Counteracting data bias and class imbalance – towards useful and reliable retinal disease recognition system
Research Square (Research Square)(2023)
摘要
Abstract Fundus images play a fundamental role in the early screening of eye diseases. On the other hand, as deep learning provides an accurate classification of medical images, it is natural to apply such techniques for fundus images. There are many developments in deep learning for such image data but are often burdened with the same common mistakes. Training data are biased, not diverse and hidden to the public. Algorithms classify diseases, which suitability for screening could be questioned. Therefore, in our research, we consolidate most of the available public data of fundus images (pathological and non-pathological) taking into consideration only image data relevant to the most distressing retinal diseases. Next, we apply some well-known state-of-the-art deep learning models for the classification of the consolidated image data addressing class imbalance problem occurring in the dataset and clinical usage. In a conclusion, we present our classification results for diabetic retinopathy, glaucoma, and age- related macular degeneration disease, which are urgent problem of ageing populations in developed countries.
更多查看译文
关键词
class imbalance,data bias,recognition
AI 理解论文
溯源树
样例
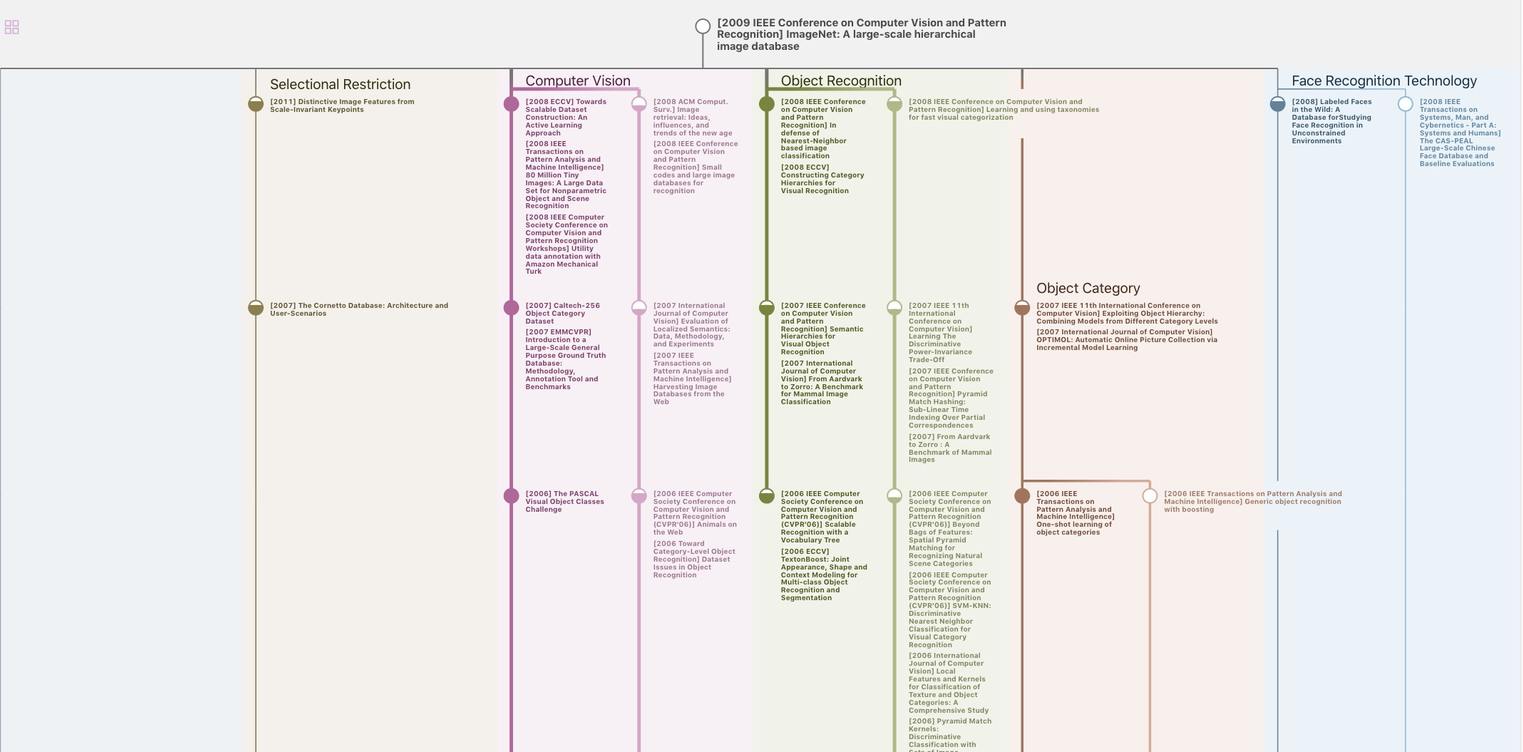
生成溯源树,研究论文发展脉络
Chat Paper
正在生成论文摘要