Stronger Privacy Amplification by Shuffling for Renyi and Approximate Differential Privacy
Society for Industrial and Applied Mathematics eBooks(2023)
摘要
The shuffle model of differential privacy has gained significant interest as an intermediate trust model between the standard local and central models [18, 12]. A key result in this model is that randomly shuffling locally randomized data amplifies differential privacy guarantees. Such amplification implies substantially stronger privacy guarantees for systems in which data is contributed anonymously [8].In this work, we improve the state of the art privacy amplification by shuffling results both theoretically and numerically. Our first contribution is the first asymptotically optimal analysis of the Renyi differential privacy parameters for the shuffled outputs of LDP randomizers. Our second contribution is a new analysis of privacy amplification by shuffling. This analysis improves on the techniques of Feldman et al. [20] and leads to tighter numerical bounds in all parameter settings.* The full version of the paper can be accessed at https://arxiv.org/abs/2208.04591
更多查看译文
关键词
stronger privacy amplification,renyi
AI 理解论文
溯源树
样例
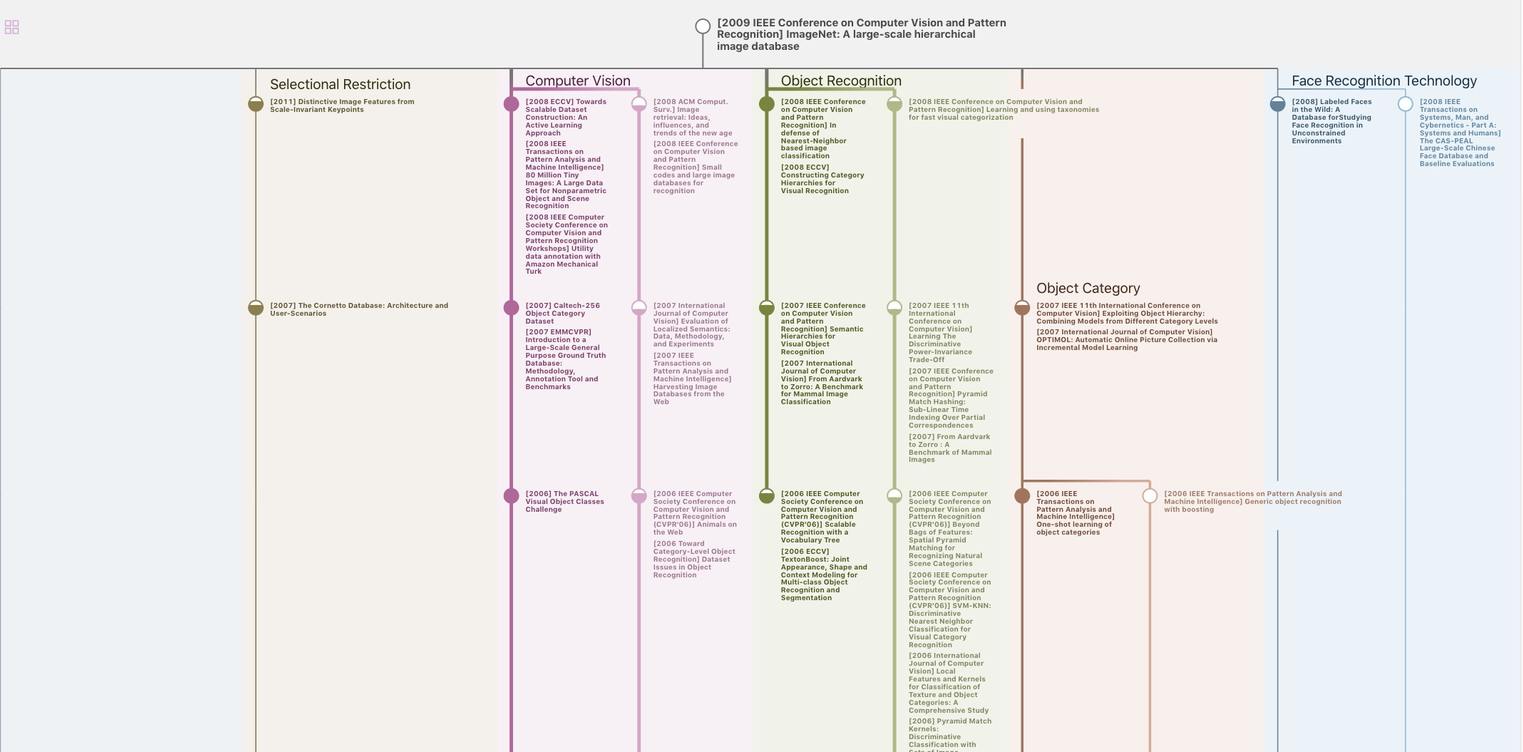
生成溯源树,研究论文发展脉络
Chat Paper
正在生成论文摘要