Reconstruction-guided attention improves the object recognition robustness of neural networks
Journal of Vision(2023)
摘要
Many visual phenomena suggest that humans use top-down generative or reconstructive processes to create visual percepts (e.g., imagery, object completion, pareidolia), but little is known about the role reconstruction plays in robust object recognition. We built an iterative encoder-decoder network that generates an object reconstruction and uses it as top-down attentional feedback to route the most relevant spatial and feature information to feed-forward object recognition processes. We tested this model using the challenging out-of-distribution object recognition dataset, MNIST-C (handwritten digits under corruptions) and IMAGENET-C (real-world objects under corruptions). Our model showed strong generalization performance against various image corruptions and significantly outperformed other feedforward convolutional neural network models (e.g., ResNet) on both datasets. Our model’s robustness was particularly pronounced under high levels of distortions, where it showed a maximum 20% accuracy improvement from the baseline model in the maximally noisy conditions in IMAGENET-C. Ablation studies further reveal two complementary roles of spatial and feature-based attention in robust object recognition, with the former largely consistent with spatial masking benefits in the attention literature (the reconstruction serves as a mask) and the latter mainly contributing to the model’s inference speed (i.e., number of time steps to reach a certain confidence threshold) by reducing the space of possible object hypotheses. Finally, the proposed model also yields high behavioral correspondence with humans, which are evaluated by the correlation between human and model’s response time (Spearman’s r=0.36, p
更多查看译文
关键词
object recognition robustness,attention,neural networks,reconstruction-guided
AI 理解论文
溯源树
样例
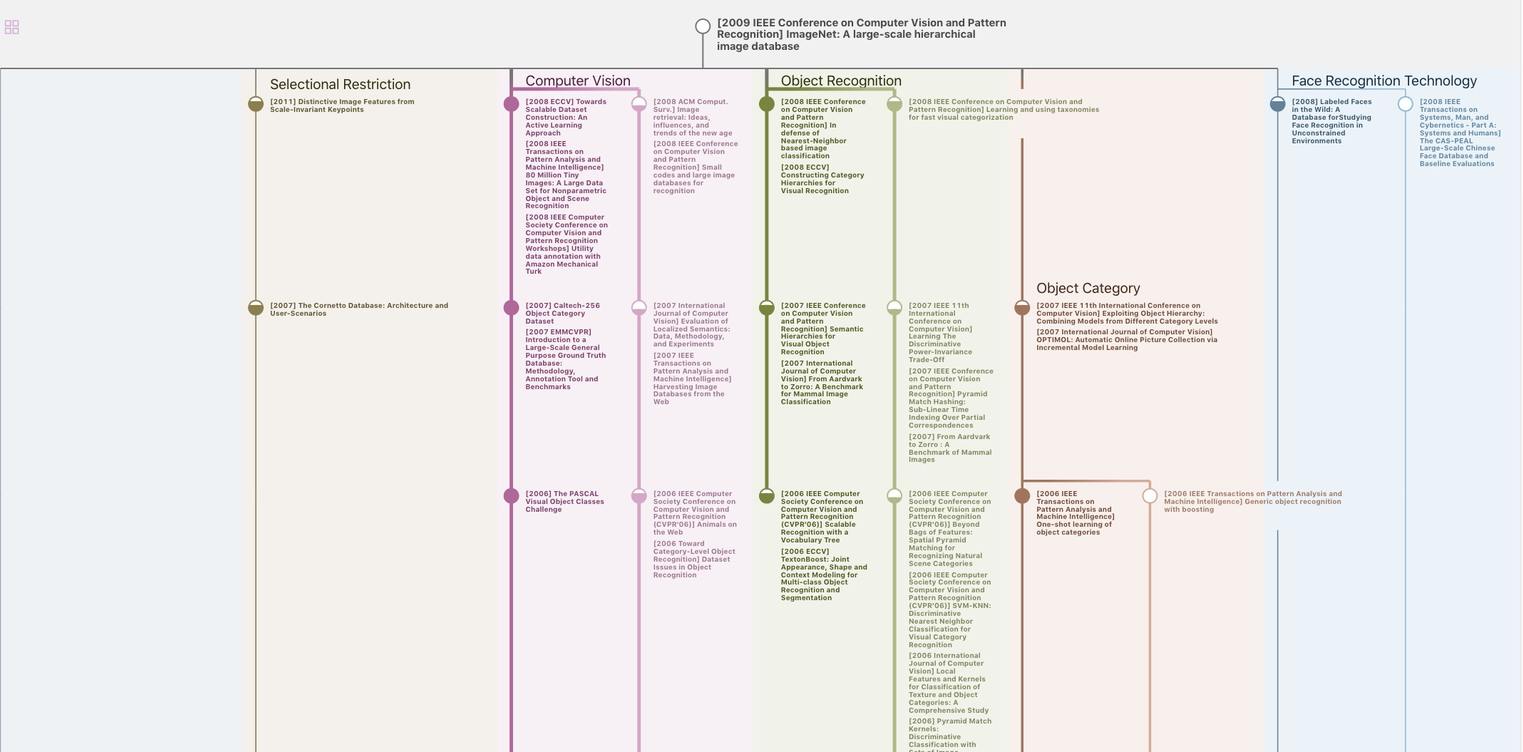
生成溯源树,研究论文发展脉络
Chat Paper
正在生成论文摘要