News-Based Sparse Machine Learning Models for Adaptive Asset Pricing
Data Science in Science(2023)
摘要
The paper proposes two sparse machine learning based asset pricing models to explain and predict the stock returns and industry returns based on the financial news. For stock returns, the proposed News Embedding UMAP Sparse Selection (NEUSS) model first derives the asset embeddings for each asset from the financial news related to it, obtains a collection of the basis assets based on their asset embeddings, and then for each stock, select the basis assets to explain and predict the stock return with high-dimensional statistical methods with sparsity. For industry returns, the proposed Industry-specific News Sparse Encoder with Rationale (INSER) model learns sparse Rationale words for each industry using a sparse generator-encoder framework and then predicts the industry returns with a Rationale-based random forest. The proposed modeling approaches are shown to have significantly better fitting and prediction power than the current benchmarks.
更多查看译文
关键词
adaptive asset pricing,asset pricing,sparse machine learning models,news-based
AI 理解论文
溯源树
样例
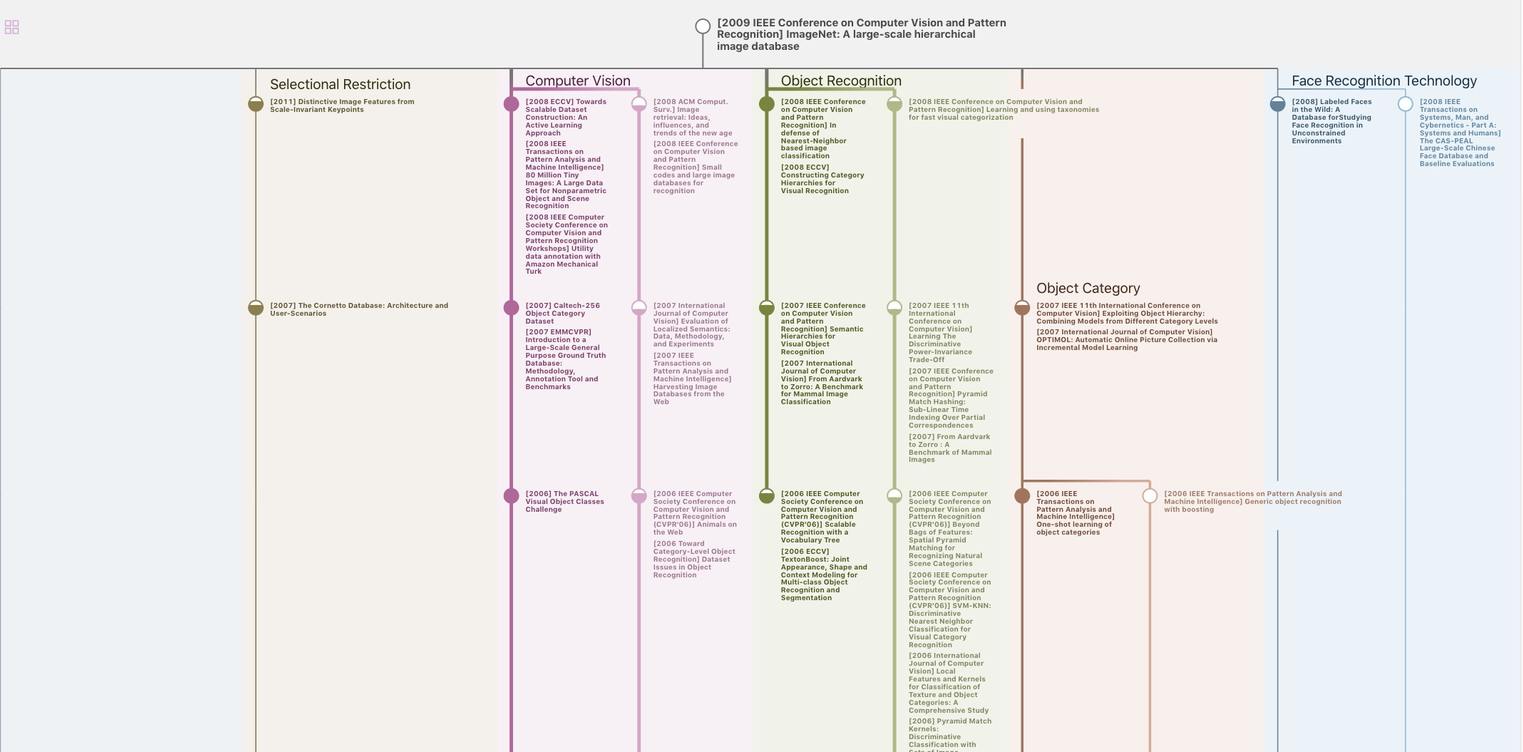
生成溯源树,研究论文发展脉络
Chat Paper
正在生成论文摘要