文发展脉络
Chat Paper
正在生成论文摘要