Network Utility Maximization with Unknown Utility Functions: A Distributed, Data-Driven Bilevel Optimization Approach
arXiv (Cornell University)(2023)
摘要
Fair resource allocation is one of the most important topics in communication networks. Existing solutions almost exclusively assume each user utility function is known and concave. This paper seeks to answer the following question: how to allocate resources when utility functions are unknown, even to the users? This answer has become increasingly important in the next-generation AI-aware communication networks where the user utilities are complex and their closed-forms are hard to obtain. In this paper, we provide a new solution using a distributed and data-driven bilevel optimization approach, where the lower level is a distributed network utility maximization (NUM) algorithm with concave surrogate utility functions, and the upper level is a data-driven learning algorithm to find the best surrogate utility functions that maximize the sum of true network utility. The proposed algorithm learns from data samples (utility values or gradient values) to autotune the surrogate utility functions to maximize the true network utility, so works for unknown utility functions. For the general network, we establish the nonasymptotic convergence rate of the proposed algorithm with nonconcave utility functions. The simulations validate our theoretical results and demonstrate the great effectiveness of the proposed method in a real-world network.
更多查看译文
关键词
unknown utility functions,optimization approach,data-driven
AI 理解论文
溯源树
样例
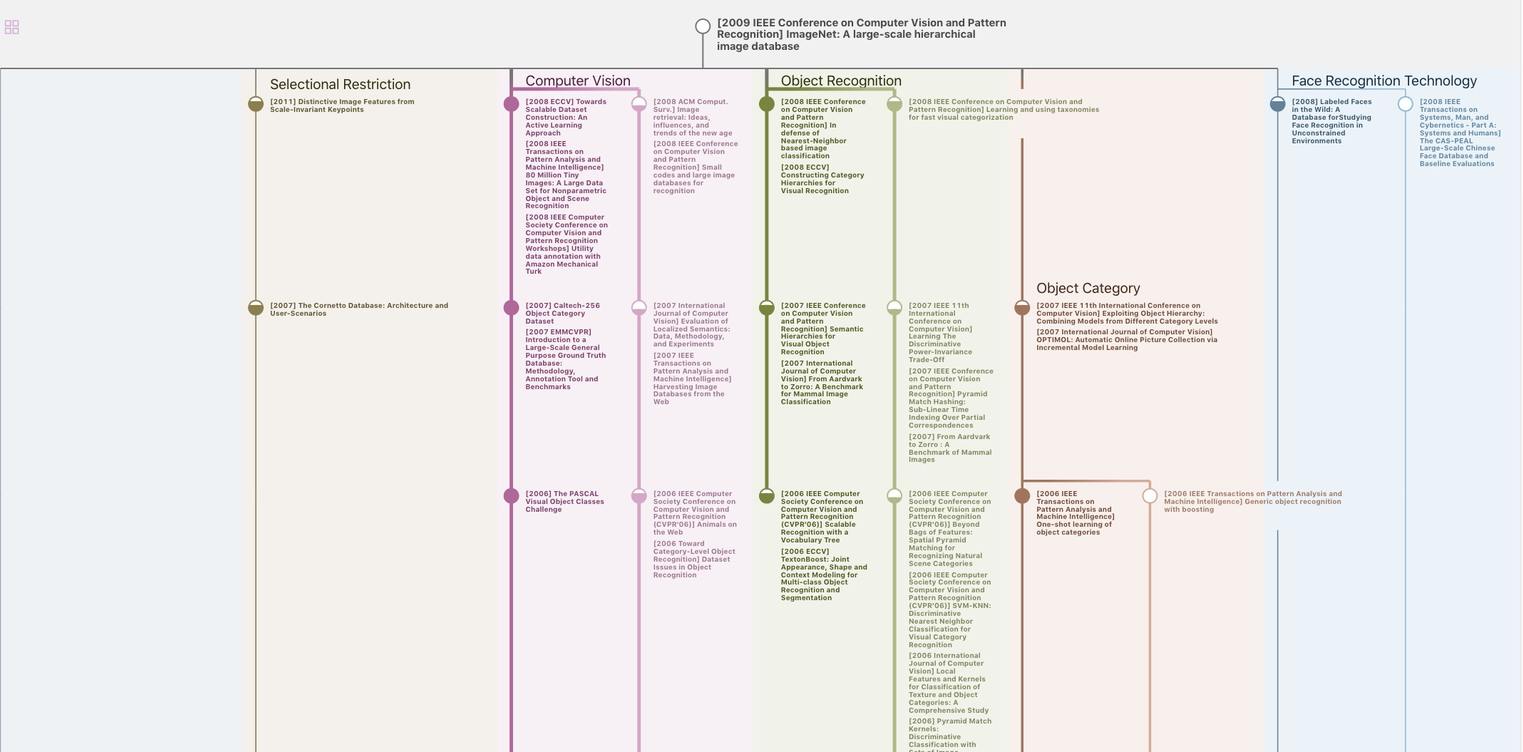
生成溯源树,研究论文发展脉络
Chat Paper
正在生成论文摘要