EV Charging Command Fast Allocation Approach Based on Deep Reinforcement Learning With Safety Modules
IEEE TRANSACTIONS ON SMART GRID(2024)
摘要
Efficient real-time management of electric vehicle (EV) charging in a charging station (CS) is vital to the integration of large-scale EVs in power grids. It faces critical challenges such as frequent changes in the grid's dispatch commands, the complexity of EVs' costs, and the uncertainties in the EVs' charging/traveling behaviors and in the future dispatch commands. To tackle these challenges, this paper proposes a deep reinforcement learning (DRL)-based allocation approach that optimally and efficiently allocates the grid's commands to the EVs and controls their charging in real time. It includes two stages. Stage 1 includes a data-driven EV cost quantification method, which efficiently quantifies the EVs' flexibility contributions with long-term return consideration. Stage 2 proposes a high sample efficiency DRL-based allocation method, which optimizes the EVs' charging and addresses the EV- and grid-related uncertainties. The proposed allocation has a fast computational speed. Finally, to address the security risk due to DRL's stochastic exploratory actions, two safety modules are developed which ensure the EV charging security and the allocation accuracy. The effectiveness and efficiency of the proposed strategy are verified by comparing its performance against multiple benchmark approaches.
更多查看译文
关键词
Deep reinforcement learning,electric vehicles,vehicle-to-grid,charging control,charging station
AI 理解论文
溯源树
样例
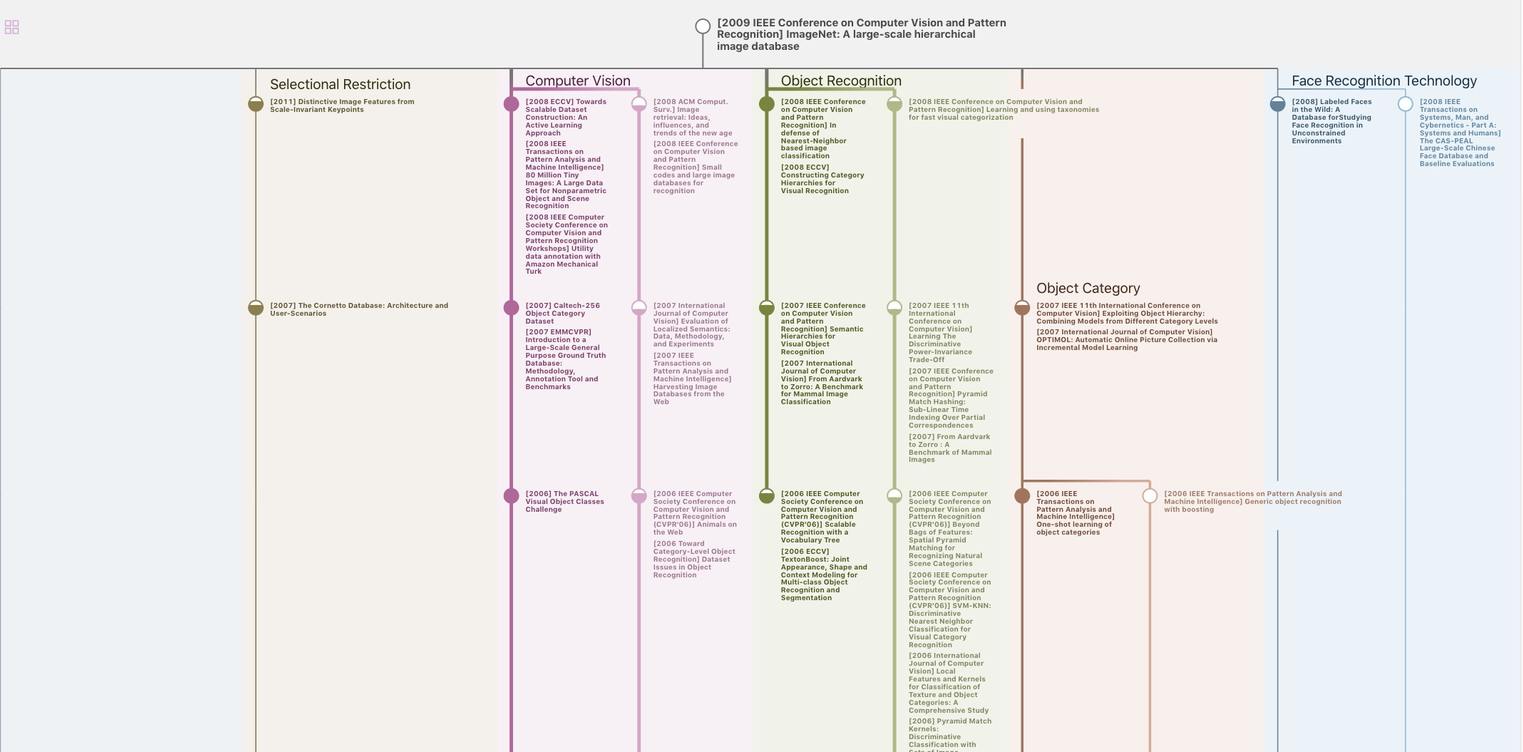
生成溯源树,研究论文发展脉络
Chat Paper
正在生成论文摘要