PrivyNAS: Privacy-Aware Neural Architecture Search for Split Computing in Edge-Cloud Systems.
IEEE Internet Things J.(2024)
摘要
Split Computing has become a prominent resource-efficient method to enable machine learning (ML) applications on user-constrained edge devices, where compute-intensive ML workloads can be delegated to remote cloud servers for processing. However, the exposure of data to the cloud service providers as such raises privacy alarms due to the possible leakage of sensitive user information. On a relevant note, typical deep neural network (DNN) design frameworks do not take into account model splitting and its complications at the early design stages of DNNs. Thus, a natural question arises on how to bridge this gap and optimize the DNN design process such that split computing operations can meet the requirements of accuracy, performance, and privacy. In this paper, we strive to address this question through adopting a privacy-by-design approach, where privacy is characterized either as a constraint or an objective to realize privacy-aware models tailored for split computing. Using the -differential privacy standard for our case study, we conduct intensive empirical analysis on the relation between architectural parameters and intrinsic privacy budgets, and propose PrivyNAS – a privacy-aware Neural Architecture Search framework for split computing. On the CIFAR-10 dataset, our approach has demonstrated promising results in providing DNN architectures that balance the required design trade-offs.
更多查看译文
关键词
Inference Privacy,Differential Privacy,Split Computing,Neural Architecture Search,Edge-Cloud
AI 理解论文
溯源树
样例
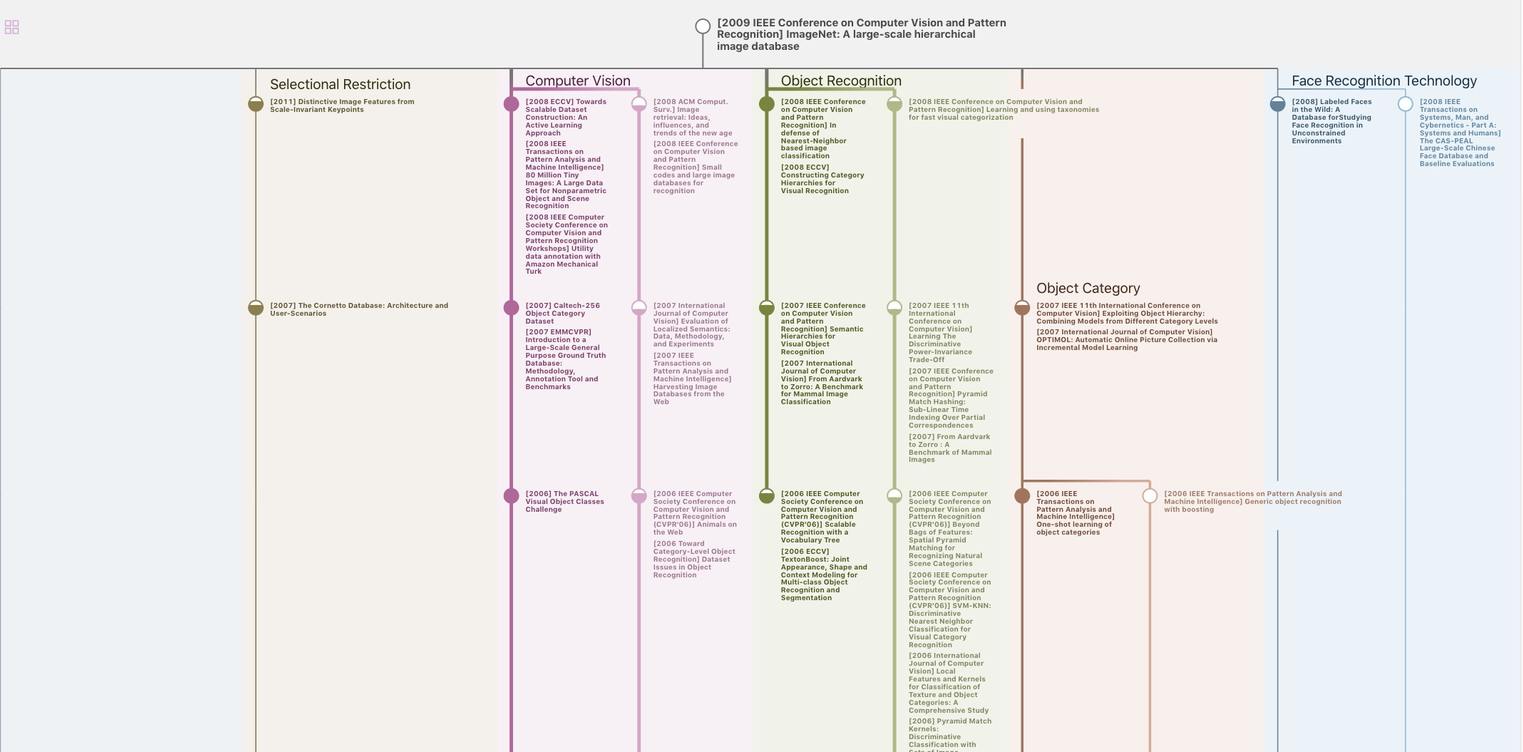
生成溯源树,研究论文发展脉络
Chat Paper
正在生成论文摘要